Dynamic Cox-Regression for Motif Prediction in Co-Evolving Time Series Data
IEEE International Joint Conference on Neural Network (IJCNN)(2022)
摘要
We investigate issues related to predicting motifs (i.e., frequently occurring patterns) in co-evolving time series. Unlike periodical series where motifs are contiguously aligned, in several applications, co-evolving series tend to exhibit motifs that are non-contiguously aligned. Capturing the mechanism of motif shifts in such evolving series poses significant challenges in predicting their respective subsequent appearances. This prompted us to devise a principled approach capable of handling series with non-contiguous motifs. In summary, we propose a sliding window analysis from which we devise a motif map that reflects the previous time-dependent mechanism of motif shifts occurring in a data set that comprises multiple time series. Based on the motif map, we model the motif transition mechanism via a dynamic Cox model and Support Vector Regression, which allows the prediction of series values at subsequent times. The originality of our approach lies in its modeling of time-dependent motif transition probabilities that have usually been assumed to be static in most of the existing work. Experiments on both synthetic and real data sets illustrate the suitability of our approach.
更多查看译文
关键词
Co-evolving time series data,Motif transition,Survival analysis,Evolving networks
AI 理解论文
溯源树
样例
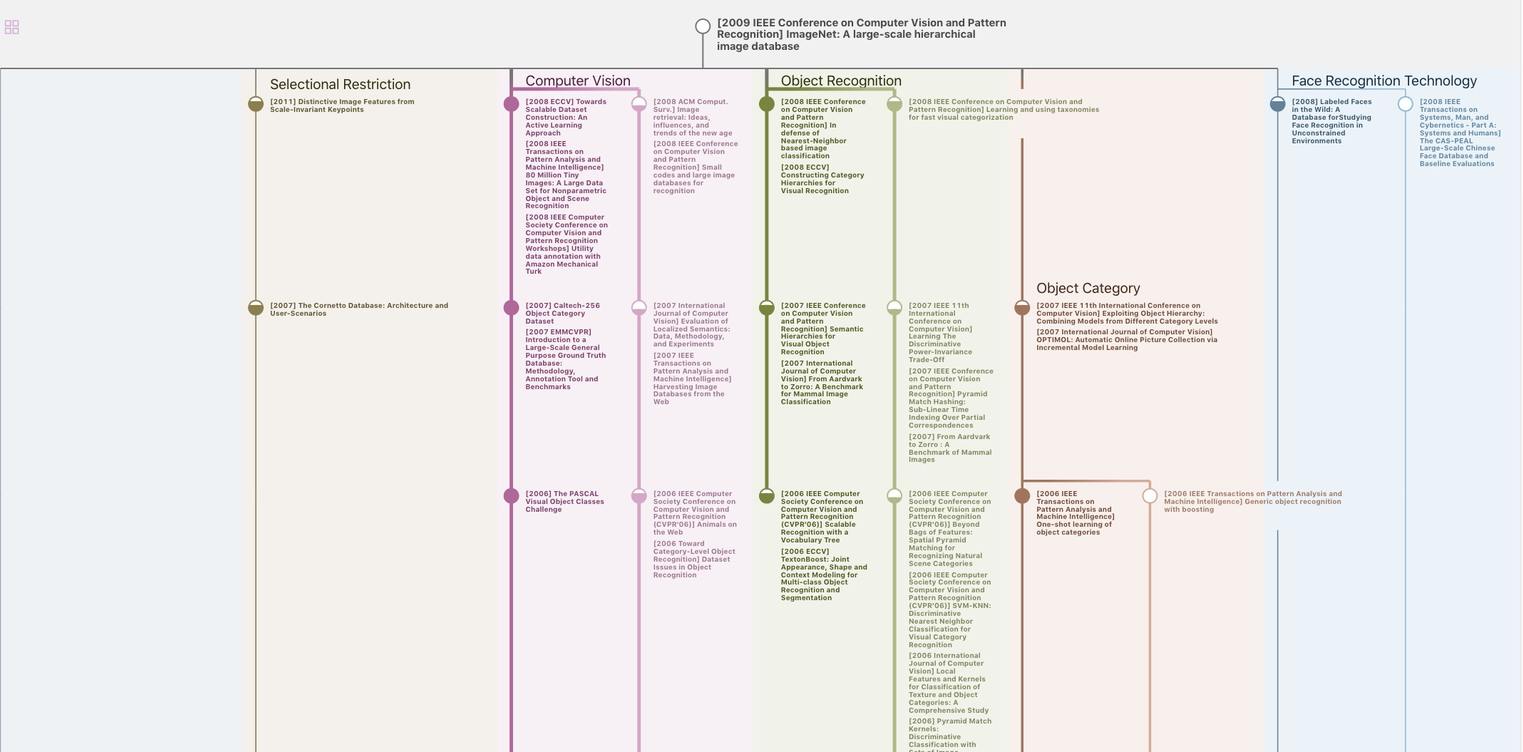
生成溯源树,研究论文发展脉络
Chat Paper
正在生成论文摘要