Automatic interpretation and clinical evaluation for fundus fluorescein angiography images of diabetic retinopathy patients by deep learning
BRITISH JOURNAL OF OPHTHALMOLOGY(2023)
Abstract
Background/aims Fundus fluorescein angiography (FFA) is an important technique to evaluate diabetic retinopathy (DR) and other retinal diseases. The interpretation of FFA images is complex and time-consuming, and the ability of diagnosis is uneven among different ophthalmologists. The aim of the study is to develop a clinically usable multilevel classification deep learning model for FFA images, including prediagnosis assessment and lesion classification. Methods A total of 15 599 FFA images of 1558 eyes from 845 patients diagnosed with DR were collected and annotated. Three convolutional neural network (CNN) models were trained to generate the label of image quality, location, laterality of eye, phase and five lesions. Performance of the models was evaluated by accuracy, F-1 score, the area under the curve and human-machine comparison. The images with false positive and false negative results were analysed in detail. Results Compared with LeNet-5 and VGG16, ResNet18 got the best result, achieving an accuracy of 80.79%-93.34% for prediagnosis assessment and an accuracy of 63.67%-88.88% for lesion detection. The human-machine comparison showed that the CNN had similar accuracy with junior ophthalmologists. The false positive and false negative analysis indicated a direction of improvement. Conclusion This is the first study to do automated standardised labelling on FFA images. Our model is able to be applied in clinical practice, and will make great contributions to the development of intelligent diagnosis of FFA images.
MoreTranslated text
Key words
Imaging,Telemedicine,Retina
AI Read Science
Must-Reading Tree
Example
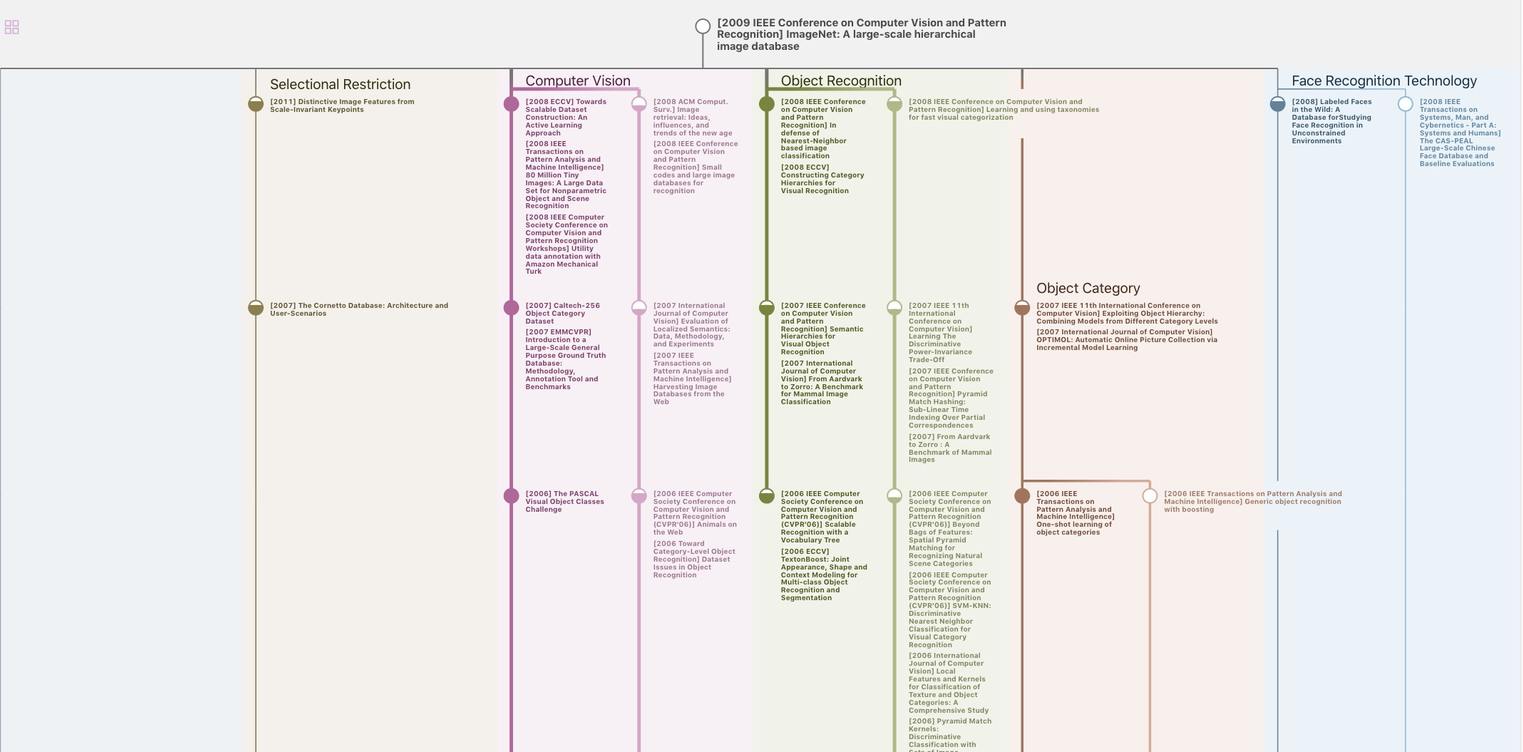
Generate MRT to find the research sequence of this paper
Chat Paper
Summary is being generated by the instructions you defined