An efficient algorithm for fair multi-agent multi-armed bandit with low regret
arxiv(2023)
摘要
Recently a multi-agent variant of the classical multi-armed bandit was proposed to tackle fairness issues in online learning. Inspired by a long line of work in social choice and economics, the goal is to optimize the Nash social welfare instead of the total utility. Unfortunately previous algorithms either are not efficient or achieve sub-optimal regret in terms of the number of rounds. We propose a new efficient algorithm with lower regret than even previous inefficient ones. We also complement our efficient algorithm with an inefficient approach with regret that matches the lower bound for one agent. The experimental findings confirm the effectiveness of our efficient algorithm compared to the previous approaches.
更多查看译文
关键词
efficient algorithm,fair,multi-agent,multi-armed
AI 理解论文
溯源树
样例
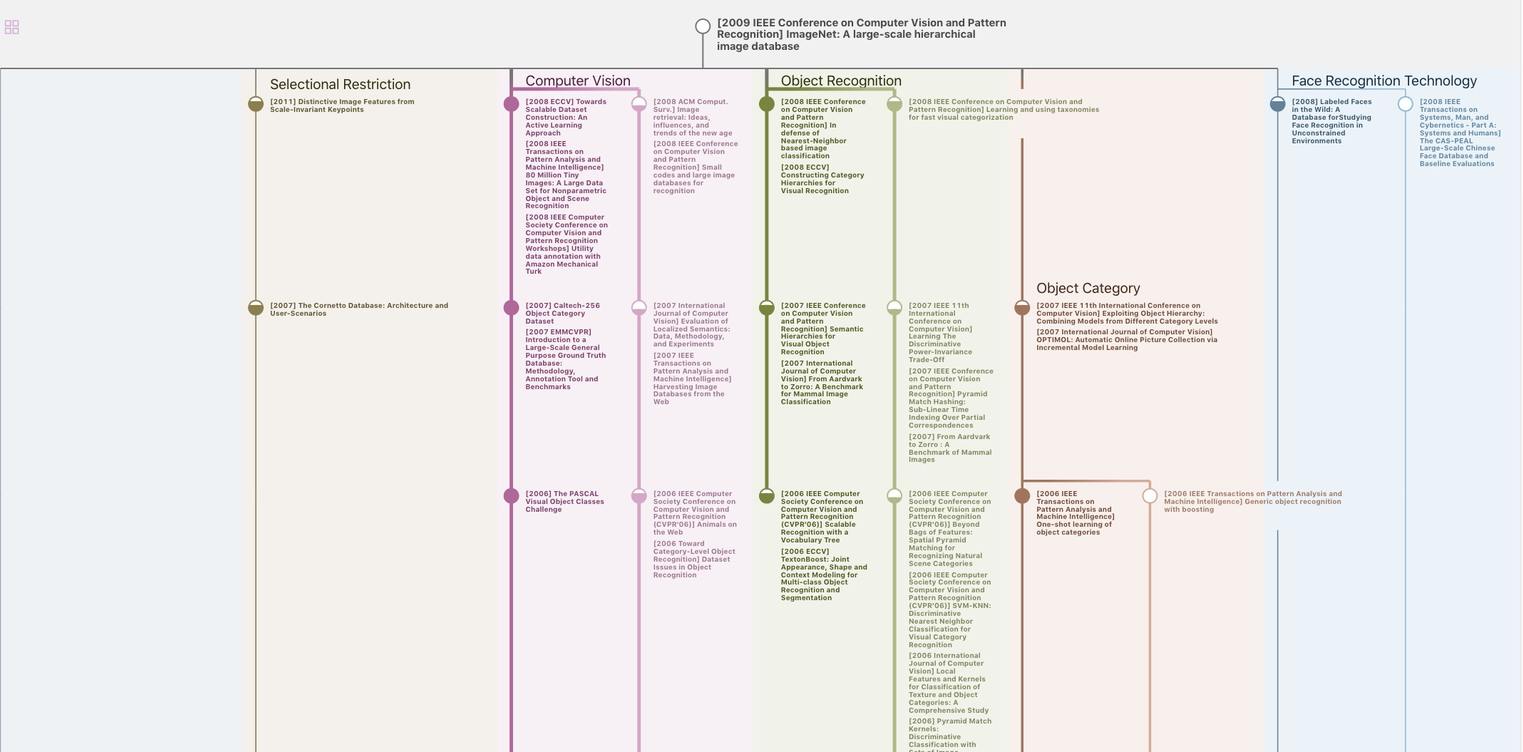
生成溯源树,研究论文发展脉络
Chat Paper
正在生成论文摘要