Learn what matters: cross-domain imitation learning with task-relevant embeddings
NeurIPS 2022(2022)
摘要
We study how an autonomous agent learns to perform a task from demonstrations in a different domain, such as a different environment or different agent. Such cross-domain imitation learning is required to, for example, train an artificial agent from demonstrations of a human expert. We propose a scalable framework that enables cross-domain imitation learning without access to additional demonstrations or further domain knowledge. We jointly train the learner agent's policy and learn a mapping between the learner and expert domains with adversarial training. We effect this by using a mutual information criterion to find an embedding of the expert's state space that contains task-relevant information and is invariant to domain specifics. This step significantly simplifies estimating the mapping between the learner and expert domains and hence facilitates end-to-end learning. We demonstrate successful transfer of policies between considerably different domains, without extra supervision such as additional demonstrations, and in situations where other methods fail.
更多查看译文
关键词
Reinforcement Learning,Learning from Observations,Inverse Reinforcement Learning,Imitation Learning,Domain Transfer
AI 理解论文
溯源树
样例
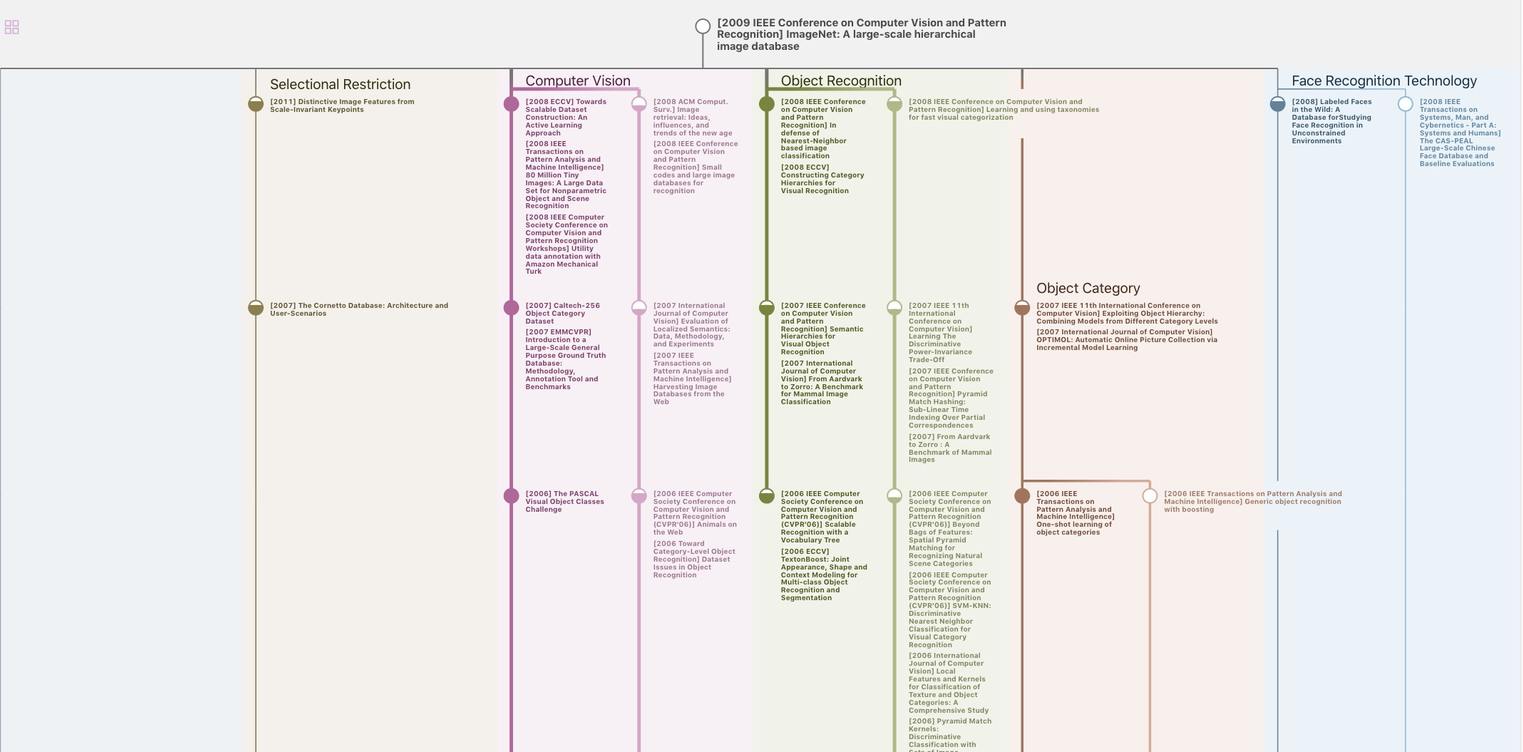
生成溯源树,研究论文发展脉络
Chat Paper
正在生成论文摘要