Rapid Profile Reconstruction of Phase Defects Via Machine-Learning Regression Model
INTERNATIONAL CONFERENCE ON EXTREME ULTRAVIOLET LITHOGRAPHY 2022(2022)
摘要
For imaging methods based on Fourier transform, such as coherent diffraction imaging (CDI), in the case where the sampling rate of the diffraction data satisfies Nyquist sampling theorem, phase retrieval algorithm can effectively recover the phase lost in the image acquisition process. In general, ptychography is used to ensure the adequate sample. But in the imaging system of EUV mask defect inspection, the acquisition of more sampling data requires multiple exposures of the mask, which increases the sampling time resulting in low throughput and causes mask irradiation damage. To solve the problem, we propose a machine learning scheme to reconstruct the profile of phase defect using a single diffraction intensity. Combining the physical model of CDI and neural network framework, the method transforms the phase retrieval problem into a regression model of machine learning. Integrating the constraints of the traditional CDI and the gradient descent algorithms in neural network framework, our approach can accelerate the convergence of the model. The performance of the method is confirmed by comparing with the conventional iterative method in the condition of sufficient sampling rate. This novel method provides a new idea of combining computational imaging with machine learning, which can simplify the data acquisition process and accelerate the iteration of algorithm in EUV mask defect inspection.
更多查看译文
关键词
EUV mask inspection,CDI,phase retrieval algorithm,machine learning
AI 理解论文
溯源树
样例
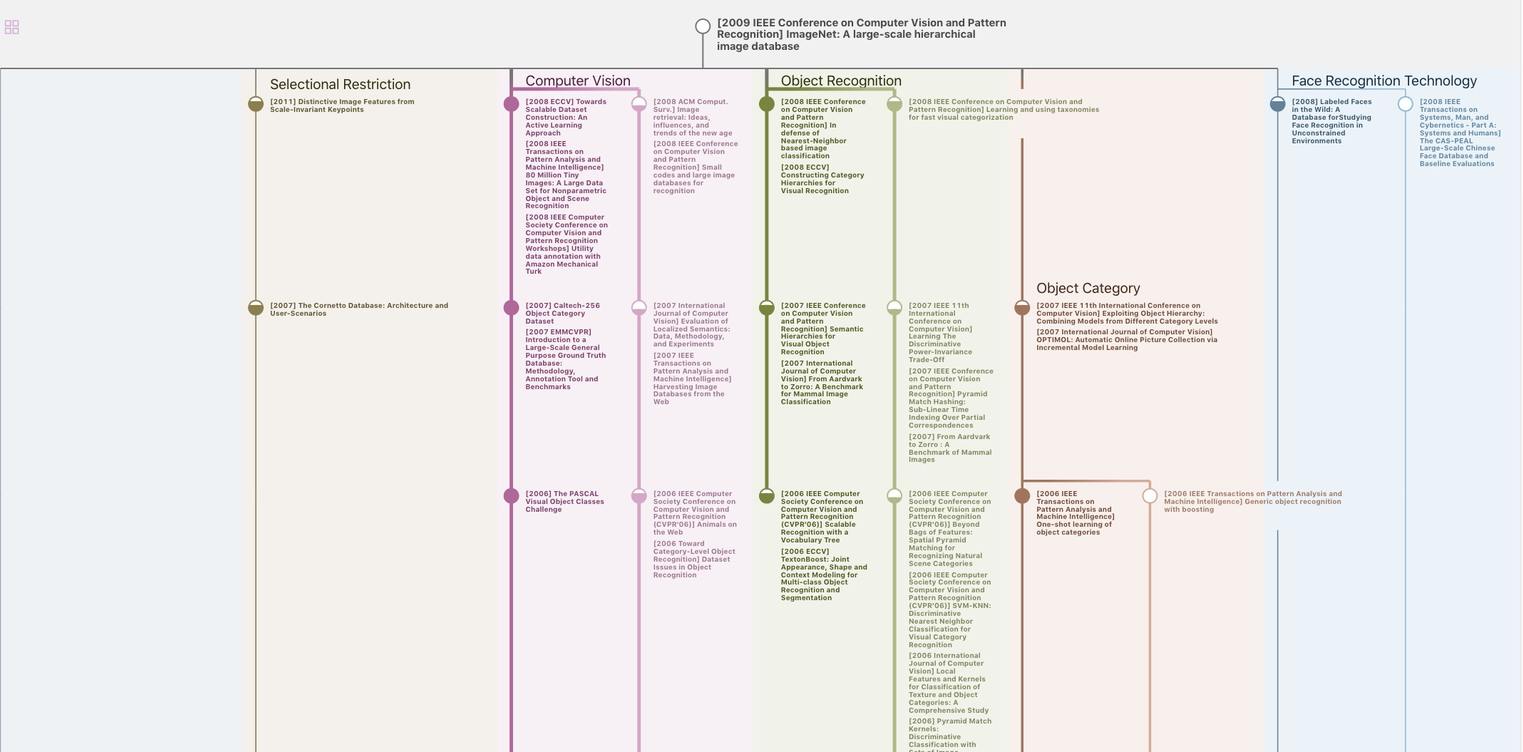
生成溯源树,研究论文发展脉络
Chat Paper
正在生成论文摘要