Automatic Detection of Outliers in Multi-Channel EMG Signals Using MFCC and SVM
Intelligent Automation & Soft Computing(2023)
摘要
The automatic detection of noisy channels in surface Electromyogram (sEMG) signals, at the time of recording, is very critical in making a noise-free EMG dataset. If an EMG signal contaminated by high-level noise is recorded, then it will be useless and can't be used for any healthcare application. In this research work, a new machine learning-based paradigm is proposed to automate the detection of low-level and high-level noises occurring in different channels of high density and multi-channel sEMG signals. A modified version of mel fre-quency cepstral coefficients (mMFCC) is proposed for the extraction of features from sEMG channels along with other statistical parameters i-e complexity coef-ficient, hurst exponent, and root mean square. Several state-of-the-art classifiers such as Support Vector Machine (SVM), Ensemble Bagged Trees, Ensemble Sub-space Discriminant, and Logistic Regression are used to automatically identify an EMG channel either bad or good based on these extracted features. Comparison -based analyses of these classifiers have also been considered based on total classi-fication accuracy, prediction speed (observations/sec), and processing time. The proposed method is tested on 320 simulated EMG channels as well as 640 experi-mental EMG channels. SVM is used as our main classifier for the detection of noisy channels which gives a total classification accuracy of 99.4% for simulated EMG channels whereas accuracy of 98.9% is achieved for experimental EMG channels.
更多查看译文
关键词
Machine learning,surface electromyography,support vector machine,classi fi cation,features
AI 理解论文
溯源树
样例
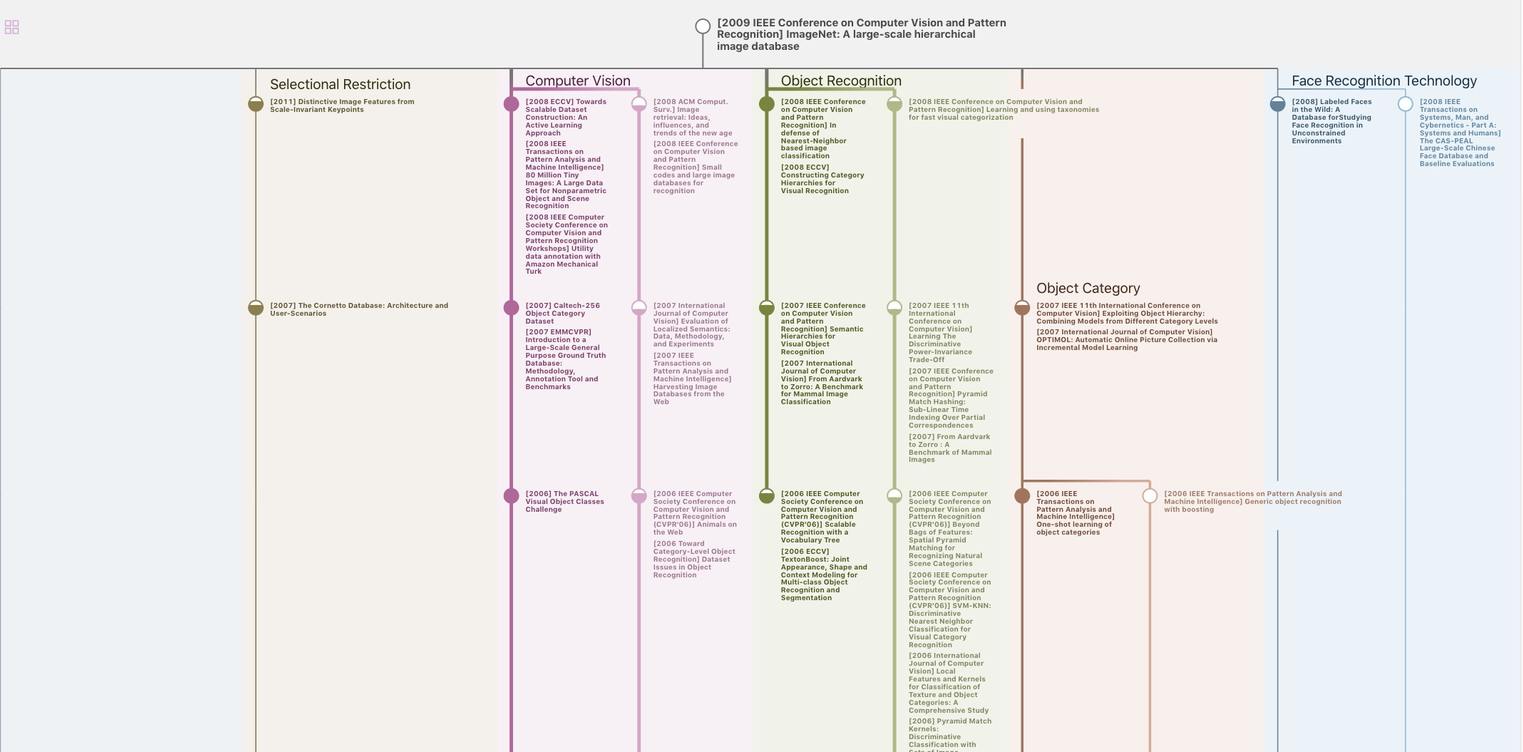
生成溯源树,研究论文发展脉络
Chat Paper
正在生成论文摘要