Weakly Supervised Referring Image Segmentation with Intra-Chunk and Inter-Chunk Consistency.
ICCV(2023)
摘要
Referring image segmentation aims to localize the object in an image referred by a natural language expression. Most previous studies learn referring image segmentation with a large-scale dataset containing segmentation labels, but they are costly. We present a weakly supervised learning method for referring image segmentation that only uses readily available image-text pairs. We first train a visual-linguistic model for image-text matching and extract a visual saliency map through Grad-CAM to identify the image regions corresponding to each word. However, we found two major problems with Grad-CAM. First, it lacks consideration of critical semantic relationships between words. We tackle this problem by modeling the relationship between words through intra-chunk and inter-chunk consistency. Second, Grad-CAM identifies only small regions of the referred object, leading to low recall. Therefore, we refine the localization maps with self-attention in Transformer and unsupervised object shape prior. On three popular benchmarks (RefCOCO, RefCOCO+, G-Ref), our method significantly outperforms recent comparable techniques. We also show that our method is applicable to various levels of supervision and obtains better performance than recent methods.
更多查看译文
AI 理解论文
溯源树
样例
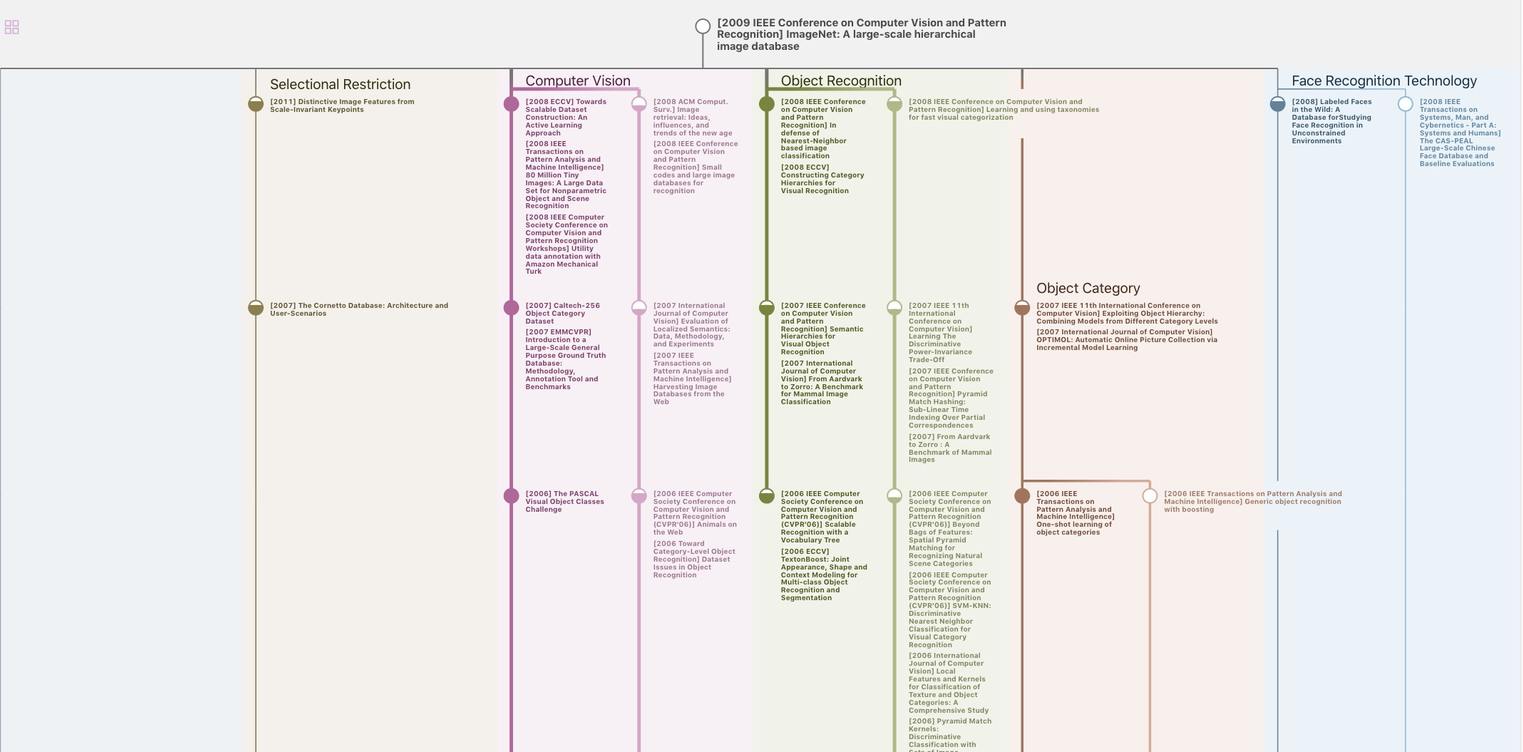
生成溯源树,研究论文发展脉络
Chat Paper
正在生成论文摘要