Multifidelity Model Calibration in Structural Dynamics Using Stochastic Variational Inference on Manifolds
ENTROPY(2022)
摘要
Bayesian techniques for engineering problems, which rely on Gaussian process (GP) regression, are known for their ability to quantify epistemic and aleatory uncertainties and for being data efficient. The mathematical elegance of applying these methods usually comes at a high computational cost when compared to deterministic and empirical Bayesian methods. Furthermore, using these methods becomes practically infeasible in scenarios characterized by a large number of inputs and thousands of training data. The focus of this work is on enhancing Gaussian process based metamodeling and model calibration tasks, when the size of the training datasets is significantly large. To achieve this goal, we employ a stochastic variational inference algorithm that enables rapid statistical learning of the calibration parameters and hyperparameter tuning, while retaining the rigor of Bayesian inference. The numerical performance of the algorithm is demonstrated on multiple metamodeling and model calibration problems with thousands of training data.
更多查看译文
关键词
Gaussian processes, stochastic variational inference, multifidelity modeling, manifold gradient ascent, structural dynamics, vibration torsion
AI 理解论文
溯源树
样例
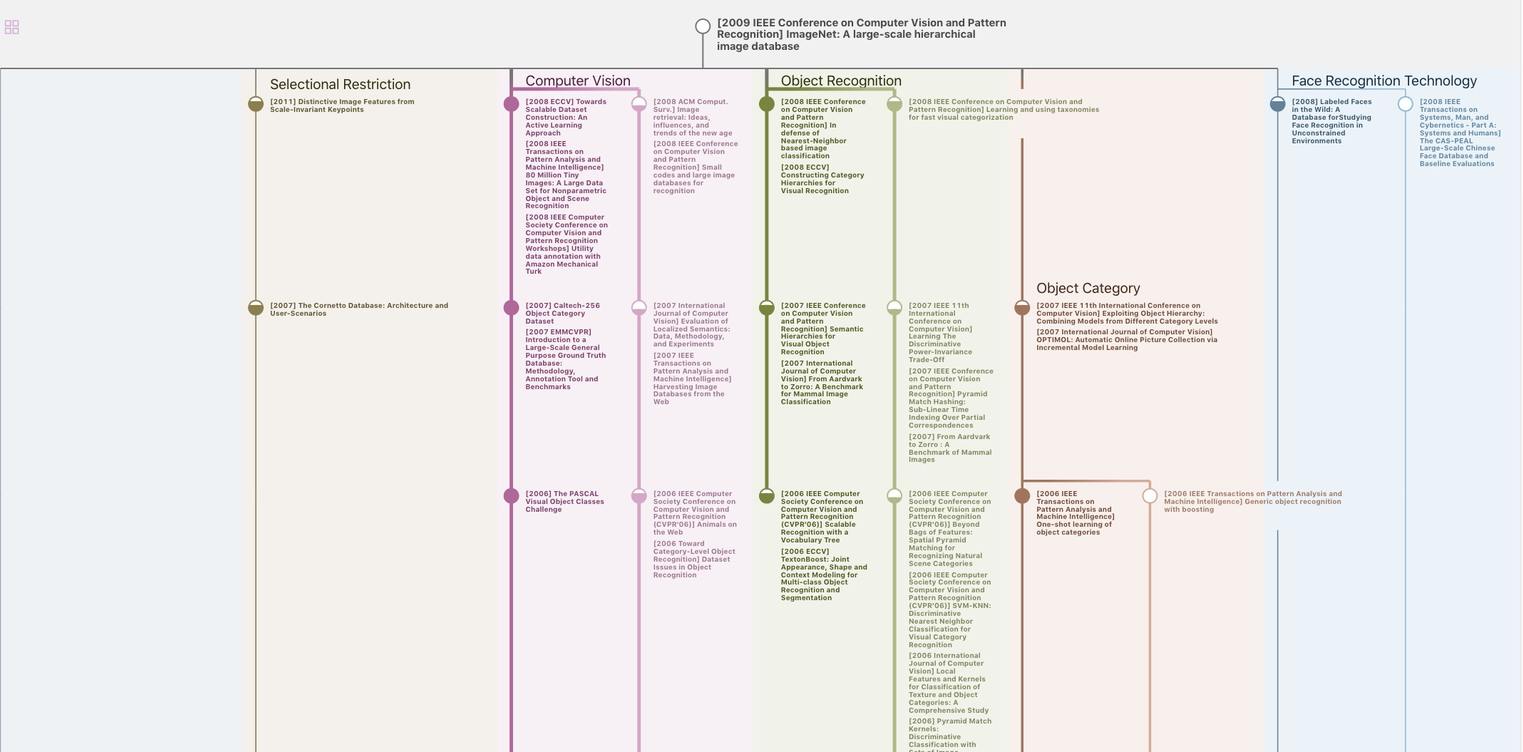
生成溯源树,研究论文发展脉络
Chat Paper
正在生成论文摘要