Tensor Neural Controlled Differential Equations
IEEE International Joint Conference on Neural Network (IJCNN)(2022)
摘要
In the recent decade, multidimensional or tensorial time series have drawn increasing attention for their rich spatial and temporal information. In data collection, missing tensorial time instances can always occur at any random time steps, which cause the time steps to be not equally spaced, i.e., irregular. Furthermore, it is very likely that the tensorial time series are influenced by other tensor factors, yet from external resources. These driving tensor factors are referred to as tensor controls, whereas the influenced tensorial time series are named as tensor responses. Existing methods either cannot predict tensor responses using all available past tensor controls, or are not able to directly model continuous-time tensor-valued processes, incurring the curse-of-dimensionality issue. Therefore, we propose tensor neural controlled differential equations (TENCDEs) which incorporate tensor controls and explicitly define the continuous-time tensor-valued processes for tensor controls and tensor responses aiming at resolving irregular tensorial time series issues. TENCDE also effectively captures both spatial and temporal information, and avoids the curse-ofdimensionality issue. The unique solution and the consistency and convergence of solutions are also guaranteed. The experiments demonstrate the proposed TENCDE outperforms the existing models including the state-of-the-art methods.
更多查看译文
关键词
Tensorial time series,irregular time series,neural controlled differential equations
AI 理解论文
溯源树
样例
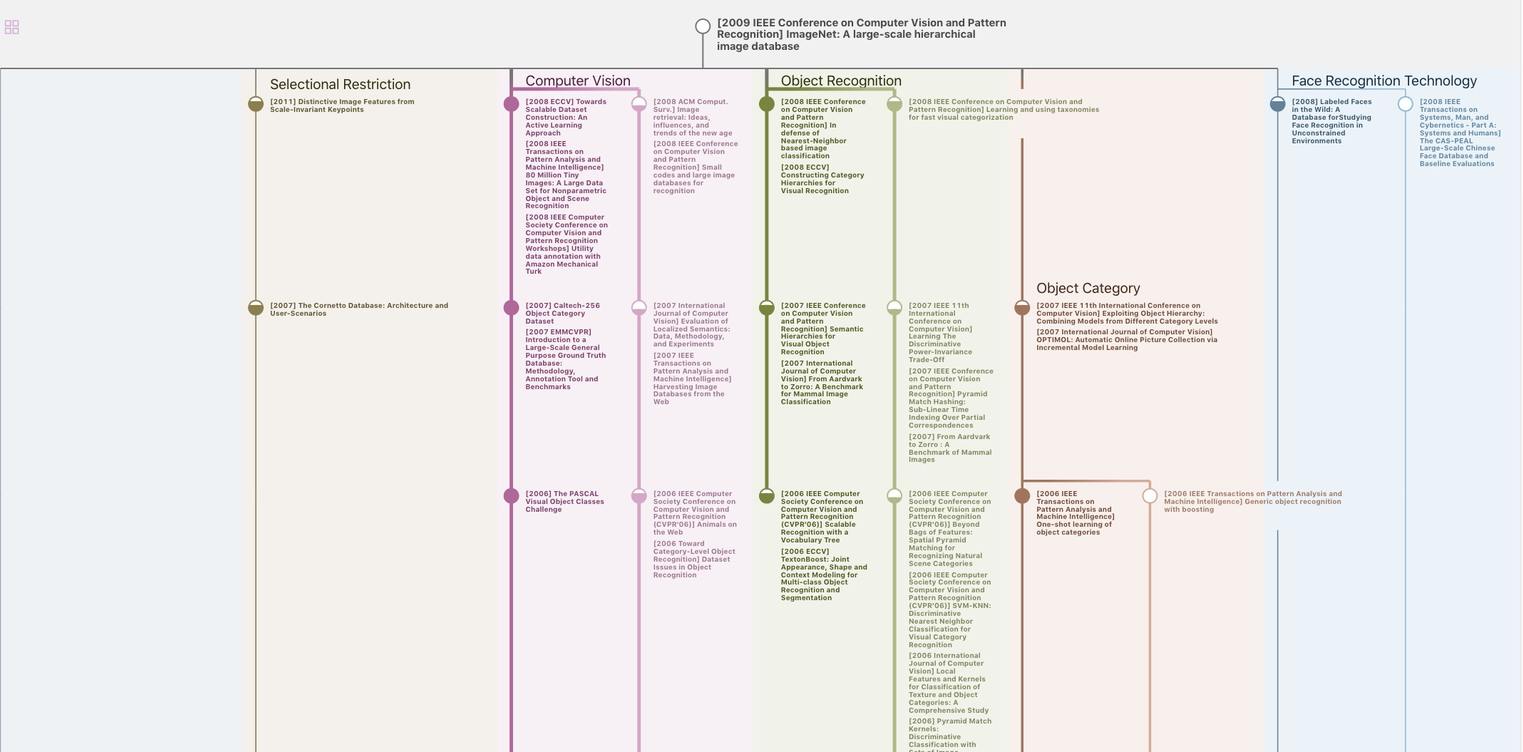
生成溯源树,研究论文发展脉络
Chat Paper
正在生成论文摘要