Shallow artificial networks with morphokinetic time‐lapse parameters coupled to ART data allow to predict live birth
Reproductive Medicine and Biology(2022)
摘要
Purpose The purpose of this work was to construct shallow neural networks (SNN) using time-lapse technology (TLT) from morphokinetic parameters coupled to assisted reproductive technology (ART) parameters in order to assist the choice of embryo(s) to be transferred with the highest probability of achieving a live birth (LB). Methods A retrospective observational single-center study was performed, 654 cycles were included. Three SNN: multilayers perceptron (MLP), simple recurrent neuronal network (simple RNN) and long short term memory RNN (LSTM-RNN) were trained with K-fold cross-validation to avoid sampling bias. The predictive power of SNNs was measured using performance scores as AUC (area under curve), accuracy, precision, Recall and F1 score. Results In the training data group, MLP and simple RNN provide the best performance scores; however, all AUCs were above 0.8. In the validating data group, all networks were equivalent with no performance scores difference and all AUC values were above 0.8. Conclusion Coupling morphokinetic parameters with ART parameters allows to SNNs to predict the probability of LB, and all SNNs seems to be efficient according to the performance scores. An automatic time recognition system coupled to one of these SNNs could allow a complete automation to choose the blastocyst(s) to be transferred.
更多查看译文
关键词
artificial intelligence, blastocyst, embryo selection, time lapse
AI 理解论文
溯源树
样例
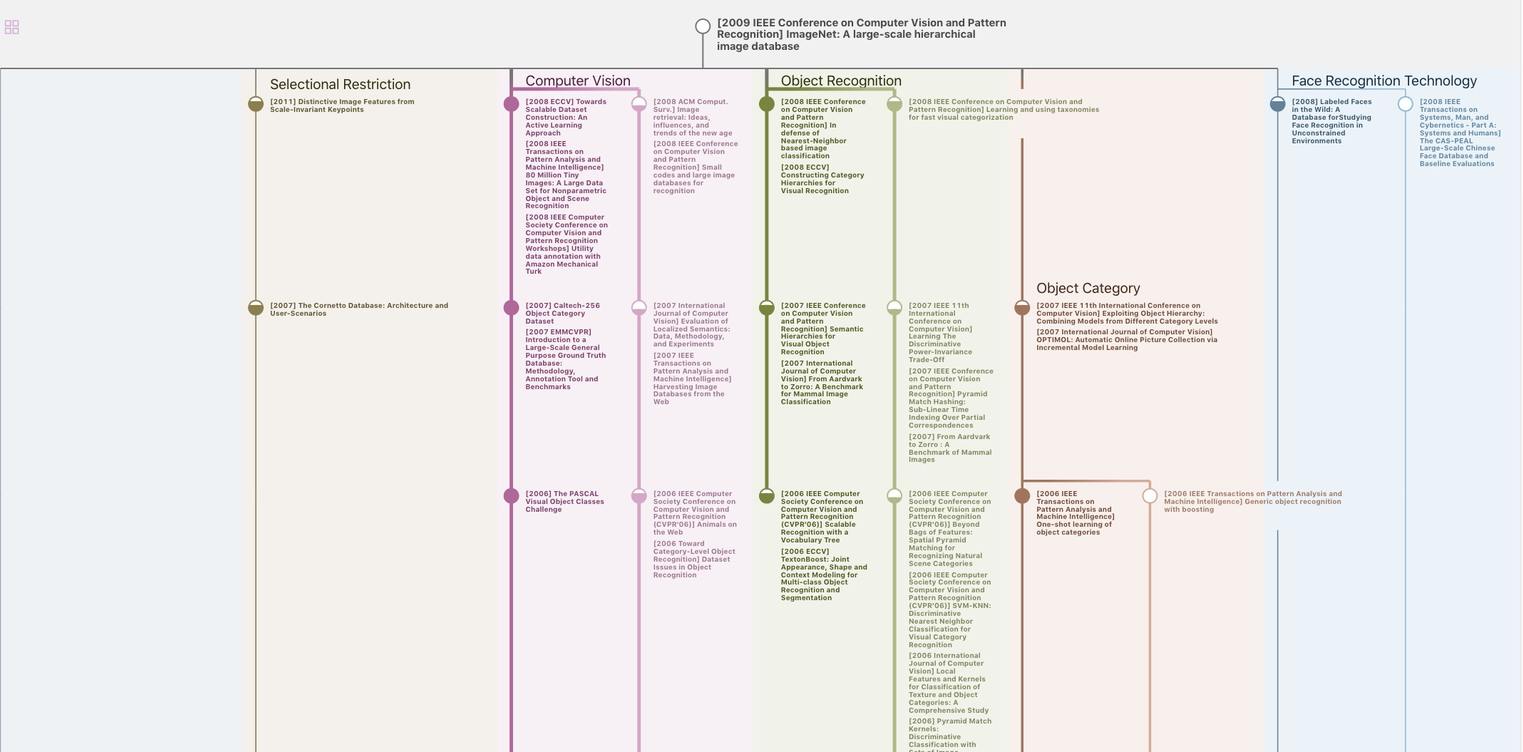
生成溯源树,研究论文发展脉络
Chat Paper
正在生成论文摘要