Learning Explicit and Implicit Dual Common Subspaces for Audio-Visual Cross-Modal Retrieval
ACM Transactions on Multimedia Computing, Communications, and Applications(2022)
摘要
Audio-visual tracks in video contain rich semantic information with potential in many applications and research. Since the audio-visual data have inconsistent distributions and the heterogeneous nature of representations, the heterogeneous gap between modalities makes them impossible to compare directly. To bridge the modality gap, a frequently adopted approach is to simultaneously project audio-visual data into a common subspace to capture the commonalities and characteristics of modalities for measurement, which has been extensively studied in relation to the issues of modality-common and modality-specific features learning in previous research. However, it is difficult for existing methods to address the trade-off between both issues, e.g., the modality-common feature is learned from the latent commonalities of audio-visual data or the correlated features as aligned projections, in which the modality-specific feature can be lost. To solve the trade-off, we propose a novel end-to-end architecture, which synchronously projects audio-visual data into the explicit and the implicit dual common subspaces. The explicit subspace is used to learn modality-common feature and reduce the modality gap of explicit paired audio-visual data, where the representation-specific details are abandoned to retain the common underlying structure of audio-visual data. The implicit subspace is used to learn modality-specific feature, where each modality privately pulls apart the features distances between different categories to maintain the category-based distinctions, by minimizing the distance between audio-visual features and corresponding labels. The comprehensive experimental results on two audio-visual datasets, VEGAS and AVE, demonstrate that our proposed model for using two different common subspaces for audio-visual cross-modal learning is effective and significantly outperforms the state-of-the-art cross-modal models that learn features from a single common subspace, by 4.30% and 2.30% in terms of average MAP on the VEGAS and AVE datasets, respectively.
更多查看译文
关键词
Modality-common,modality-specific,explicit and implicit,audio-visual cross-modal retrieval
AI 理解论文
溯源树
样例
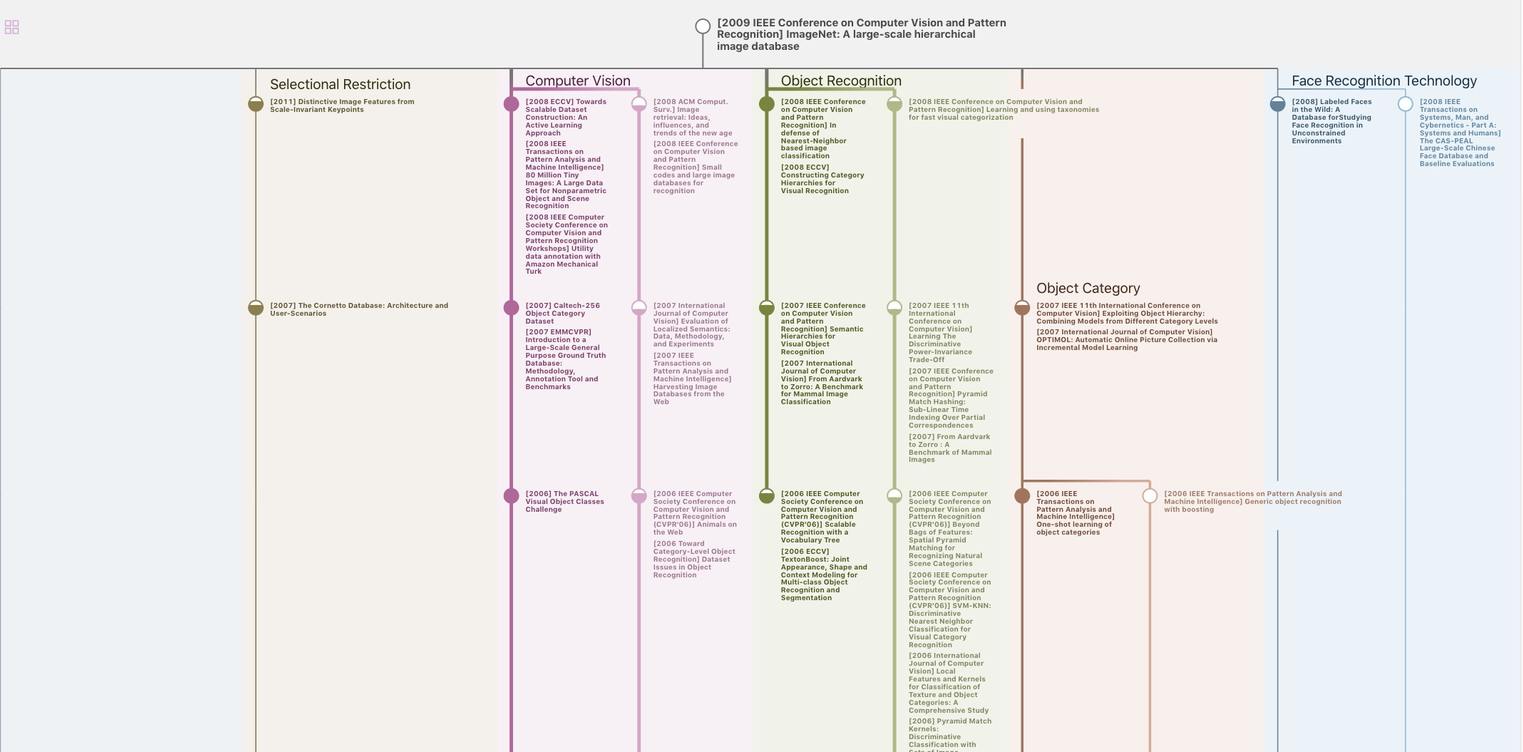
生成溯源树,研究论文发展脉络
Chat Paper
正在生成论文摘要