PLFM: Pixel-Level Merging of Intermediate Feature Maps by Disentangling and Fusing Spatial and Temporal Data for Cloud Removal
IEEE TRANSACTIONS ON GEOSCIENCE AND REMOTE SENSING(2022)
摘要
Cloud removal is a relevant topic in remote sensing, fostering medium- and high-resolution optical (OPT) image usability for Earth monitoring and study. Recent applications of deep generative models and sequence-to-sequence-based models have proved their capability to advance the field significantly. Nevertheless, there are still some gaps: the amount of cloud coverage, the landscape temporal changes, and the density and thickness of clouds need further investigation. We fill some of these gaps in this work by introducing an innovative deep model. The proposed model is multimodal, relying on both spatial and temporal sources of information to restore the whole optical scene of interest. We use the outcomes of both temporal-sequence blending and direct translation from synthetic aperture radar (SAR) to optical images to obtain a pixel-wise restoration of the whole scene. The reconstructed images preserve scene details without resorting to a considerable portion of a clean image. Our approach's advantage is demonstrated across various atmospheric conditions tested on different datasets. Quantitative and qualitative results prove that the proposed method obtains cloud-free images coping with landscape changes.
更多查看译文
关键词
Clouds,Optical imaging,Optical sensors,Image reconstruction,Radar polarimetry,Adaptation models,Image restoration,Cloud removal (CR),conditional generative adversarial networks (cGANs),convolutional long short-term memory (ConvLSTM),deep hierarchical model,multitemporal remote sensing (RS) images,synthetic aperture radar (SAR)-optical (OPT) data fusion
AI 理解论文
溯源树
样例
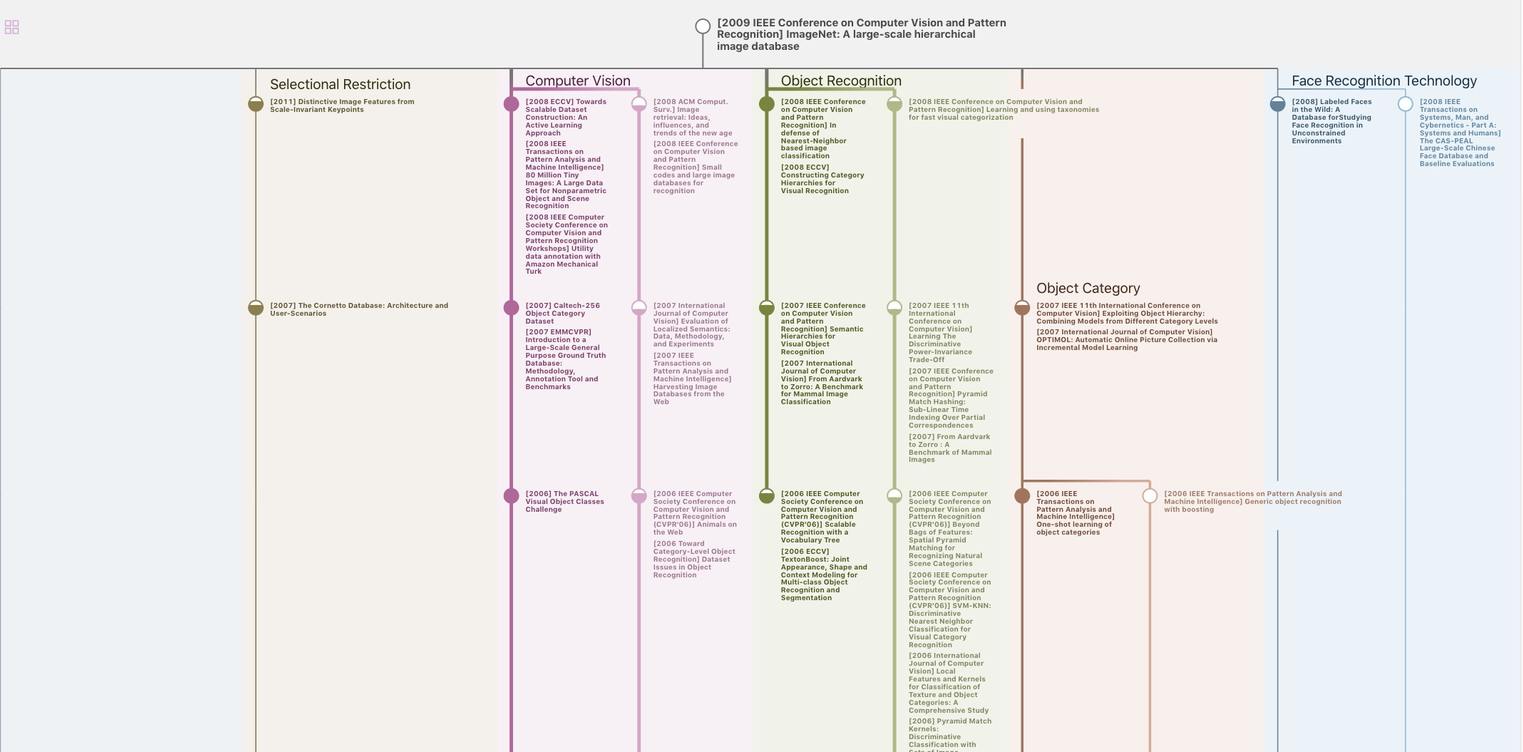
生成溯源树,研究论文发展脉络
Chat Paper
正在生成论文摘要