Localized Feature Ranking approach for Multi-Target Regression
IEEE International Joint Conference on Neural Network (IJCNN)(2022)
摘要
Multi-target regression (MTR) aims at designing models able to predict multiple continuous variables simultaneously. The key for designing an effective feature selection model for MTR is to develop a framework under which the feature importances are measured using the correlation between features and targets in a natural way. So far, feature importances in MTR problems were evaluated in a global sense where proposed approaches generate a single ordered list of features common for all the targets. In this work, we adapt the Ensemble of Regressor Chains algorithm in tandem with the random forest paradigm to appropriately model both dependencies among features and targets in a target-specific (localized) feature ranking process. We provide empirical results on several benchmark MTR data sets indicating the effectiveness of our strategy to perform better than selecting one global ranking for all targets with existing state-of-the-art algorithms.
更多查看译文
关键词
Multi-Target Regression,Feature Importance,Local variable selection
AI 理解论文
溯源树
样例
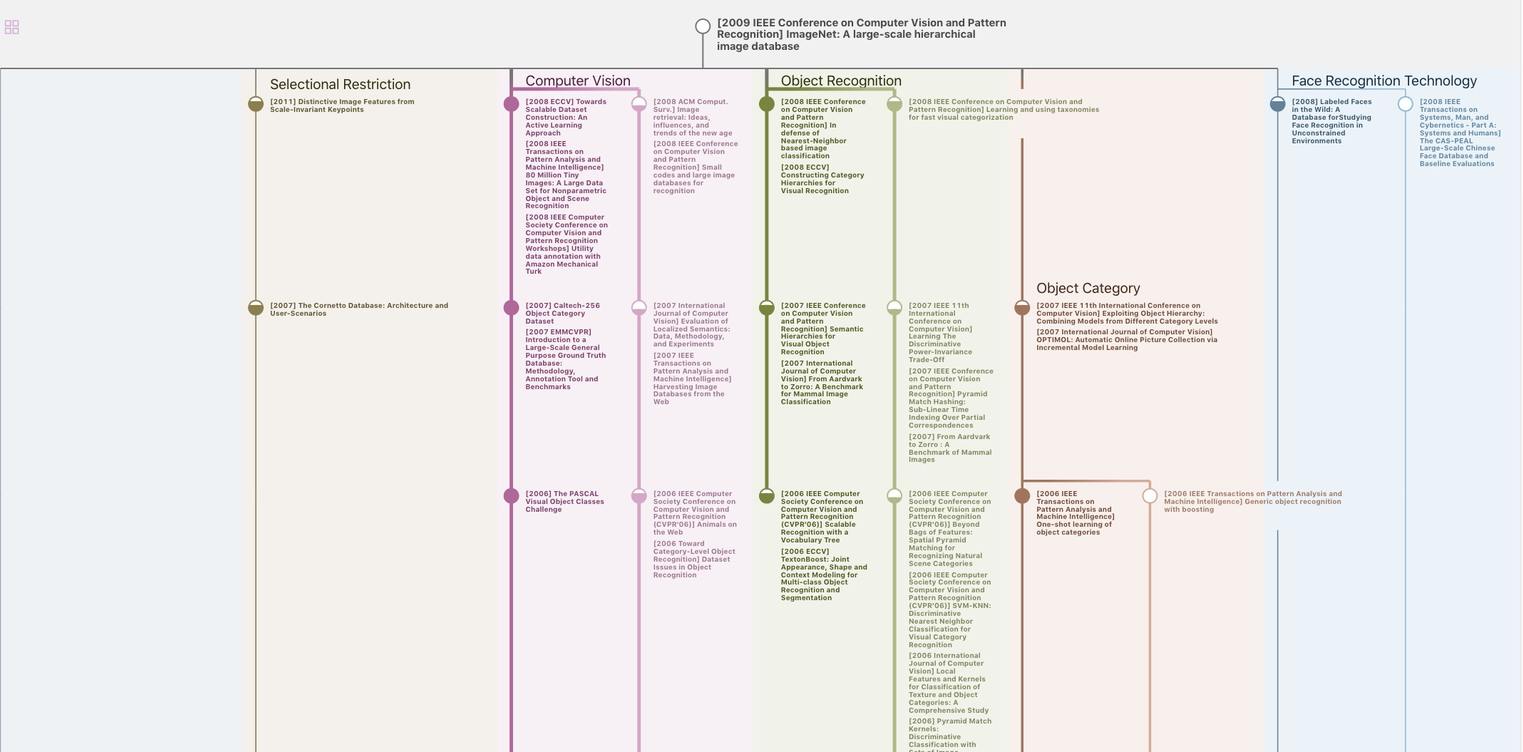
生成溯源树,研究论文发展脉络
Chat Paper
正在生成论文摘要