@ME: A Fine-grained Route Recommendation System to Grab Impatient Passengers
IEEE International Joint Conference on Neural Network (IJCNN)(2022)
摘要
Data analysis reveals that passengers can only endure a few minutes before taking a taxi. However, most existing route recommendation systems are not adequate to satisfy impatient customers due to two shortcomings: inaccurate demand forecast and the lack of an efficient supply-demand balance mechanism. In this paper, we propose a recommendation system called @Me, which aims to dispatch vacant taxis to the vicinity of potential customers at the right time. To achieve minute-level demand forecasting, we ensemble a contextualized spatial-temporal network (CSTN) with an LSTM network to optimize prediction accuracy. In addition, we characterize the attractiveness of the road grid to vacant taxis as a force model, on which a taxi scheduling algorithm is proposed to dynamically balance supply and demand. Extensive experiments on real datasets clearly indicate that our method is superior to the selected baselines. Vacant taxis that follow the routes suggested by @ME can catch more impatient customers in a shorter cruising time. The 7-day experimental results on the Manhattan dataset show that @Me can carry an additional 48,332 passengers and increase drivers' revenue by $570,317.
更多查看译文
关键词
route recommendation system,impatient passengers,data analysis,existing route recommendation systems,impatient customers,inaccurate demand forecast,efficient supply-demand balance mechanism,vacant taxis,minute-level demand forecasting,spatial-temporal network,LSTM network,taxi scheduling algorithm,additional 48 passengers,332 passengers
AI 理解论文
溯源树
样例
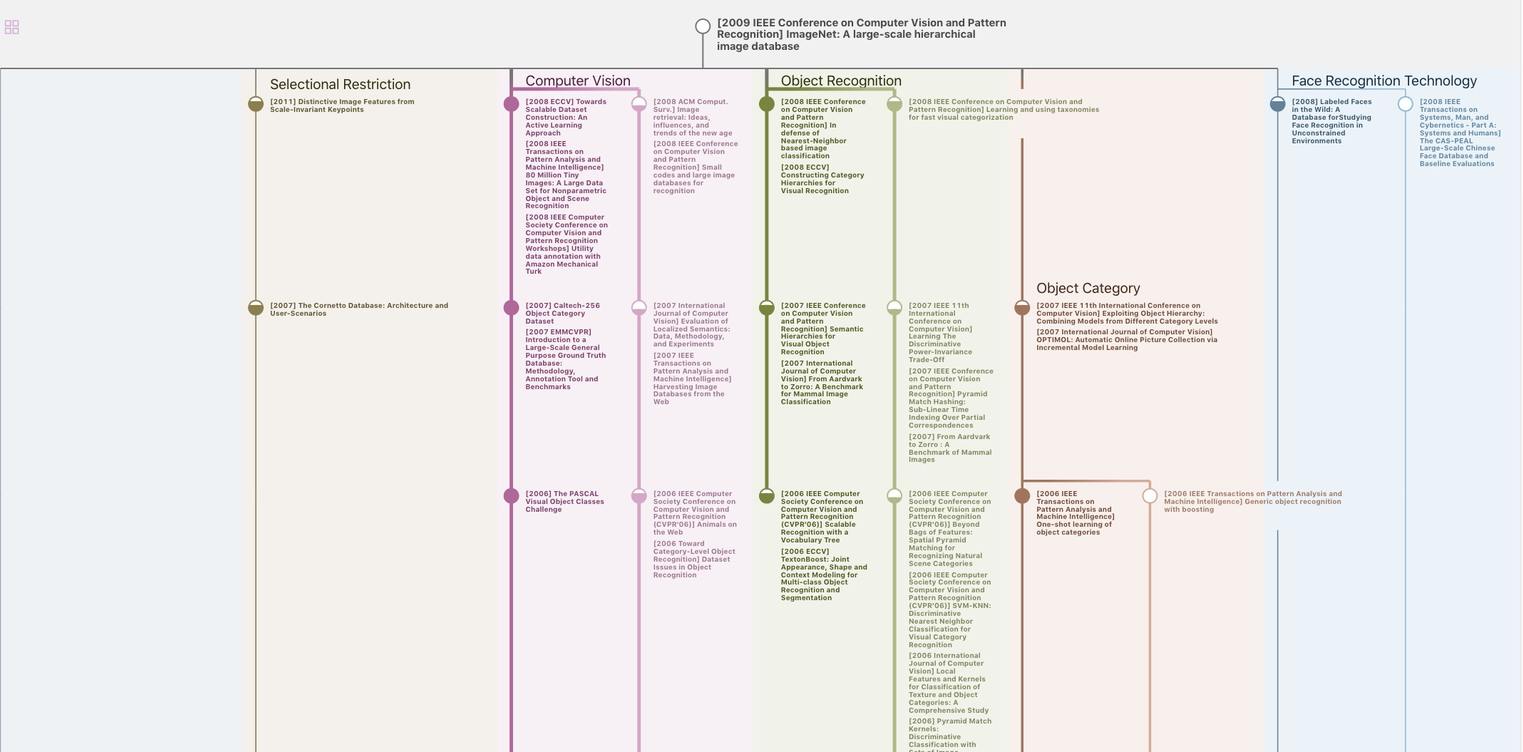
生成溯源树,研究论文发展脉络
Chat Paper
正在生成论文摘要