Short-Term Power Load Forecasting Model Based on t-SNE Dimension Reduction Visualization Analysis, VMD and LSSVM Improved with Chaotic Sparrow Search Algorithm Optimization
Journal of Electrical Engineering & Technology(2022)
摘要
The stable operation of power system has the strong constraint of load balance. Accurate power load forecasting is of great significance in ensuring power system planning and reliable and economic operation. For this purpose, a novel power load forecasting integrating variational modal decomposition (VMD), t-distributed stochastic neighbor embedding dimension reduction visualization analysis (t-SNE), compound prediction models adopting least squares support vector machine (LSSVM) and Tent mapping function as well as chaotic sparrow search algorithm (CSSA), is proposed in this paper. To begin with, for the high-dimensional meteorological data affecting the power load forecasting, the t-SNE is adopted. Meanwhile, the comparison experiments with five common dimensional reduction algorithms prove that t-SNE can better map high-dimensional meteorological data to low-dimensional space. Then, the VMD is used to decompose the electricity load, which decomposes the non-stationary electricity load series into multiple sets of relatively stationary sub-series. Meanwhile, key parameters in the LSSVM model are optimized using the CSSA optimization algorithm under Tent chaotic perturbation, and the component is predicted by optimized LSSVM model. Finally, the ultimate forecasting results of the electricity load are calculated by superimposing the predicted values of all components. The experiments results reveal that the proposed model provides competitive advantages over other models and offers greater prediction accuracy.
更多查看译文
关键词
Short-term electricity load forecasting, t-Distributed stochastic neighbor embedding, Variational mode decomposition, Chaotic sparrow search algorithm, Least squares support vector machine
AI 理解论文
溯源树
样例
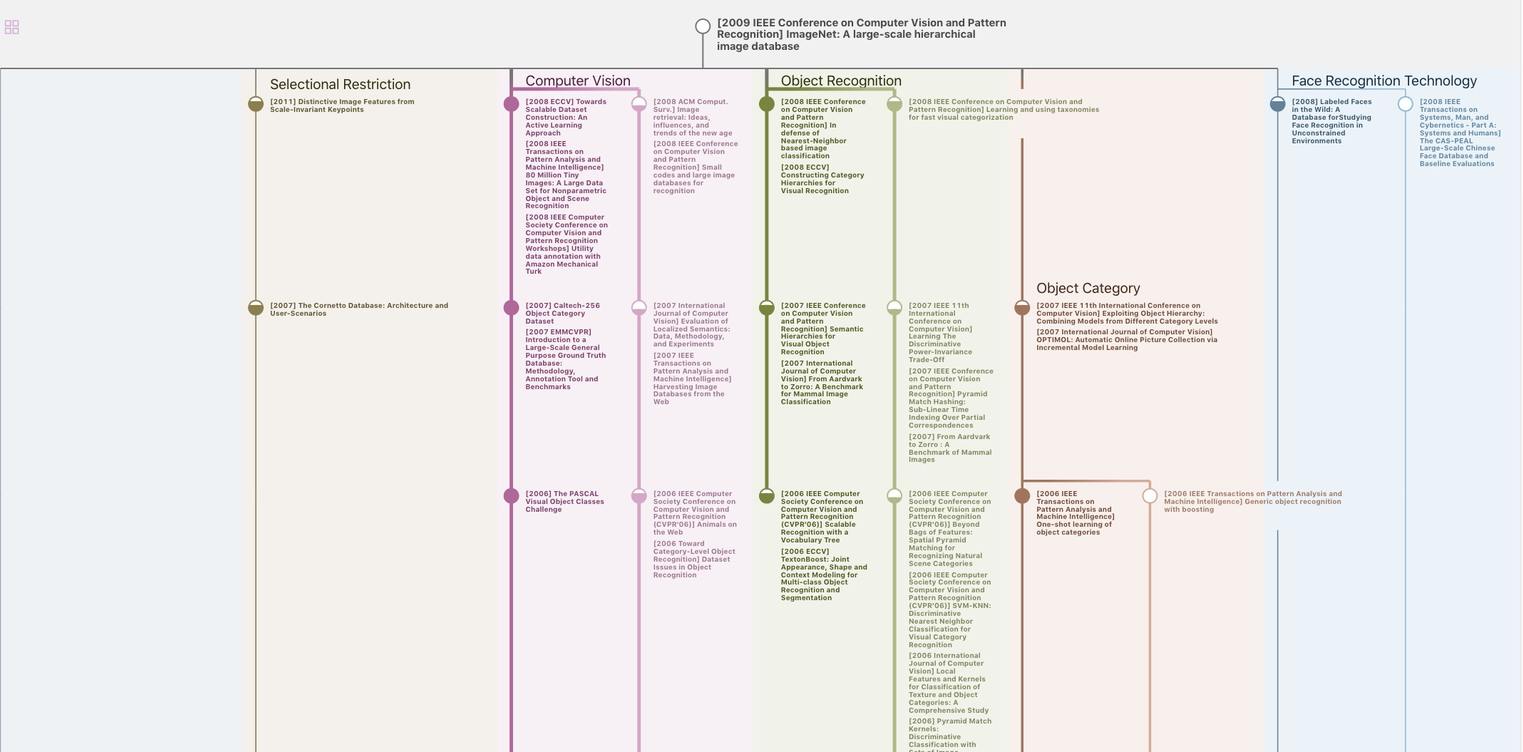
生成溯源树,研究论文发展脉络
Chat Paper
正在生成论文摘要