Data Augmentation using Feature Generation for Volumetric Medical Images
CoRR(2022)
摘要
Medical image classification is one of the most critical problems in the image recognition area. One of the major challenges in this field is the scarcity of labelled training data. Additionally, there is often class imbalance in datasets as some cases are very rare to happen. As a result, accuracy in classification task is normally low. Deep Learning models, in particular, show promising results on image segmentation and classification problems, but they require very large datasets for training. Therefore, there is a need to generate more of synthetic samples from the same distribution. Previous work has shown that feature generation is more efficient and leads to better performance than corresponding image generation. We apply this idea in the Medical Imaging domain. We use transfer learning to train a segmentation model for the small dataset for which gold-standard class annotations are available. We extracted the learnt features and use them to generate synthetic features conditioned on class labels, using Auxiliary Classifier GAN (ACGAN). We test the quality of the generated features in a downstream classification task for brain tumors according to their severity level. Experimental results show a promising result regarding the validity of these generated features and their overall contribution to balancing the data and improving the classification class-wise accuracy.
更多查看译文
关键词
volumetric medical images,medical images,feature generation
AI 理解论文
溯源树
样例
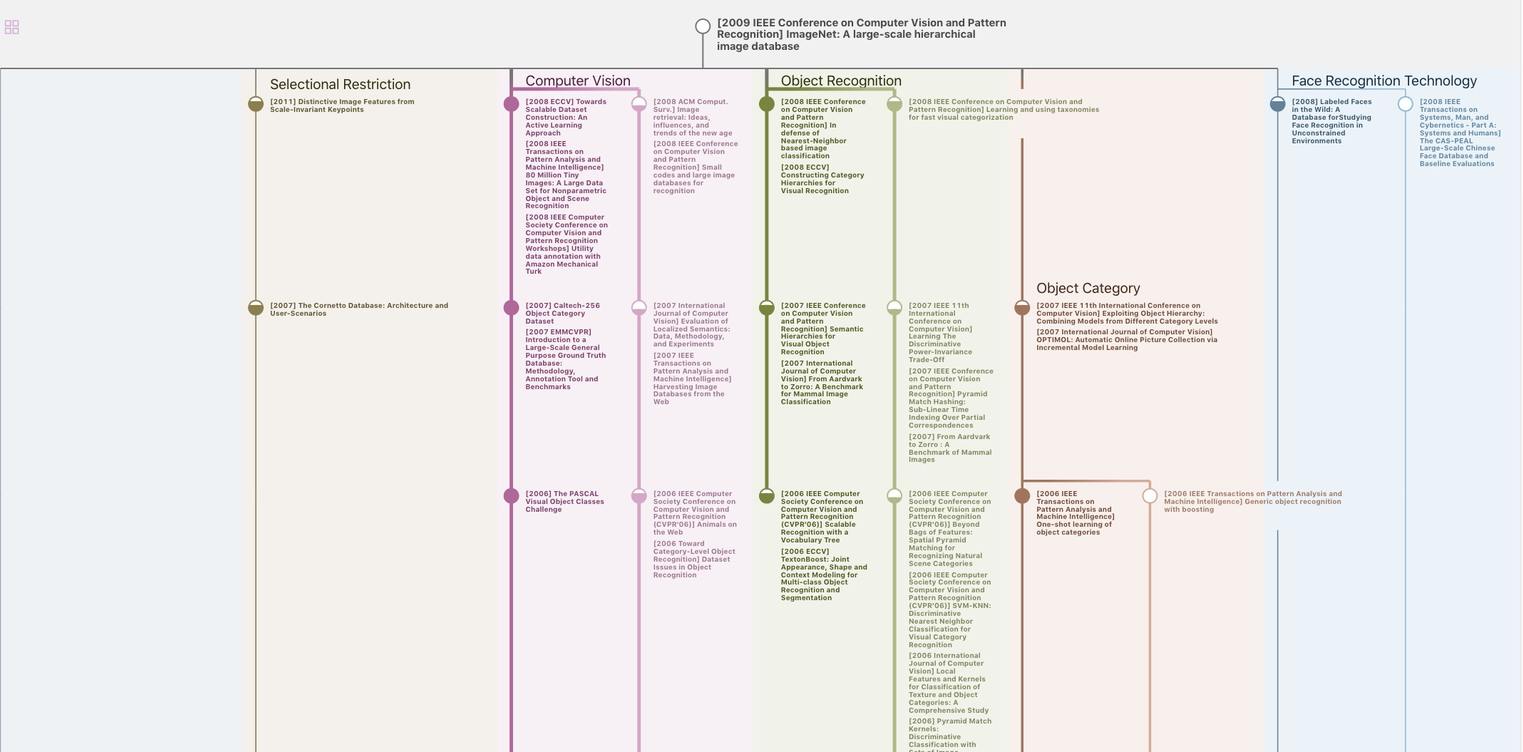
生成溯源树,研究论文发展脉络
Chat Paper
正在生成论文摘要