StarSum: A Star Architecture Based Model for Extractive Summarization
IEEE-ACM TRANSACTIONS ON AUDIO SPEECH AND LANGUAGE PROCESSING(2022)
摘要
Extractive summarization aims to produce a concise summary while retaining the key information through the way of selecting sentences from the original document. Under such background, learning inter-sentence relations has hitherto been the issue of most concern. In this study, we propose a Star architecture based model for extractive summarization (StarSum), that takes advantage of self-attention strategy based Transformer and star-shaped structure, models sentences within a document as satellite nodes and introduces a virtual star node, constructs a star model for each document to learn inter-sentence relations. Based on the constructed star-shaped model, we further develop two sentence representation learning algorithms, namely star guiding satellite (SGS) algorithm and star incorporating satellite (SIS) algorithm, in order to extract summary-worthy sentences. Experimental results on CNN/Daily Mail, New York Times (NYT) and XSum datasets prove that StarSum model achieves advanced performance for extractive summarization and has comparable performance to the state-of-the-art extractive summarization model. The results also demonstrate that the SIS algorithm is more effective than the SGS algorithm.
更多查看译文
关键词
Stars,Satellites,Data mining,Feature extraction,Transformers,Recurrent neural networks,Semantics,Extractive summarization,star architecture,inter-sentence relations,sentence representation learning
AI 理解论文
溯源树
样例
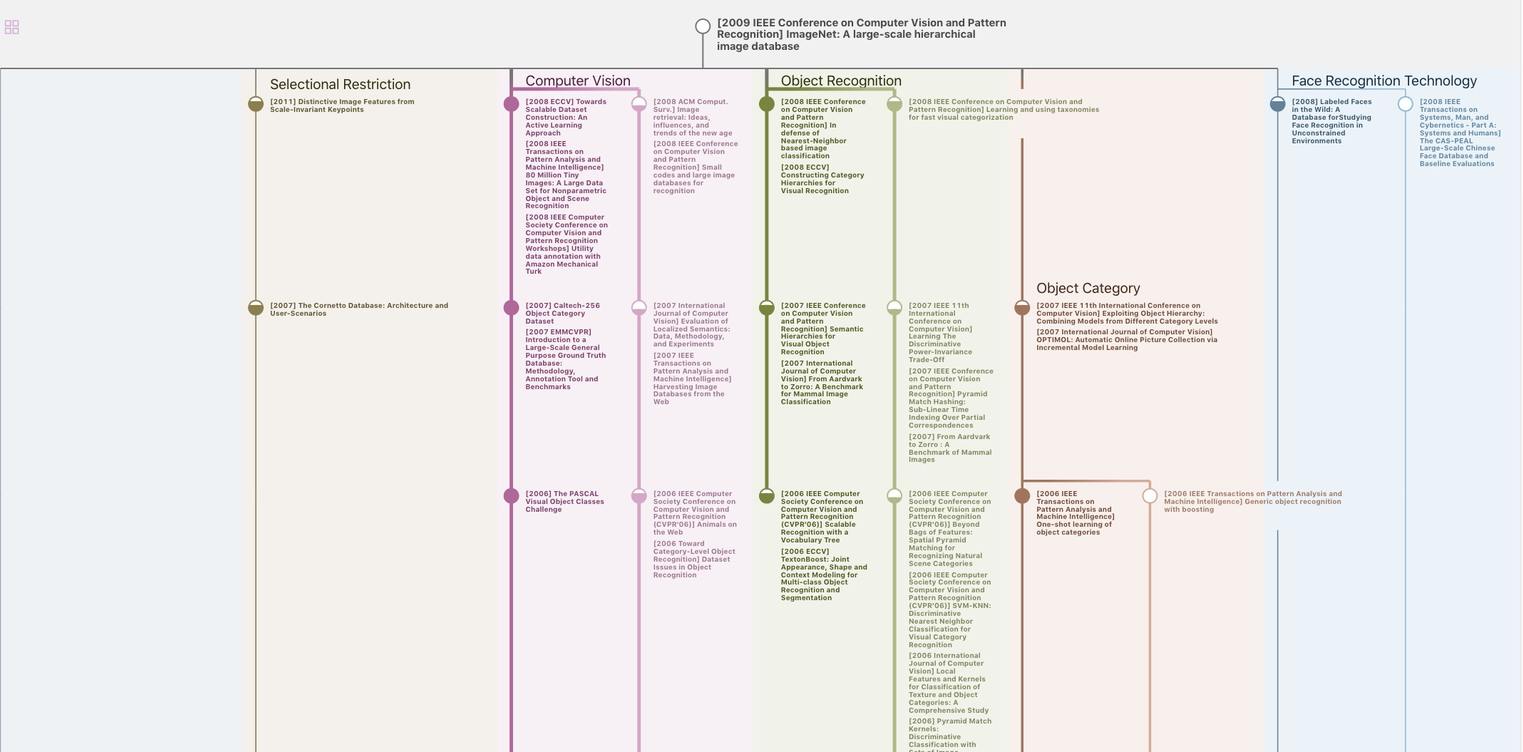
生成溯源树,研究论文发展脉络
Chat Paper
正在生成论文摘要