U-Net Based Segmentation and Characterization of Gliomas
CANCERS(2022)
摘要
Simple Summary Gliomas comprise 80% of all malignant brain tumors. We aimed to develop a deep learning-based framework for the automatic segmentation and characterization of gliomas. In this retrospective study, patients were included if they: (1) had a diagnosis of glioma confirmed by histopathology and (2) had preoperative MRI with the inclusion of FLAIR imaging. The deep learning-based U-Net framework was developed based on manual segmentation on FLAIR as the ground truth mask for automatic segmentation and feature extraction, which were used for the prediction of biomarker status and prognosis. A total of 208 patients were included from our internal dataset with stratified sampling to split the database into training and validation. An external dataset (n = 31) from an outside institution was used for testing. The dice similarity coefficient of the generated mask was 0.93 on the testing dataset. The prediction of the radiomic model achieved an AUC of 0.88 for IDH-1 and 0.62 for MGMT on the testing dataset. Our deep learning-based framework can detect and segment gliomas with excellent performance for the prediction of IDH-1 biomarker status and survival. (1) Background: Gliomas are the most common primary brain neoplasms accounting for roughly 40-50% of all malignant primary central nervous system tumors. We aim to develop a deep learning-based framework for automated segmentation and prediction of biomarkers and prognosis in patients with gliomas. (2) Methods: In this retrospective two center study, patients were included if they (1) had a diagnosis of glioma with known surgical histopathology and (2) had preoperative MRI with FLAIR sequence. The entire tumor volume including FLAIR hyperintense infiltrative component and necrotic and cystic components was segmented. Deep learning-based U-Net framework was developed based on symmetric architecture from the 512 x 512 segmented maps from FLAIR as the ground truth mask. (3) Results: The final cohort consisted of 208 patients with mean +/- standard deviation of age (years) of 56 +/- 15 with M/F of 130/78. DSC of the generated mask was 0.93. Prediction for IDH-1 and MGMT status had a performance of AUC 0.88 and 0.62, respectively. Survival prediction of <18 months demonstrated AUC of 0.75. (4) Conclusions: Our deep learning-based framework can detect and segment gliomas with excellent performance for the prediction of IDH-1 biomarker status and survival.
更多查看译文
关键词
deep learning, glioma, tumor segmentation, radiogenomic, isocitrate dehydrogenase 1, magnetic resonance imaging
AI 理解论文
溯源树
样例
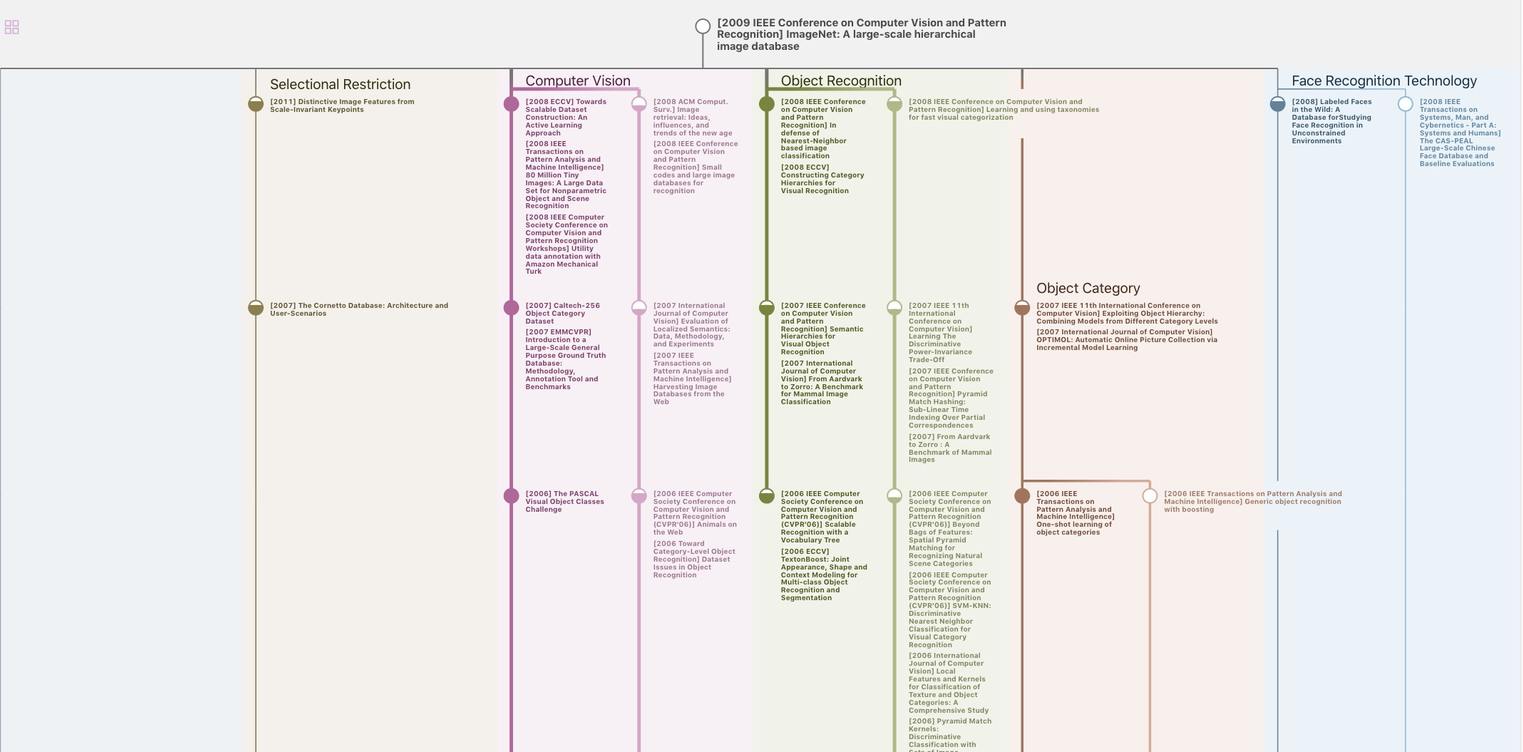
生成溯源树,研究论文发展脉络
Chat Paper
正在生成论文摘要