Selecting simple, transferable models with the supremum principle
Physical Review Research(2022)
摘要
We consider how mathematical models enable predictions for conditions that are qualitatively different from the training data. We propose techniques based on information topology to find models that can apply their learning in regimes for which there is no data. The first step is to use the manifold boundary approximation method to construct simple, reduced models of target phenomena in a data-driven way. We consider the set of all such reduced models and use the topological relationships among them to reason about model selection for new, unobserved phenomena. Given minimal models for several target behaviors, we introduce the supremum principle as a criterion for selecting a new, transferable model. The supremal model, i.e., the least upper bound, is the simplest model that reduces to each of the target behaviors. We illustrate how to discover supremal models with several examples; in each case, the supremal model unifies causal mechanisms to transfer successfully to new target domains. We use these examples to motivate a general algorithm that has formal connections to theories of analogical reasoning in cognitive psychology.Received 23 September 2021Revised 11 February 2022Accepted 1 June 2022DOI:https://doi.org/10.1103/PhysRevResearch.4.L032044Published by the American Physical Society under the terms of the Creative Commons Attribution 4.0 International license. Further distribution of this work must maintain attribution to the author(s) and the published article's title, journal citation, and DOI.Published by the American Physical SocietyPhysics Subject Headings (PhySH)Research AreasCell signalingScientific reasoning & problem solvingSignal transductionPhysical SystemsCellsTechniquesInformation theoryNetwork ModelsTime series analysisStatistical PhysicsBiological PhysicsGeneral Physics
更多查看译文
AI 理解论文
溯源树
样例
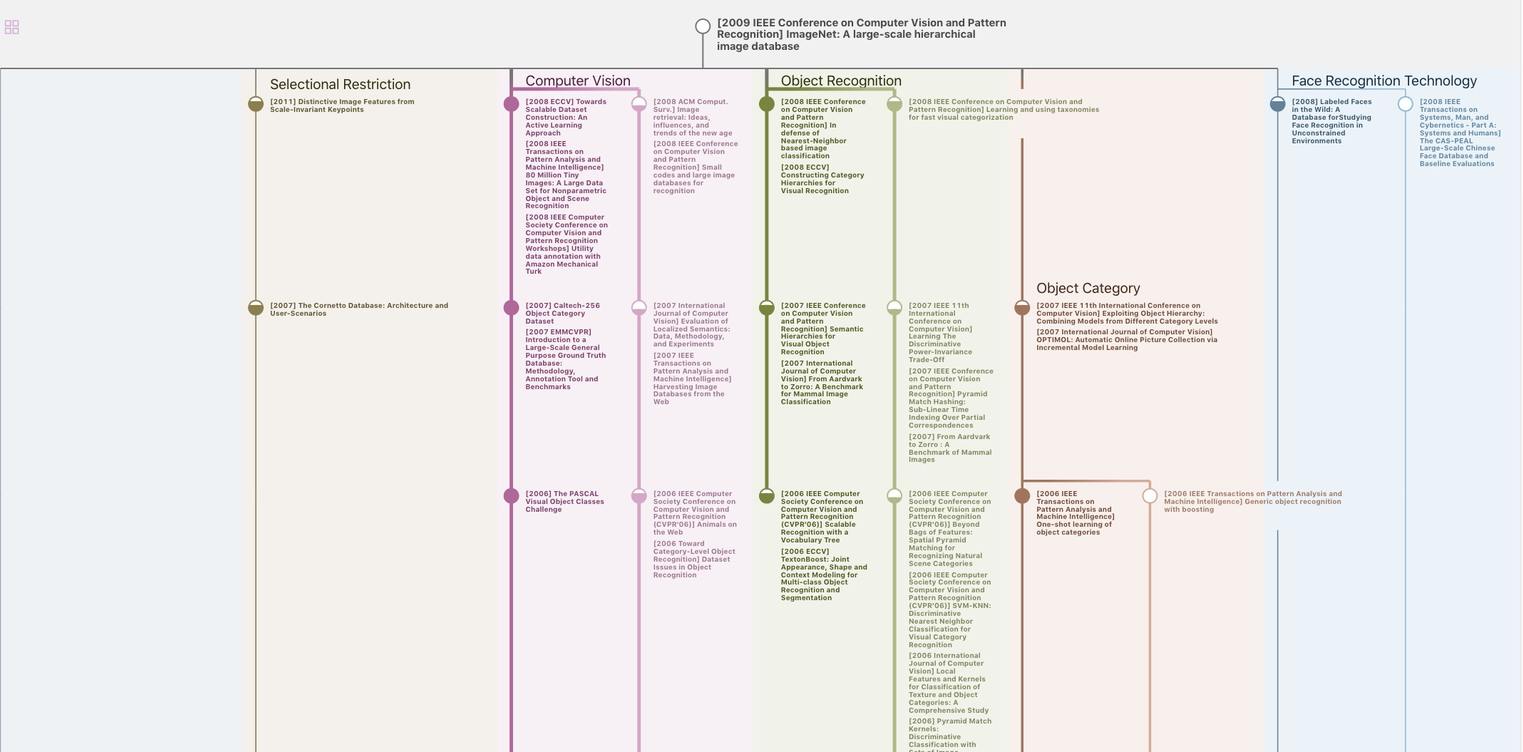
生成溯源树,研究论文发展脉络
Chat Paper
正在生成论文摘要