Multi-enhanced Adaptive Attention Network for RGB- T Salient Object Detection
IEEE International Joint Conference on Neural Network (IJCNN)(2022)
摘要
Nowadays, Salient object detection (SOD) on RGB images has achieved remarkable success. However, the performance of this single-modal SOD will be considerably reduced when faced with complicated situations. To deal with these challenges, the fusion of RGB and thermal infrared images, termed as RGB-T SOD, becomes a new SOD research direction recently. Thermal images can supply the essential additional information to RGB because they are immune to illumination and weather conditions. Though in this area, existing methods don't take full advantage of multi-level encoded features to generate global context. In addition, these approaches feed unprocessed encoded features that contain interference such as background directly to the decoder and don't explicitly establish the correlation of two heterogeneous modalities. In this paper, we proposed a multi-enhanced adaptive attention network (MEAANet) to solve aforementioned problems. Specifically, we use a multi-modal multi-level feature fusion (MMFF) module to fuse low-level and high-level encoded features to enhance the global context. Then, we design the thermal adaptive attention module (TAAM) to enhance encoded features while reducing noise interference. Moreover, to explore the correlation between the two modalities, we utilize the cross-enhanced integration module (CIM) to learn the shared features of two modalities. The comprehensive experimental results demonstrate the effectiveness of our proposed approach against the state-of-the-art.
更多查看译文
关键词
Salient object detection,RGB-T,encode feature fusion,thermal adaptive attention module,shared features
AI 理解论文
溯源树
样例
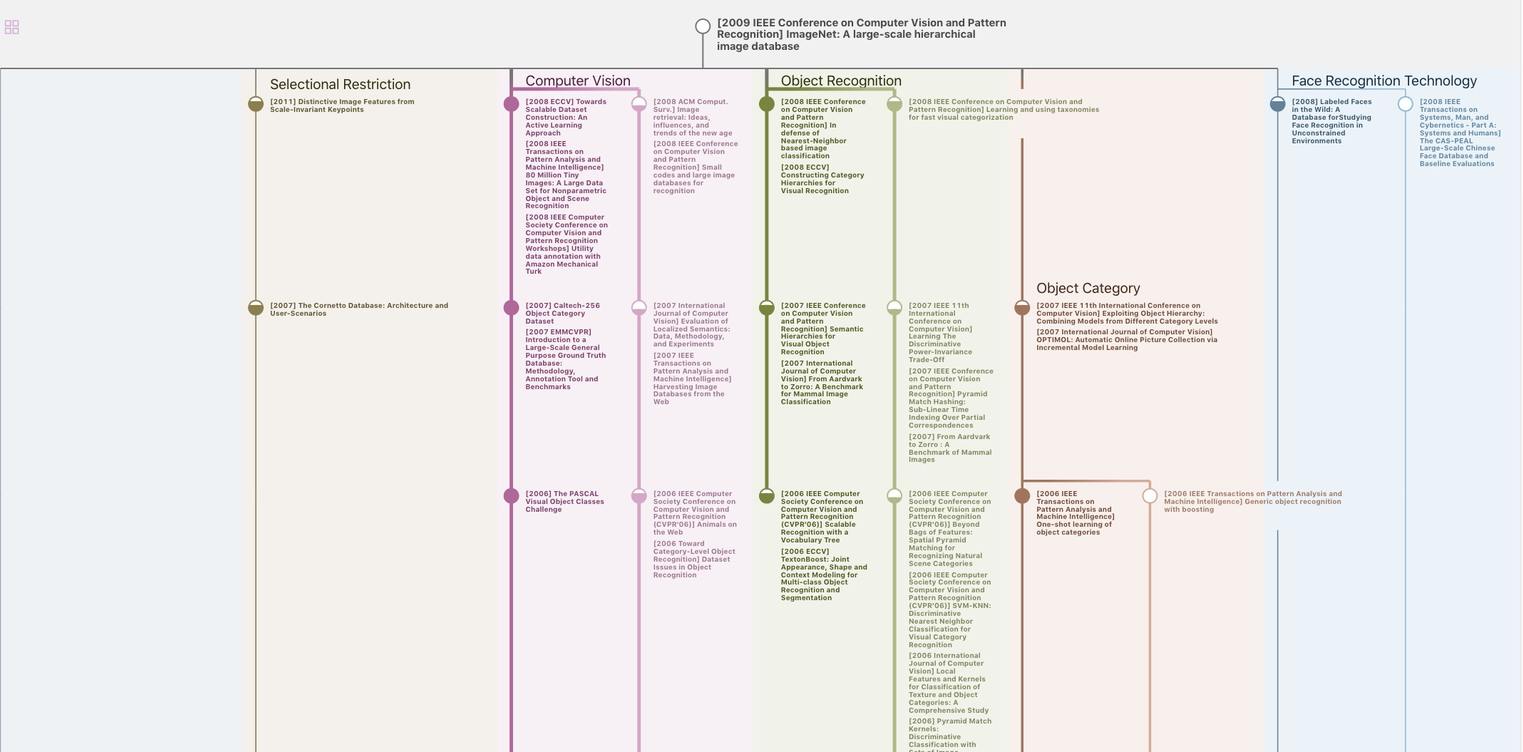
生成溯源树,研究论文发展脉络
Chat Paper
正在生成论文摘要