QoS-Aware Diversified Service Selection
IEEE Transactions on Services Computing(2023)
摘要
In QoS-aware service selection, merely considering the prediction accuracy of QoS is prone to redundant results, which hinders practical service composition and also undermines user's preference for rich service attributes. To address the problem, a novel diversity-aware graph-based QoS prediction model-DSSN (Diversified Service Selection Network) is proposed in this work. DSSN alleviates selection redundancy through enhancing the selection diversity besides QoS prediction accuracy. To improve the model performance, techniques like high-order message propagation and multi-task structure are integrated into the graph based neural network model. And to enhance the service diversity, a service distance based attention mechanism is designed to embed the users in the model, so that users are connected to services with diverse attributes. We evaluate DSSN on a public dataset via extensive comparison experiments with both diversity-aware and non-diversified service selection models. In the comparison experiments, the DSSN: 1) clearly outperforms the state-of-the-art diversity-aware models in both accuracy and diversity; 2) achieves concrete diversity improvement at the cost of an acceptable decrease in the QoS prediction compared to non-diversified baselines. The results demonstrate that DSSN is more suitable for diversity-aware service selection with ambiguous user requirements than traditional QoS-centric selection scenarios.
更多查看译文
关键词
Graph convolutional network,multi-task learning,service diversity,service selection,quality of service
AI 理解论文
溯源树
样例
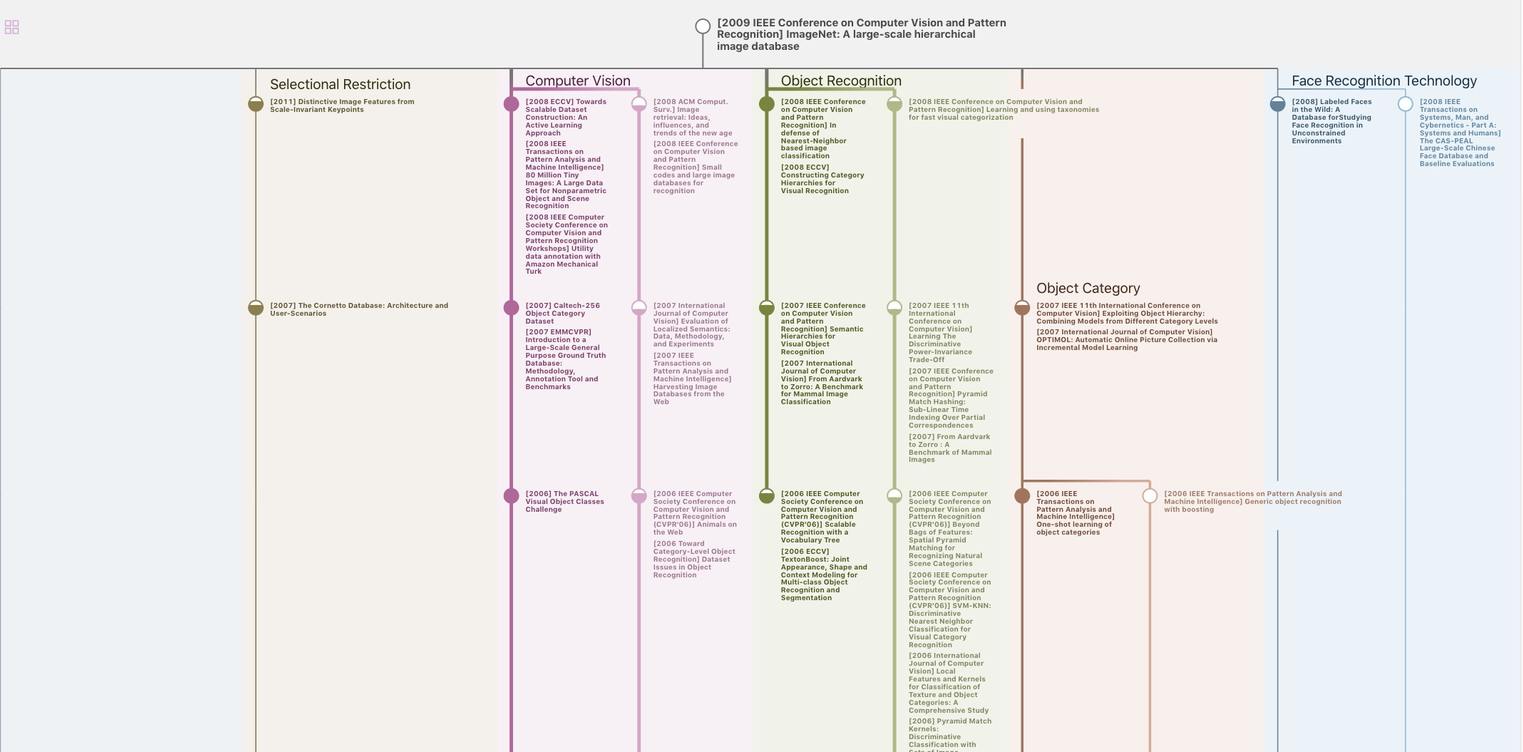
生成溯源树,研究论文发展脉络
Chat Paper
正在生成论文摘要