Disentangling Reasoning Capabilities from Language Models with Compositional Reasoning Transformers
conf_acl(2022)
摘要
This paper presents ReasonFormer, a unified reasoning framework for mirroring the modular and compositional reasoning process of humans in complex decision-making. Inspired by dual-process theory in cognitive science, the representation module (automatic thinking) and reasoning modules (controlled thinking) are decoupled to capture different levels of cognition. Upon the top of the representation module, the pre-trained reasoning modules are modular and professional in specific and fundamental reasoning skills (e.g., logic, simple QA, etc). To mimic the controlled compositional thinking process, different reasoning modules are dynamically activated and composed in both parallel and cascaded manners to control what reasoning skills are activated and how deep the reasoning process will be reached to solve the current problems. The unified reasoning framework solves multiple tasks with a single model, and is trained and inferred in an end-to-end manner. Evaluated on 11 datasets requiring different reasoning skills and complexity, ReasonFormer demonstrates substantial performance boosts, revealing the compositional reasoning ability. Few-shot experiments exhibit better generalization ability by learning to compose pre-trained skills for new tasks with limited data, and decoupling the representation module and the reasoning modules. Further analysis shows the modularity of reasoning modules as different tasks activate distinct reasoning skills at different reasoning depths.
更多查看译文
关键词
compositional reasoning transformers,reasoning capabilities,language models
AI 理解论文
溯源树
样例
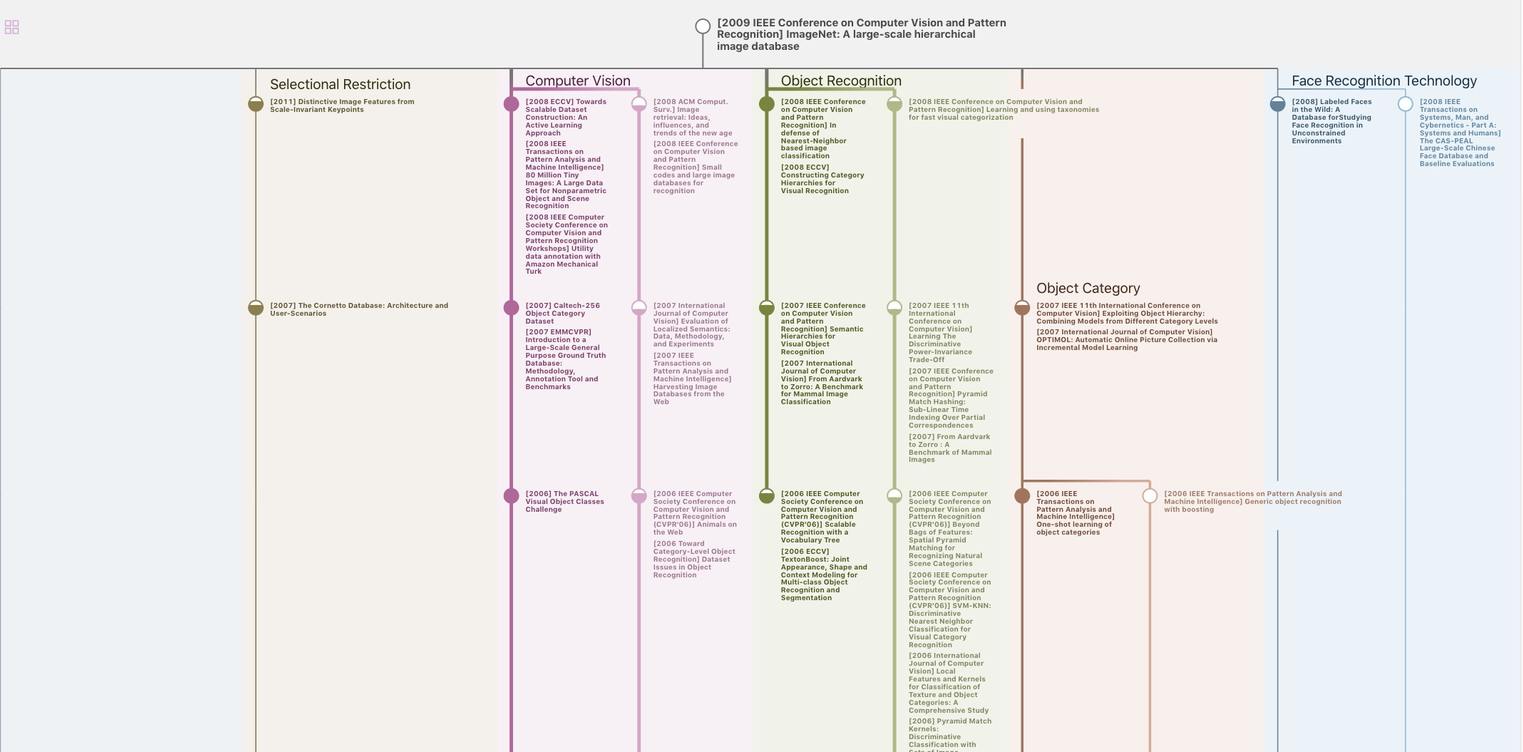
生成溯源树,研究论文发展脉络
Chat Paper
正在生成论文摘要