Lightweight Model Uncertainty Estimation for Deep Neural Object Detection
IEEE International Joint Conference on Neural Network (IJCNN)(2022)
摘要
Quantifying model uncertainty of Deep Neural Network (DNN) is important to understand the reliability of the model prediction and avoid risks in safety critical applications. Various approaches, including Bayesian neural networks, Monte-Carlo dropout, and ensembles, are suggested to measure the model uncertainty; but with huge computational cost. We present ModelNet, an Artificial Neural Network (ANN) that can estimate spatial/semantic model uncertainties of a DNN based object detection with less computation overhead. ModelNet is a deterministic ANN that distills the predictive distribution of stochastic DNN. Experimental results show that ModelNet can learn the uncertainty estimation from stochastic DNN in various architectures. ModelNet can perform as a probabilistic object detector with 39x-179x less number of operations, or as an uncertainty assistant to a task network with 1.4x more parameters and 38x less number of operations compared to stochastic DNN. Moreover, a case study of uncertainty driven adaptive sensor using ModelNet is presented.
更多查看译文
关键词
deep neural object detection,uncertainty,model
AI 理解论文
溯源树
样例
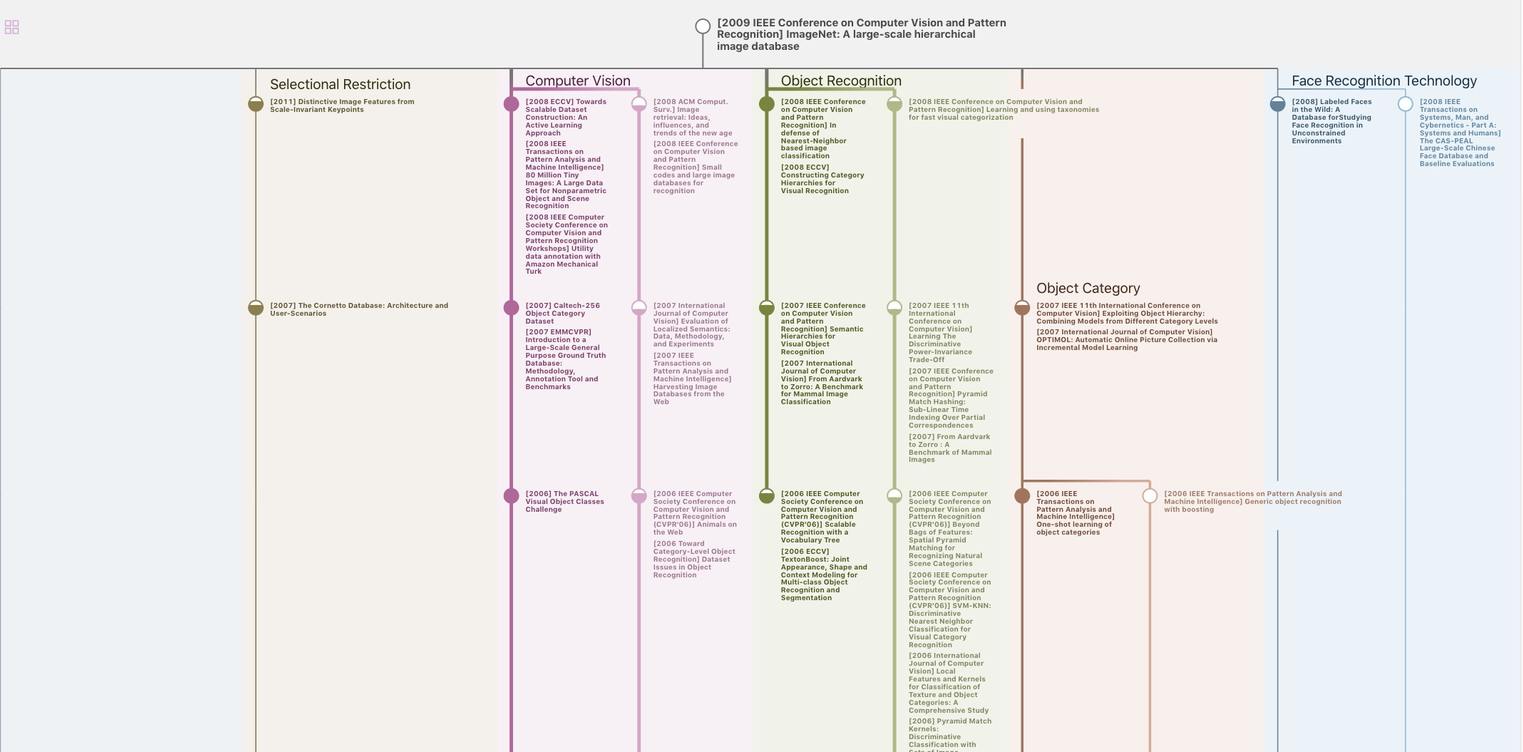
生成溯源树,研究论文发展脉络
Chat Paper
正在生成论文摘要