Bayes goes fast: Uncertainty quantification for a covariant energy density functional emulated by the reduced basis method
arxiv(2023)
摘要
A covariant energy density functional is calibrated using a principled Bayesian statistical framework informed by experimental binding energies and charge radii of several magic and semi-magic nuclei. The Bayesian sampling required for the calibration is enabled by the emulation of the high-fidelity model through the implementation of a reduced basis method (RBM)-a set of dimensionality reduction techniques that can speed up demanding calculations involving partial differential equations by several orders of magnitude. The RBM emulator we build-using only 100 evaluations of the high-fidelity model-is able to accurately reproduce the model calculations in tens of milliseconds on a personal computer, an increase in speed of nearly a factor of 3,300 when compared to the original solver. Besides the analysis of the posterior distribution of parameters, we present model calculations for masses and radii with properly estimated uncertainties. We also analyze the model correlation between the slope of the symmetry energy L and the neutron skin of Ca-48 and Pb-208. The straightforward implementation and outstanding performance of the RBM makes it an ideal tool for assisting the nuclear theory community in providing reliable estimates with properly quantified uncertainties of physical observables. Such uncertainty quantification tools will become essential given the expected abundance of data from the recently inaugurated and future experimental and observational facilities.
更多查看译文
关键词
bayesian,reduced basis method (RBM),relativistic mean field (RMF) theory,nuclear physics,density functional theory
AI 理解论文
溯源树
样例
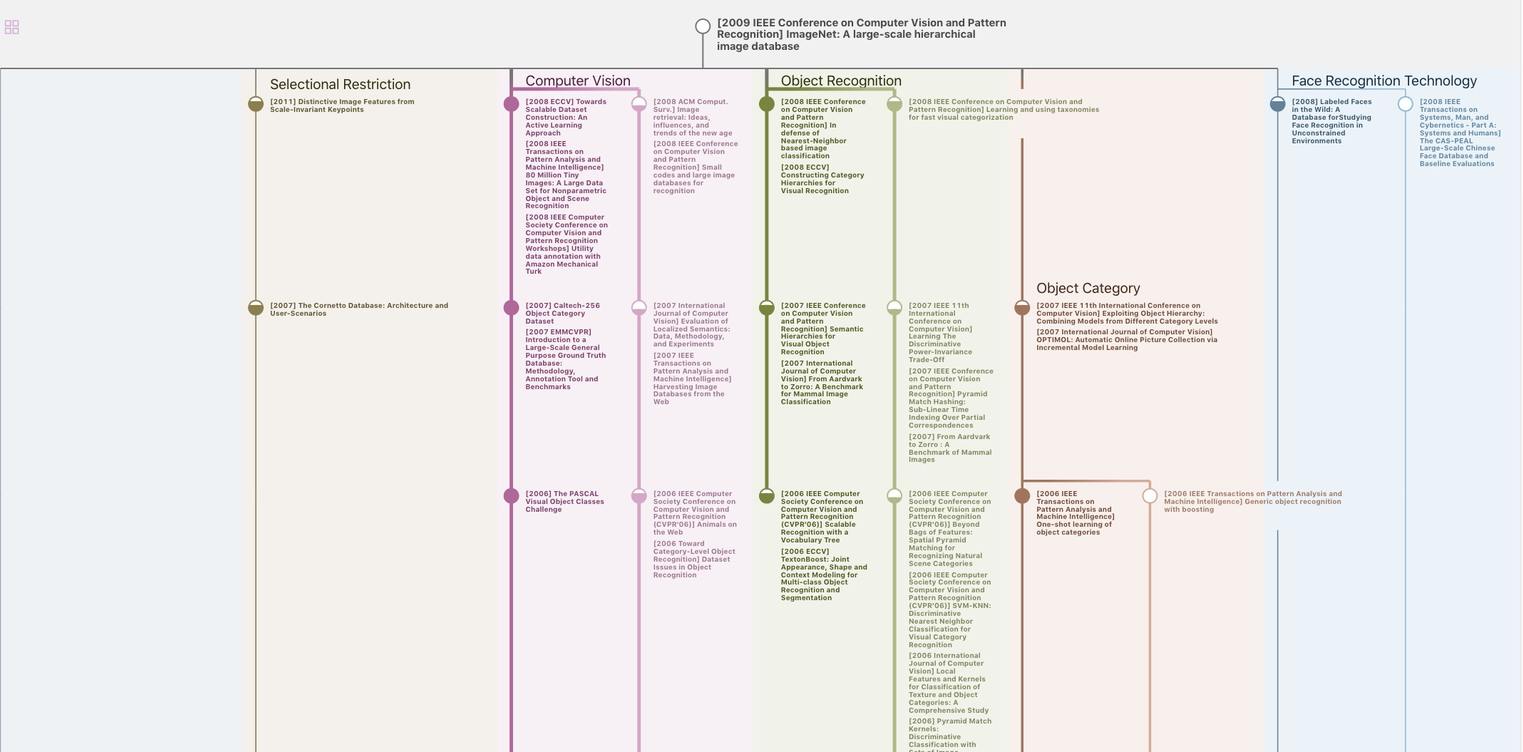
生成溯源树,研究论文发展脉络
Chat Paper
正在生成论文摘要