Deep Federated Learning Based Convergence Analysis in Relaying-Aided MEC-IoT Networks
Journal of Engineering(2022)
摘要
Recently, deep federated learning has attracted much attention from researchers in the fields of wireless communications, where the relaying technique has been shown as a powerful technology to assist the wireless signals and enhance the transmission quality, which is very important to the development of mobile edge computing (MEC) based Internet of Things (IoT) networks. In a relaying-aided MEC-IoT system, it is of vital importance to deeply investigate the system signal-to-noise ratio (SNR) at the receiver side, as it mainly determines the system performance metrics, such as capacity (or achievable data rate), outage probability, and bit-error-rate (BER). To this end, we first investigate the instantaneous convergence error, by deeply studying the relationship between the instantaneous two-hop relaying channels. We then investigate the statistical convergence error, by performing the statistical expectation with respect to the two-hop relaying channels. We finally present some results to show that the analysis of the convergence error is effective. The work in this paper can provide some theoretical foundation for deep federated learning and computing networks.
更多查看译文
关键词
networks,relaying-aided,mec-iot
AI 理解论文
溯源树
样例
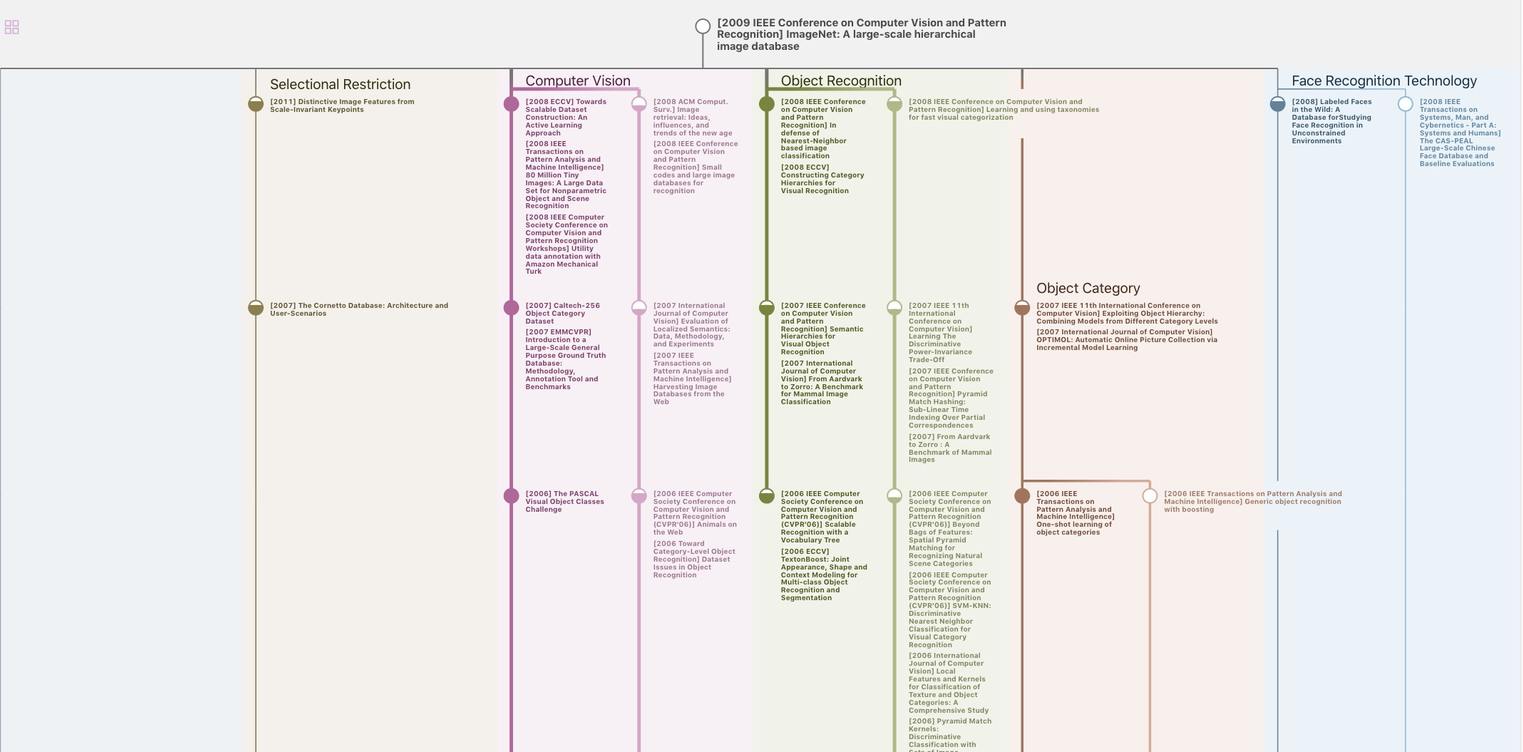
生成溯源树,研究论文发展脉络
Chat Paper
正在生成论文摘要