PearNet: A Pearson Correlation-based Graph Attention Network for Sleep Stage Recognition
2022 IEEE 9th International Conference on Data Science and Advanced Analytics (DSAA)(2022)
摘要
Sleep stage recognition is crucial for assessing sleep and diagnosing chronic diseases. Deep learning models, such as Convolutional Neural Networks and Recurrent Neural Networks, are trained using grid data as input, making them not capable of learning relationships in non-Euclidean spaces. Graph-based deep models have been developed to address this issue when investigating the external relationship of electrode signals across different brain regions. However, the models cannot solve problems related to the internal relationships between segments of electrode signals within a specific brain region. In this study, we propose a Pearson correlation-based graph attention network, called PearNet, as a solution to this problem. Graph nodes are generated based on the spatial-temporal features extracted by a hierarchical feature extraction method, and then the graph structure is learned adaptively to build node connections. Based on our experiments on the Sleep-EDF-20 and Sleep-EDF-78 datasets, PearNet performs better than the state-of-the-art baselines.
更多查看译文
关键词
Sleep stage recognition,EEG measurement,Feature representation,Graph attention neural network
AI 理解论文
溯源树
样例
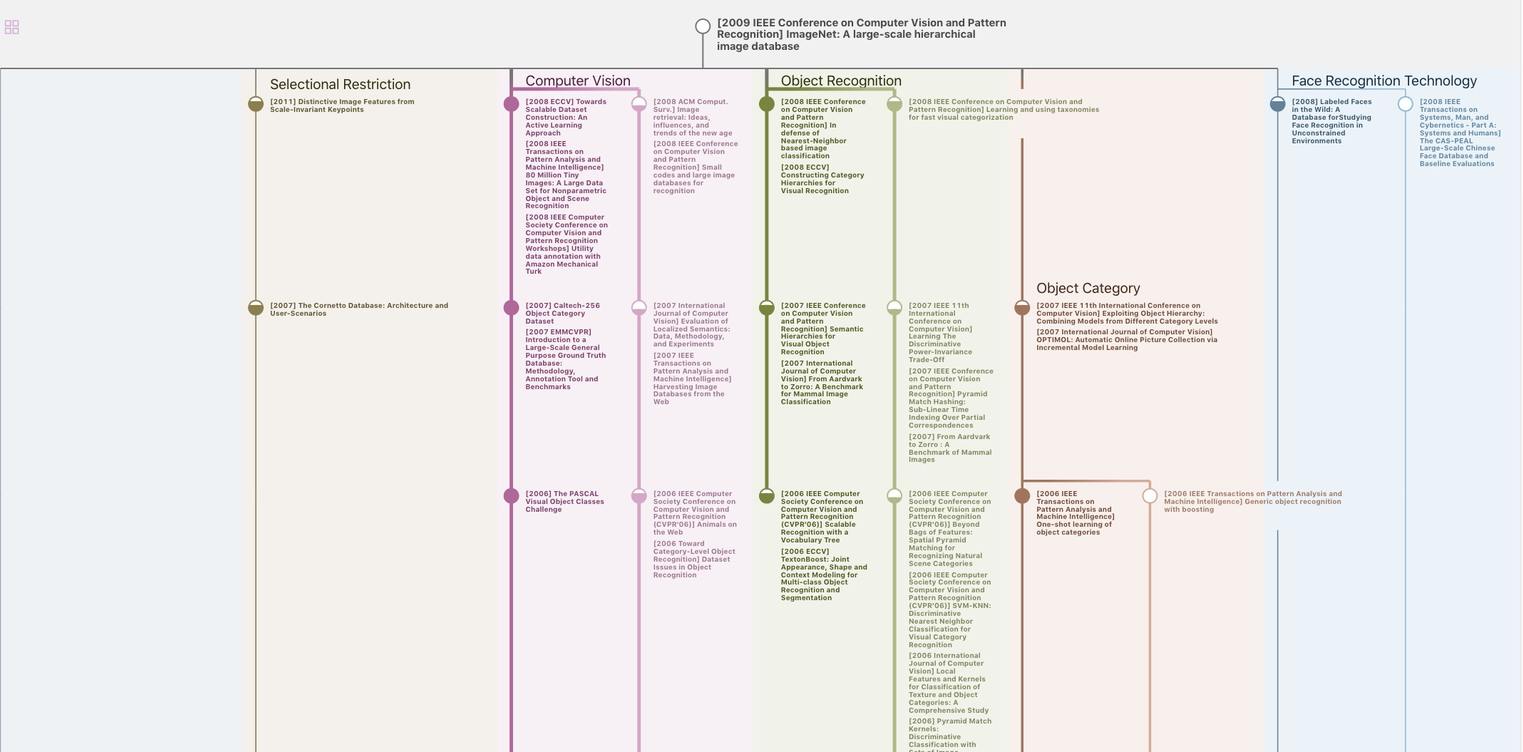
生成溯源树,研究论文发展脉络
Chat Paper
正在生成论文摘要