MalWhiteout: Reducing Label Errors in Android Malware Detection
ASE 2022(2022)
摘要
Machine learning based Android malware detection has attracted a great deal of research work in recent years. A reliable malware dataset is critical to evaluate the effectiveness of malware detection approaches. Unfortunately, existing malware datasets used in our community are mainly labelled by taking advantage of existing anti-virus services (i.e., VirusTotal), which are prone to mislabelling. This, however, would lead to the inaccurate evaluation of the malware detection techniques. Removing the label noises from Android malware datasets can be quite challenging, especially at a large data scale. To address this problem, we propose an effective approach called MalWhiteout to reduce the label errors in Android malware datasets. Specifically, we creatively introduce Confident Learning (CL), an advanced noise estimation approach, to the domain of Android malware detection. To combat false positives introduced by CL, we incorporate the idea of ensemble learning and inter-app relation to achieve a more robust capability in noise detection. We evaluate MalWhiteout on a curated large-scale and reliable benchmark dataset. Experimental results show that MalWhiteout is capable of detecting label noises with over 94% accuracy even at a high noise ratio (i.e., 30%) of the dataset. MalWhiteout outperforms the state-of-the-art approach in terms of both effectiveness (8% to 218% improvement) and efficiency (70 to 249 times faster) across different settings. By reducing label noises, we further show that the performance of existing malware detection approaches can be improved.
更多查看译文
关键词
label noise, Android malware detection, confident learning
AI 理解论文
溯源树
样例
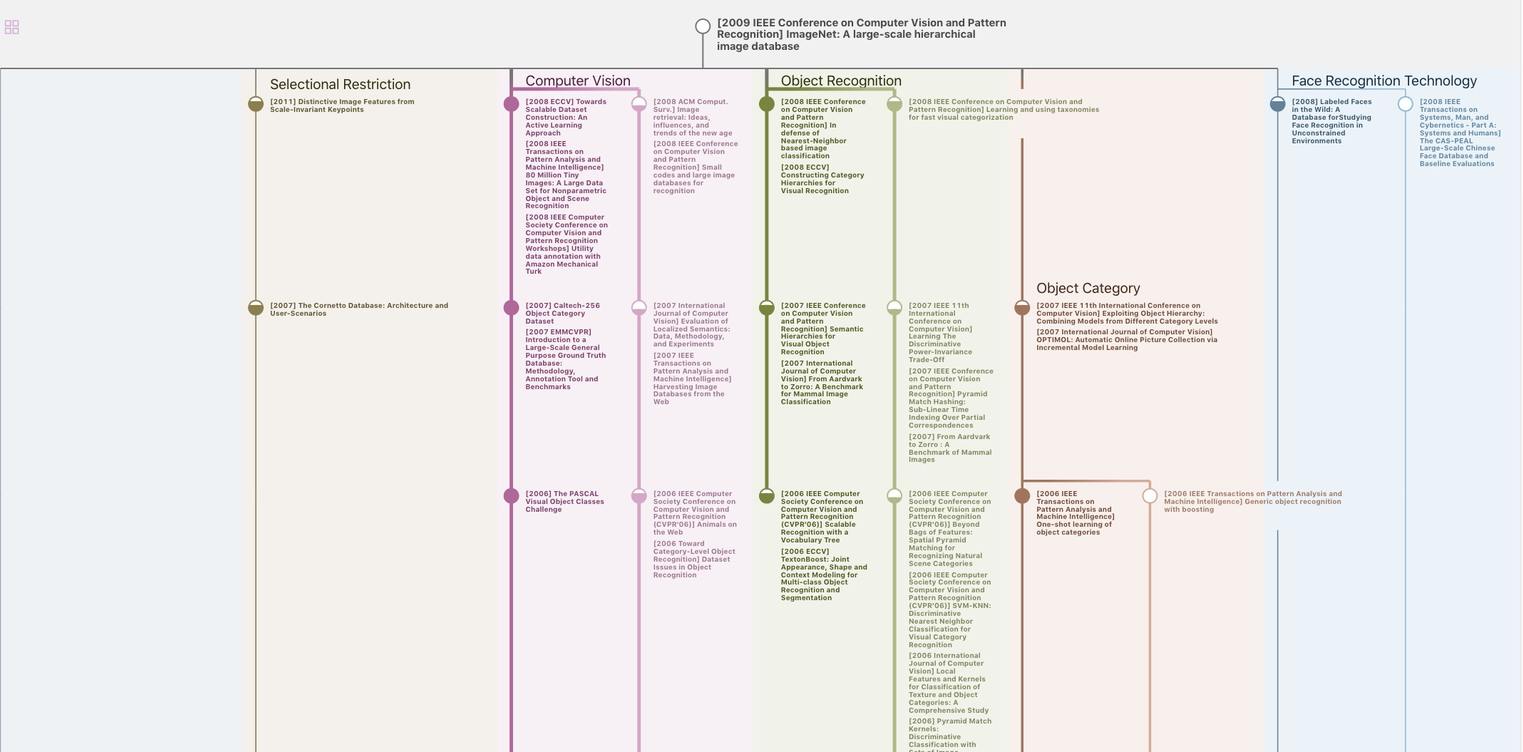
生成溯源树,研究论文发展脉络
Chat Paper
正在生成论文摘要