Usability of Hospital Price Estimators for Lumbar Spine MRI
Journal of the American College of Radiology(2022)
摘要
Purpose
The aim of this study was to evaluate the usability of online hospital price estimators for a common imaging examination using surrogate patients.
Methods
Using the Amazon Mechanical Turk platform, the authors recruited adult English-speaking US residents as surrogate patients to find the cash price for a noncontrast lumbar spine MRI examination for a self-pay patient using price estimator tools at four hospitals. All were asked to view a 3-min tutorial video and report their experiences with the task, including the System Usability Scale (SUS) for the estimator, through a paid survey. Participants were queried about demographics, insurance, prior imaging exposure, and assessed health literacy and health insurance literacy using validated measures. Multivariable analysis for correct price identification and price estimator SUS were performed.
Results
Of 660 respondents, 476 met eligibility criteria (72.7% <45 years of age, 41.7% female, 86.1% white); 76.9% found all four estimators, and 9.2% were unable to locate any. Only 27.7% found the correct price at all four hospitals, with 67.4% being able to find at least one correct cash price. Average SUS score for the hospitals’ estimators ranged between 62.4 and 77.5. The hospital with a similar estimator to that used in the tutorial video had the highest SUS score. Accuracy of price identification improved with later tasks. Higher health insurance literacy was associated with higher identification of at least one correct price (odds ratio, 1.21; 95% confidence interval, 1.02-1.44) and higher SUS score (B = 1.68; 95% confidence interval, 1.07-2.29).
Conclusions
Surrogate patients were able to locate hospital price estimators but unable to effectively use them to obtain correct prices. Tutorial videos improved SUS score, but correct price identification improved with practice.
更多查看译文
关键词
Price transparency,usability,medical imaging
AI 理解论文
溯源树
样例
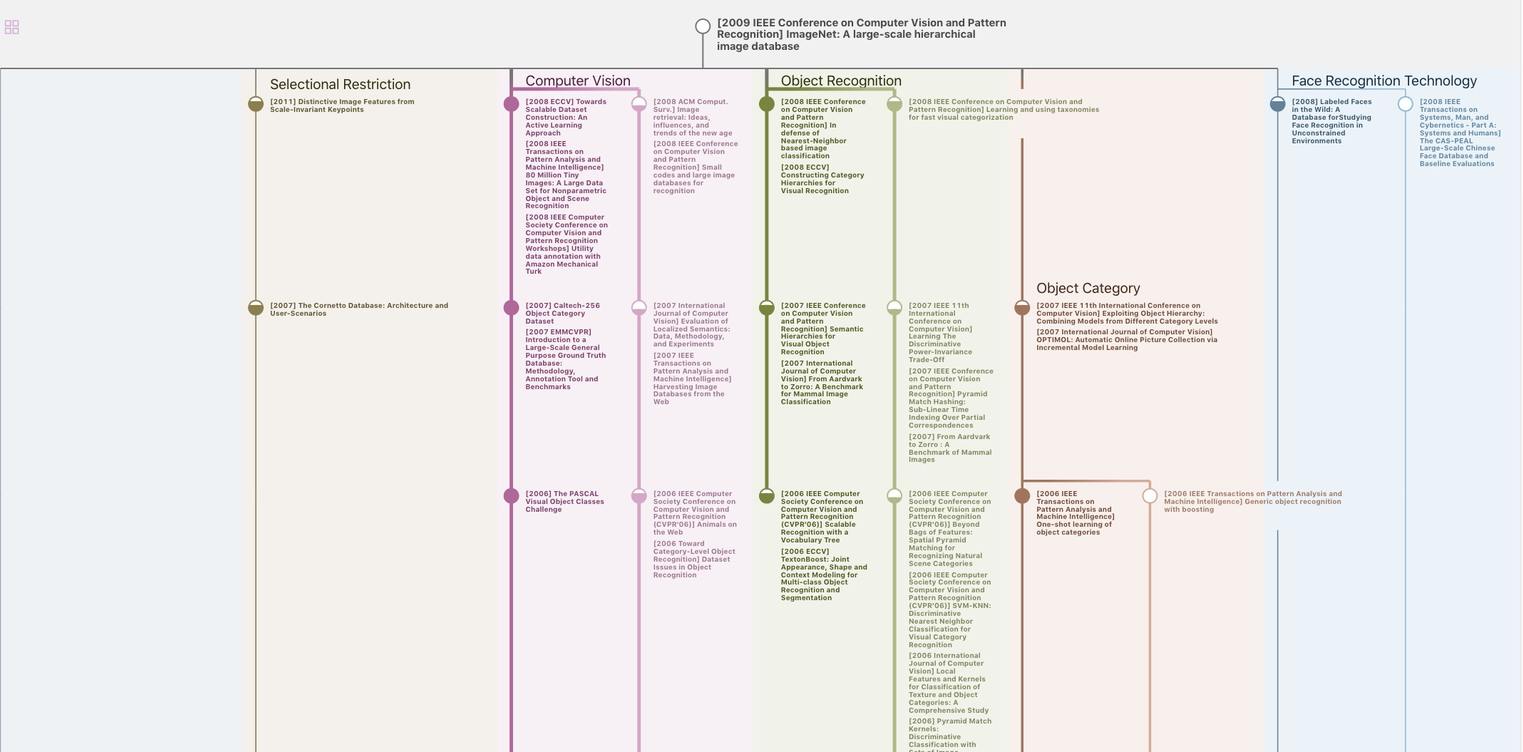
生成溯源树,研究论文发展脉络
Chat Paper
正在生成论文摘要