Two-Stage Sparse Representation Clustering for Dynamic Data Streams.
IEEE transactions on cybernetics(2022)
摘要
Data streams are a potentially unbounded sequence of data objects, and the clustering of such data is an effective way of identifying their underlying patterns. Existing data stream clustering algorithms face two critical issues: 1) evaluating the relationship among data objects with individual landmark windows of fixed size and 2) passing useful knowledge from previous landmark windows to the current landmark window. Based on sparse representation techniques, this article proposes a two-stage sparse representation clustering (TSSRC) method. The novelty of the proposed TSSRC algorithm comes from evaluating the effective relationship among data objects in the landmark windows with an accurate number of clusters. First, the proposed algorithm evaluates the relationship among data objects using sparse representation techniques. The dictionary and sparse representations are iteratively updated by solving a convex optimization problem. Second, the proposed TSSRC algorithm presents a dictionary initialization strategy that seeks representative data objects by making full use of the sparse representation results. This efficiently passes previously learned knowledge to the current landmark window over time. Moreover, the convergence and sparse stability of TSSRC can be theoretically guaranteed in continuous landmark windows under certain conditions. Experimental results on benchmark datasets demonstrate the effectiveness and robustness of TSSRC.
更多查看译文
关键词
Clustering algorithms,Dictionaries,Heuristic algorithms,Machine learning,Streaming media,Data models,Convergence,Clustering,data stream,dictionary learning,sparse representation
AI 理解论文
溯源树
样例
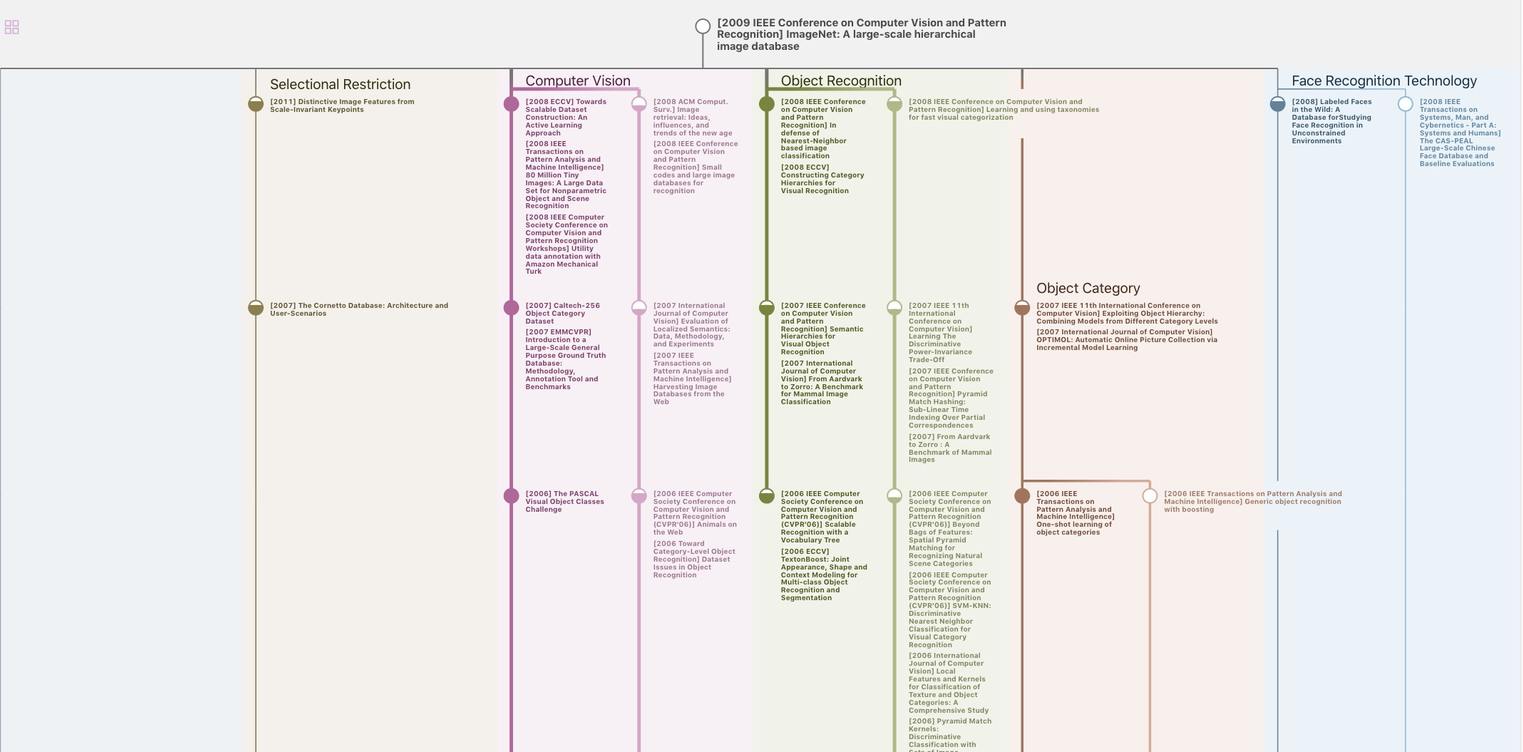
生成溯源树,研究论文发展脉络
Chat Paper
正在生成论文摘要