Re-contextualizing Fairness in NLP: The Case of India.
AACL/IJCNLP (1)(2022)
Abstract
Recent research has revealed undesirable biases in NLP data and models. However, these efforts focus on social disparities in West, and are not directly portable to other geo-cultural contexts. In this paper, we focus on NLP fair-ness in the context of India. We start with a brief account of the prominent axes of social disparities in India. We build resources for fairness evaluation in the Indian context and use them to demonstrate prediction biases along some of the axes. We then delve deeper into social stereotypes for Region andReligion, demonstrating its prevalence in corpora and models. Finally, we outline a holistic research agenda to re-contextualize NLP fairness research for the Indian context, ac-counting for Indian societal context, bridging technological gaps in NLP capabilities and re-sources, and adapting to Indian cultural values. While we focus on India, this framework can be generalized to other geo-cultural contexts.
MoreTranslated text
AI Read Science
Must-Reading Tree
Example
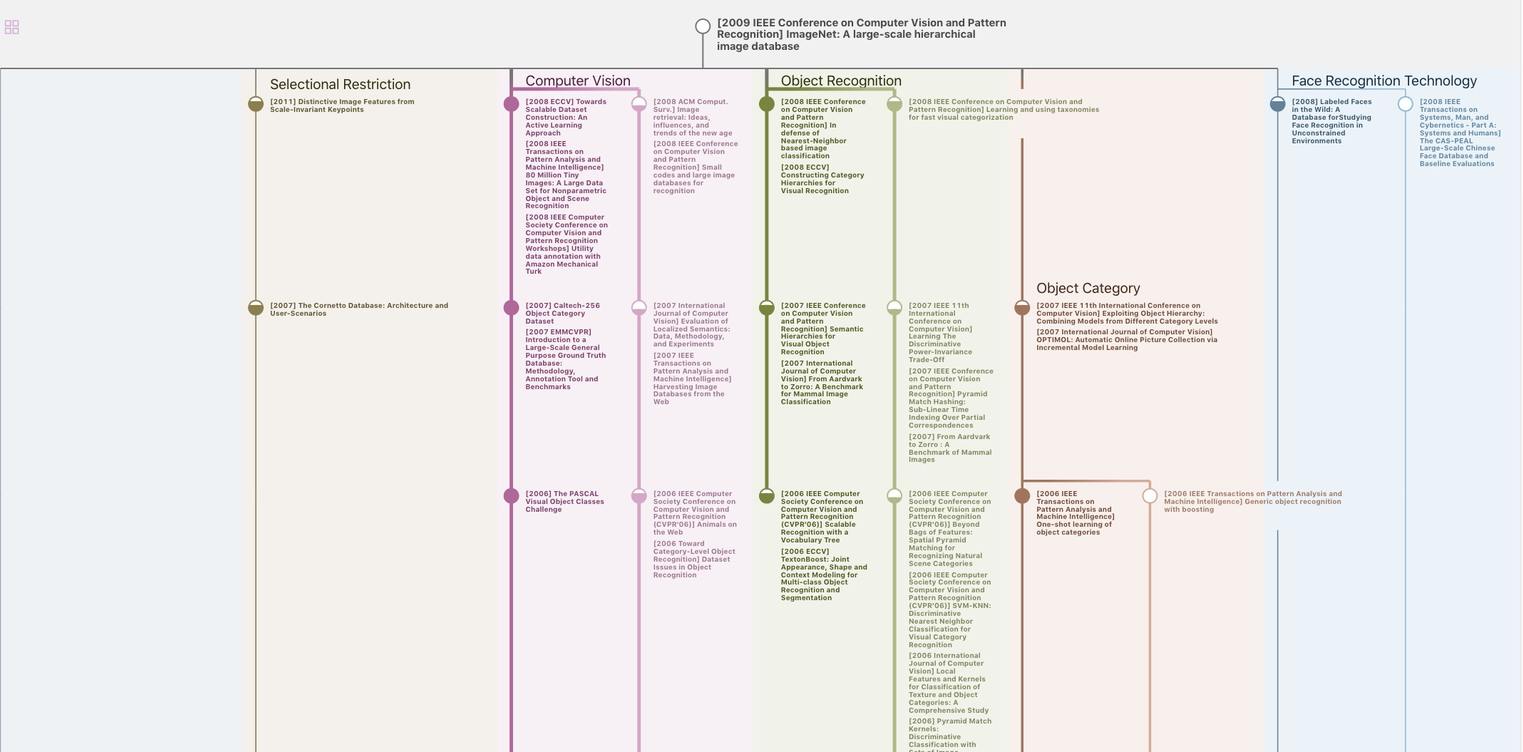
Generate MRT to find the research sequence of this paper
Chat Paper
Summary is being generated by the instructions you defined