Adnexal Mass Segmentation with Ultrasound Data Synthesis
SIMPLIFYING MEDICAL ULTRASOUND, ASMUS 2022(2022)
摘要
Ovarian cancer is the most lethal gynaecological malignancy. The disease is most commonly asymptomatic at its early stages and its diagnosis relies on expert evaluation of transvaginal ultrasound images. Ultrasound is the first-line imaging modality for characterising adnexal masses, it requires significant expertise and its analysis is subjective and labour-intensive, therefore open to error. Hence, automating processes to facilitate and standardise the evaluation of scans is desired in clinical practice. Using supervised learning, we have demonstrated that segmentation of adnexal masses is possible, however, prevalence and label imbalance restricts the performance on under-represented classes. To mitigate this we apply a novel pathology-specific data synthesiser. We create synthetic medical images with their corresponding ground truth segmentations by using Poisson image editing to integrate less common masses into other samples. Our approach achieves the best performance across all classes, including an improvement of up to 8% when compared with nnU-Net baseline approaches.
更多查看译文
关键词
ultrasound,segmentation
AI 理解论文
溯源树
样例
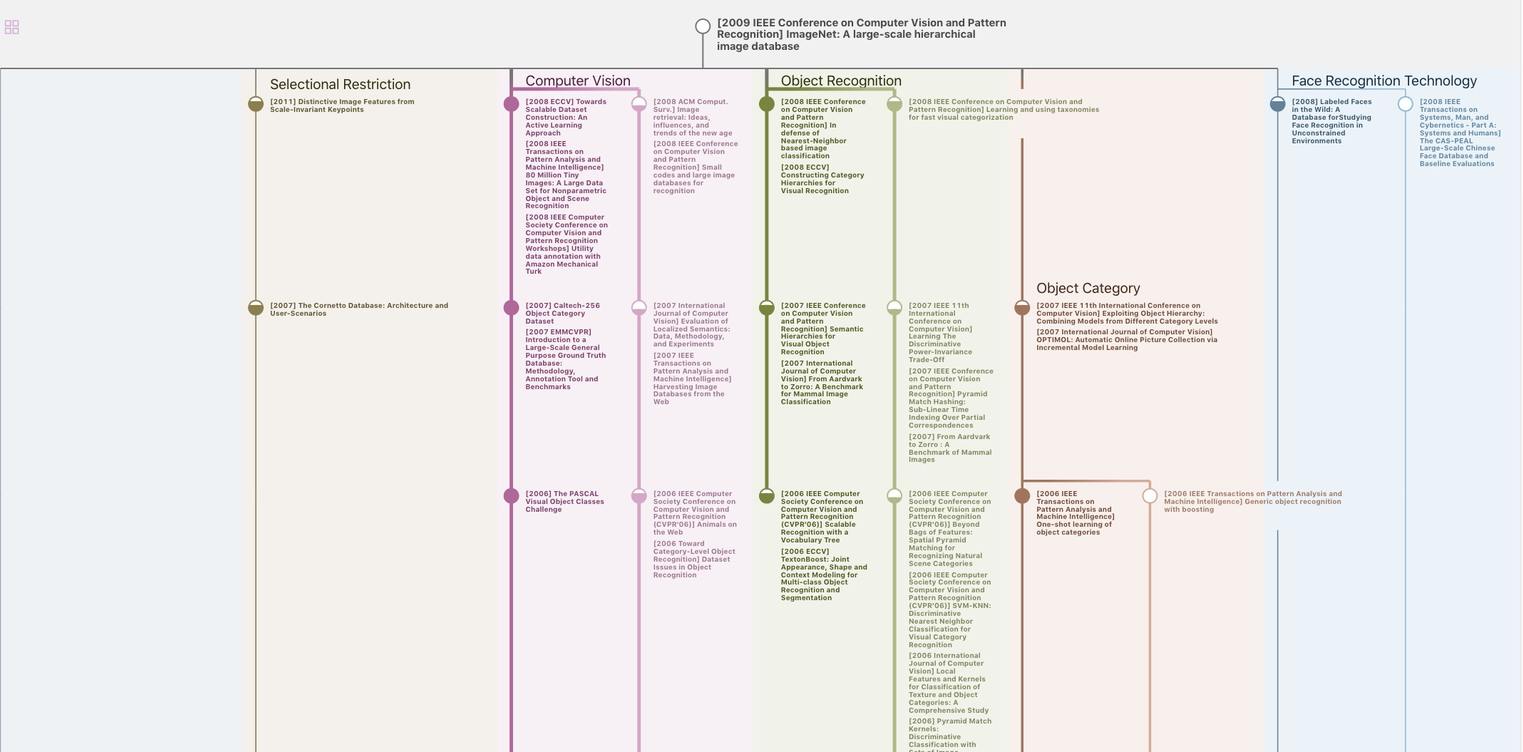
生成溯源树,研究论文发展脉络
Chat Paper
正在生成论文摘要