Joint task offloading and resource optimization in NOMA-based vehicular edge computing: A game-theoretic DRL approach
Journal of Systems Architecture(2023)
摘要
Vehicular edge computing (VEC) becomes a promising paradigm for the development of emerging intelligent transportation systems. Nevertheless, the limited resources and massive transmission demands bring great challenges on implementing vehicular applications with stringent deadline requirements. This work presents a non-orthogonal multiple access (NOMA) based architecture in VEC, where heterogeneous edge nodes are cooperated for real-time task processing. We derive a vehicle-to-infrastructure (V2I) transmission model by considering both intra-edge and inter-edge interferences and formulate a cooperative resource optimization (CRO) problem by jointly optimizing the task offloading and resource allocation, aiming at maximizing the service ratio. Further, we decompose the CRO into two subproblems, namely, task offloading and resource allocation. In particular, the task offloading subproblem is modeled as an exact potential game (EPG), and a multi-agent distributed distributional deep deterministic policy gradient (MAD4PG) is proposed to achieve the Nash equilibrium. The resource allocation subproblem is divided into two independent convex optimization problems, and an optimal solution is proposed by using a gradient-based iterative method and KKT condition. Finally, we build the simulation model based on real-world vehicular trajectories and give a comprehensive performance evaluation, which conclusively demonstrates the superiority of the proposed solutions.
更多查看译文
关键词
Vehicular edge computing,Real-time task offloading,Heterogeneous resource allocation,Deep reinforcement learning,Exact potential game
AI 理解论文
溯源树
样例
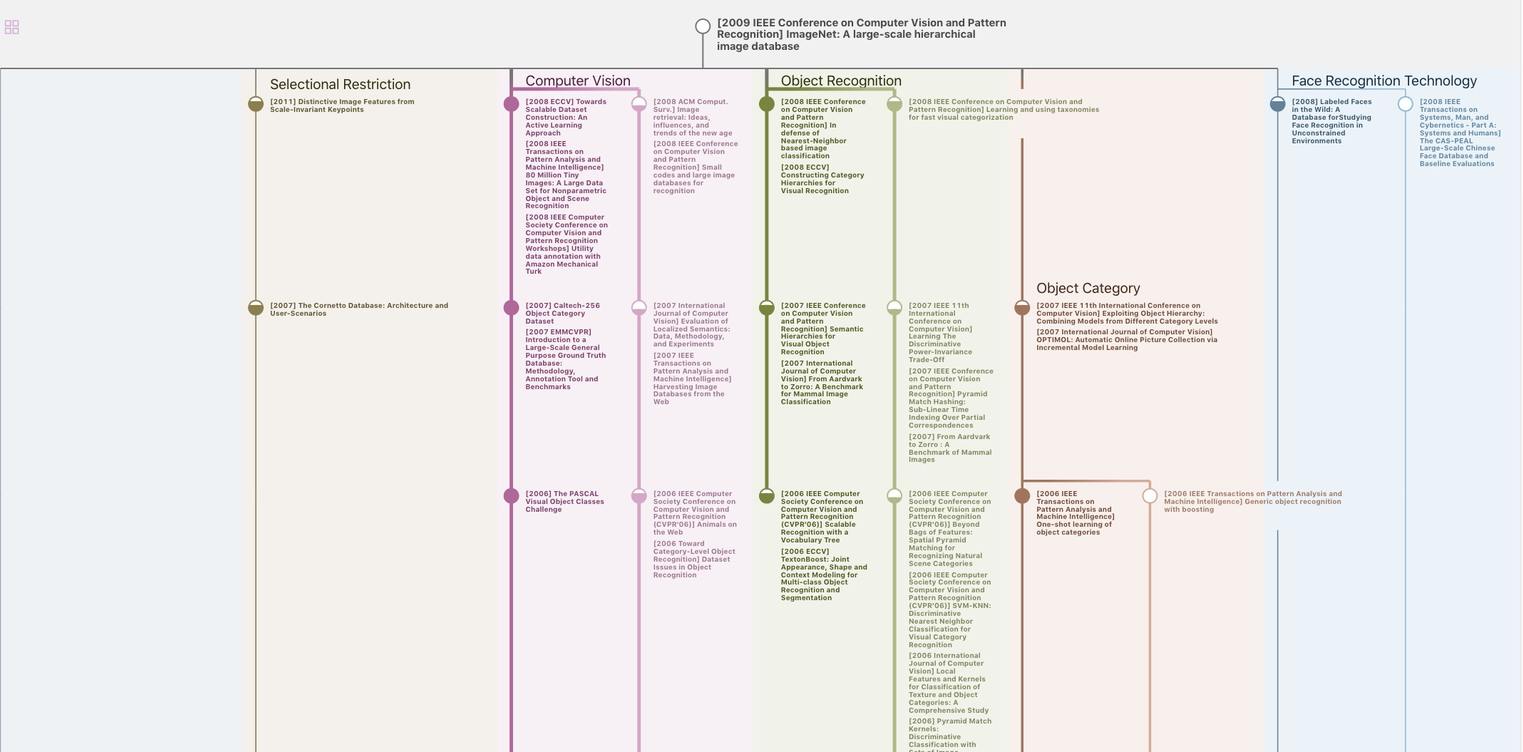
生成溯源树,研究论文发展脉络
Chat Paper
正在生成论文摘要