A large TV dataset for speech and music activity detection
EURASIP Journal on Audio, Speech, and Music Processing(2022)
摘要
Automatic speech and music activity detection (SMAD) is an enabling task that can help segment, index, and pre-process audio content in radio broadcast and TV programs. However, due to copyright concerns and the cost of manual annotation, the limited availability of diverse and sizeable datasets hinders the progress of state-of-the-art (SOTA) data-driven approaches. We address this challenge by presenting a large-scale dataset containing Mel spectrogram, VGGish, and MFCCs features extracted from around 1600 h of professionally produced audio tracks and their corresponding noisy labels indicating the approximate location of speech and music segments. The labels are several sources such as subtitles and cuesheet. A test set curated by human annotators is also included as a subset for evaluation. To validate the generalizability of the proposed dataset, we conduct several experiments comparing various model architectures and their variants under different conditions. The results suggest that our proposed dataset is able to serve as a reliable training resource and leads to SOTA performances on various public datasets. To the best of our knowledge, this dataset is the first large-scale, open-sourced dataset that contains features extracted from professionally produced audio tracks and their corresponding frame-level speech and music annotations.
更多查看译文
关键词
Speech and music activation detection,Dataset,Production TV audio
AI 理解论文
溯源树
样例
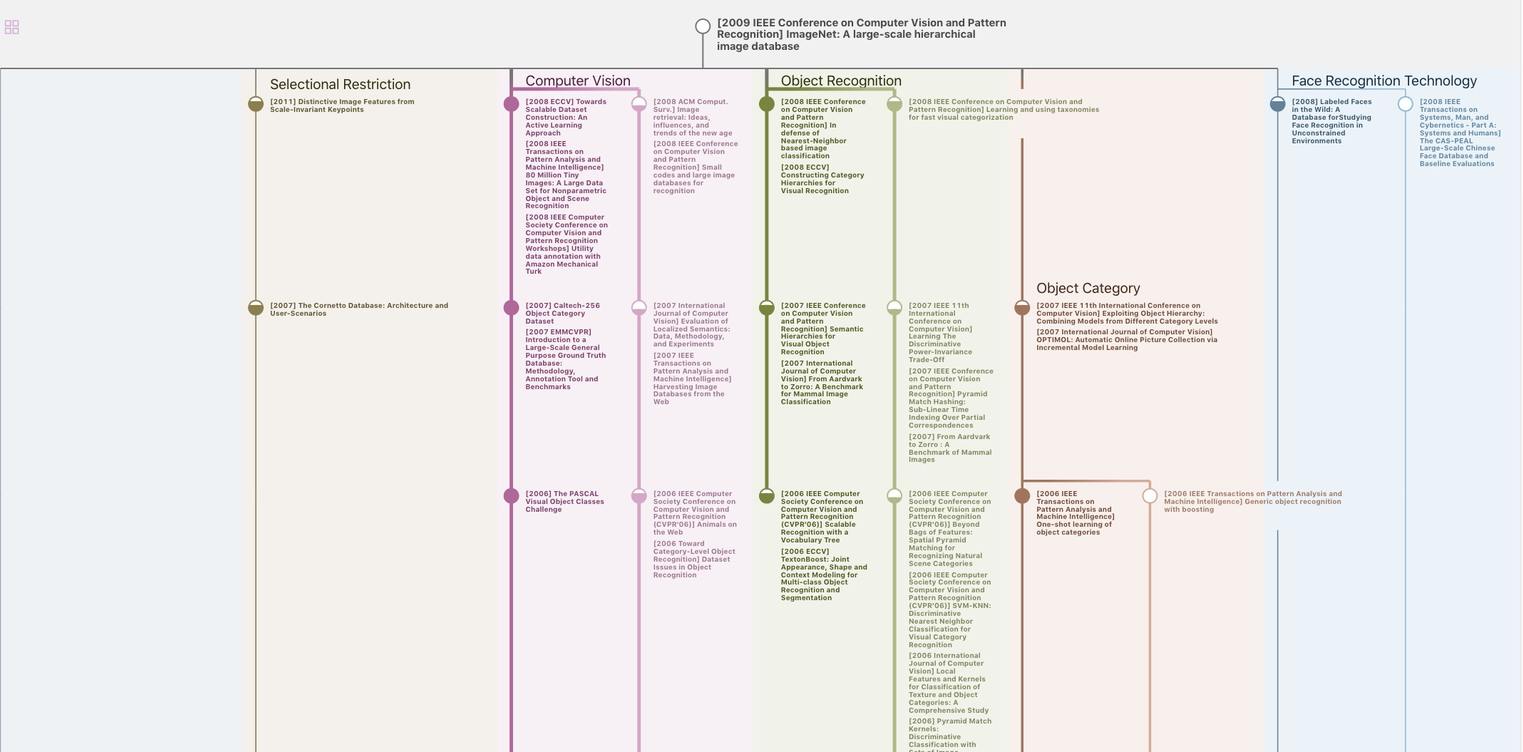
生成溯源树,研究论文发展脉络
Chat Paper
正在生成论文摘要