Zero-Shot Retargeting of Learned Quadruped Locomotion Policies Using Hybrid Kinodynamic Model Predictive Control
arXiv (Cornell University)(2022)
摘要
Reinforcement Learning (RL) has witnessed great strides for quadruped locomotion, with continued progress in the reliable sim-to-real transfer of policies. However, it remains a challenge to reuse a policy on another robot, which could save time for retraining. In this work, we present a framework for zero-shot policy retargeting wherein diverse motor skills can be transferred between robots of different shapes and sizes. The new framework centers on a planning-and-control pipeline that systematically integrates RL and Model Predictive Control (MPC). The planning stage employs RL to generate a dynamically plausible trajectory as well as the contact schedule, avoiding the combinatorial complexity of contact sequence optimization. This information is then used to seed the MPC to stabilize and robustify the policy roll-out via a new Hybrid Kinodynamic (HKD) model that implicitly optimizes the foothold locations. Hardware results show an ability to transfer policies from both the A1 and Laikago robots to the MIT Mini Cheetah robot without requiring any policy re-tuning.
更多查看译文
关键词
learned quadruped locomotion policies,zero-shot
AI 理解论文
溯源树
样例
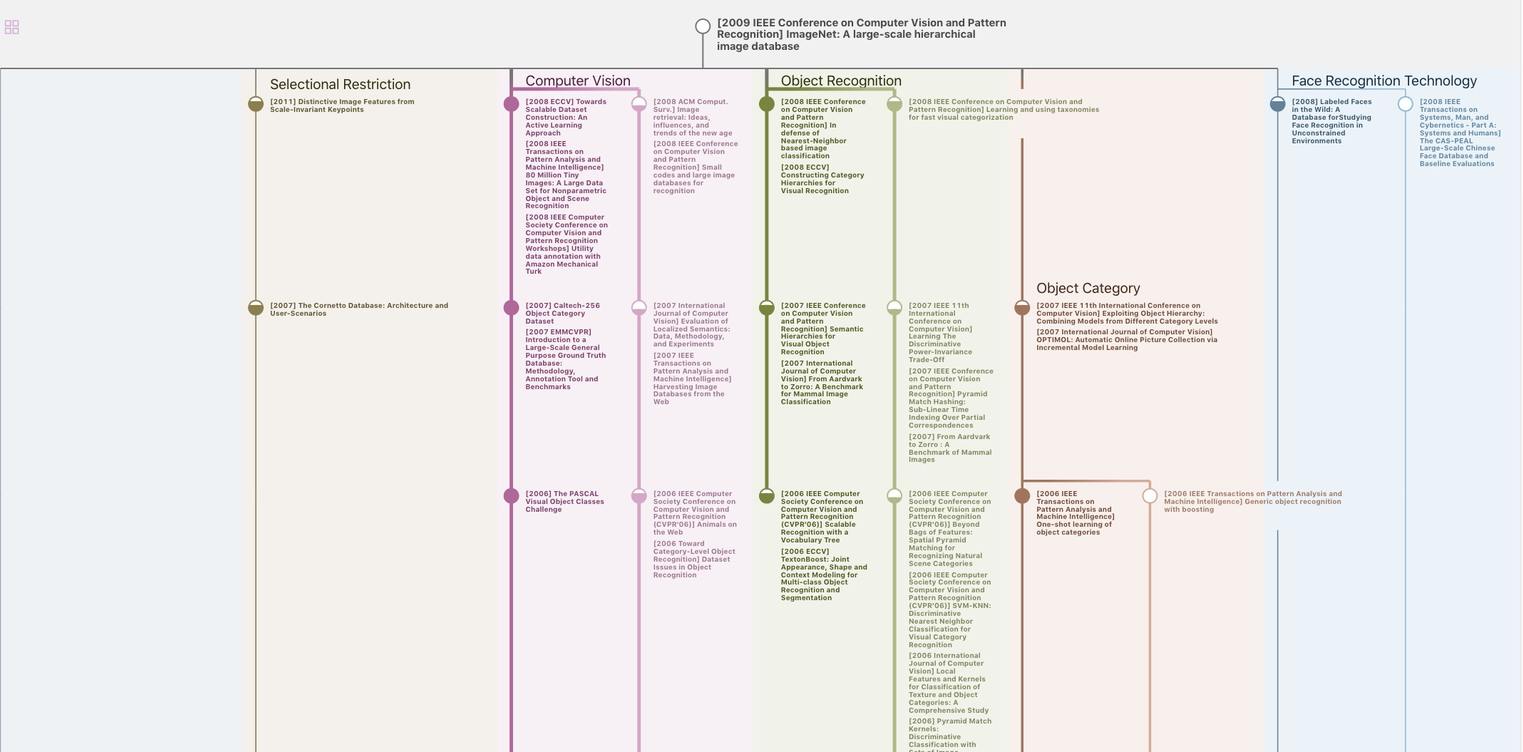
生成溯源树,研究论文发展脉络
Chat Paper
正在生成论文摘要