Stage Evolving Graph Neural Network based Dynamic Recommendation with Life Cycles
IEEE International Joint Conference on Neural Network (IJCNN)(2022)
摘要
Existing methods of dynamic recommender systems usually build neural networks or collaborative filtering models according to the absolute timeline, which fails to consider the preferences of users or characteristics of products in their life cycles. With the rise of graph neural networks, researchers have recently proposed methods based on graph neural networks to solve the recommendation problem. However, such models do not consider evolving stages in their life cycles. In this paper, we study the dynamic recommendation problem and propose a novel model named Stage Evolving Graph Neural Network (SEGNN). SEGNN learns the life stage representation by the Gated Stage Attention Unit (GSAU) and then uses a fully connected layer as the rating score predictor. GSAU adopts two types of attention layers to learn the preferences of users and products within one coupled life stage and discover the preferences over life stages, respectively. GSAU also utilizes a memory mechanism to ensure that the evolutionary patterns are maintained in the final representations of users and products. Due to the lack of information caused by data sparsity, SEGNN designs a masked loss function to train the model. Extensive experiments are done on real-world datasets, and the experimental results show the effectiveness of our proposed model.
更多查看译文
关键词
Stage Evolving Graph Neural Network,life cycles,dynamic recommender systems,collaborative filtering models,graph neural networks,dynamic recommendation problem,life stage representation,Gated Stage Attention Unit,GSAU,coupled life stage,life stages
AI 理解论文
溯源树
样例
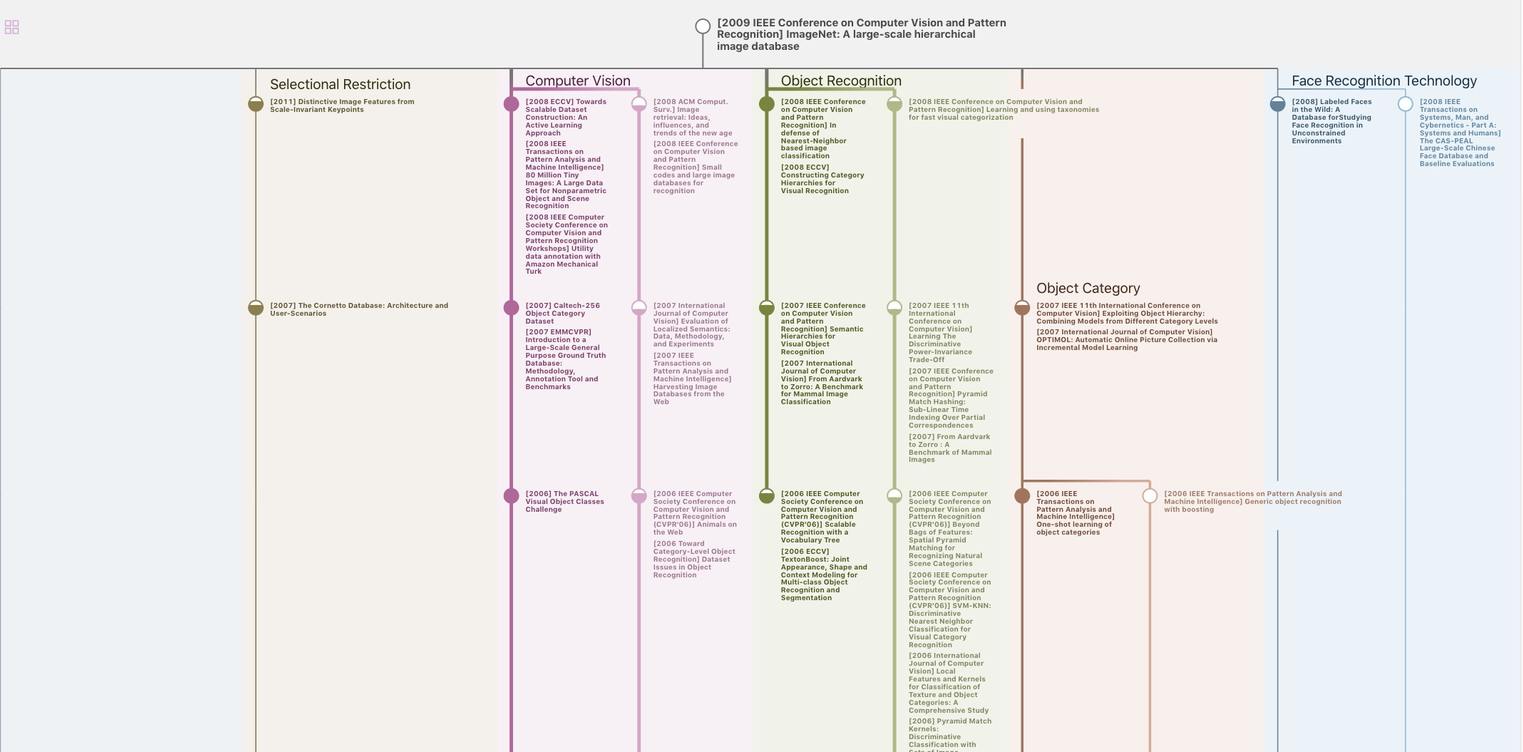
生成溯源树,研究论文发展脉络
Chat Paper
正在生成论文摘要