Tasking and Estimation for Minimum-Time Space Object Search and Recovery
The Journal of the Astronautical Sciences(2022)
摘要
A critical challenge in Space Domain Awareness (SDA) is gaining custody of newly detected or maneuvering objects. The dynamically feasible location of one such object may be represented as an admissible region or reachable set. This paper proposes techniques for exploring search sets using sample-based planning. Several methods for sampling feasible regions of measurement space are developed, and Monte Carlo Tree Search (MCTS) is applied over a limited horizon to determine time-optimal sets of actions. Tree search aids in overcoming local minima in solutions found with traditional optimization methods. With tasking methodologies in place, a second contribution of this paper explores estimation methodologies in the presence of null detections; these developments are critical to the search and recovery problem, in which the target probability density projected to measurement space is generally much larger than the sensor field of view. A special focus is applied to the admissible region, and given a Gaussian mixture representation of the admissible region, a novel methodology for splitting mixands in an arbitrary measurement space is presented. A mixand weight update is derived for the key scenario in which no detection is made at a measurement epoch. Merging methodologies are applied, and the resultant Gaussian sum filter is demonstrated for a representative case in which follow-on tracking of a geostationary object is desired.
更多查看译文
关键词
Space domain awareness,Sensor tasking,Monte Carlo Tree Search,Gaussian sum filter,Negative information
AI 理解论文
溯源树
样例
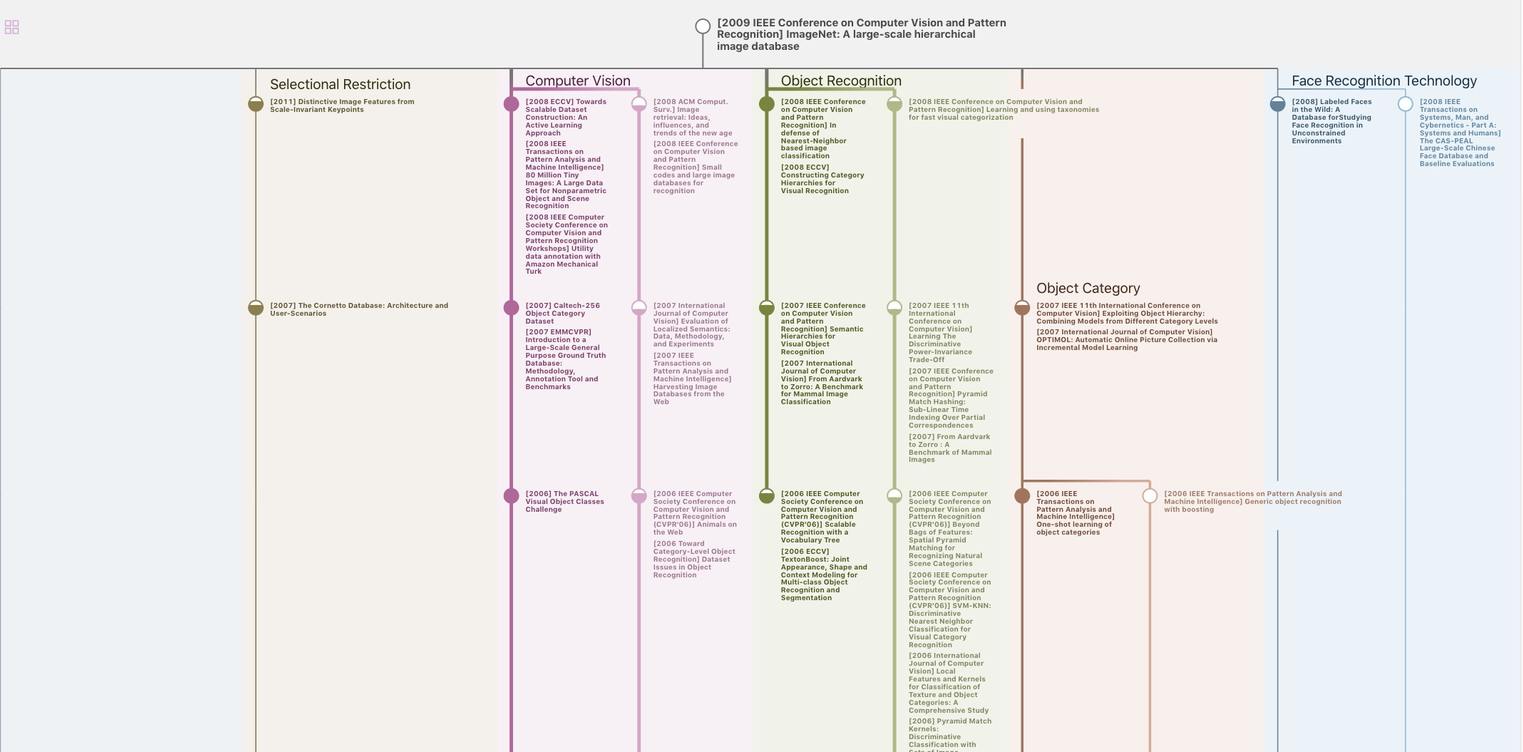
生成溯源树,研究论文发展脉络
Chat Paper
正在生成论文摘要