Learning Deep Contrastive Network for Facial Age Estimation
2022 International Joint Conference on Neural Networks (IJCNN)(2022)
摘要
Age estimation from a single facial image is an attractive and challenging research topic in the computer vision community. Most of previous works conventionally estimate absolute age from the input face images. However, telling someone's precise age at a glance without any reference information is essentially difficult even for humans. In this paper, we propose a novel Deep Contrastive Network (DCN) for age estimation, which can mine the variation information of samples. DCN model is trained end-to-end to learn the age distances between input unseen face and several reference images by contrasting deep feature maps. In the test phase, the input image is compared with a set of selected references to determine how many years younger or older than each of baselines which is more conform with human cognitive processes. We also propose a Cyclic Iterative Approximation Algorithm (CIAA) for post age voting which can further improve the accuracy. Therefore, the age estimation problem is cast as a metric learning task in our DCN model. By jointly learned with the cost sensitive loss and KL divergence loss, our DCN is easy to train and has very stable convergence. Extensive experiments show that the proposed approach significantly outperforms other state-of-the-art age estimation methods on MORPH II and FG-NET datasets.
更多查看译文
关键词
Age Estimation,Contrastive Network,Deep Learning,Metric Learning,Convolution Neural Network
AI 理解论文
溯源树
样例
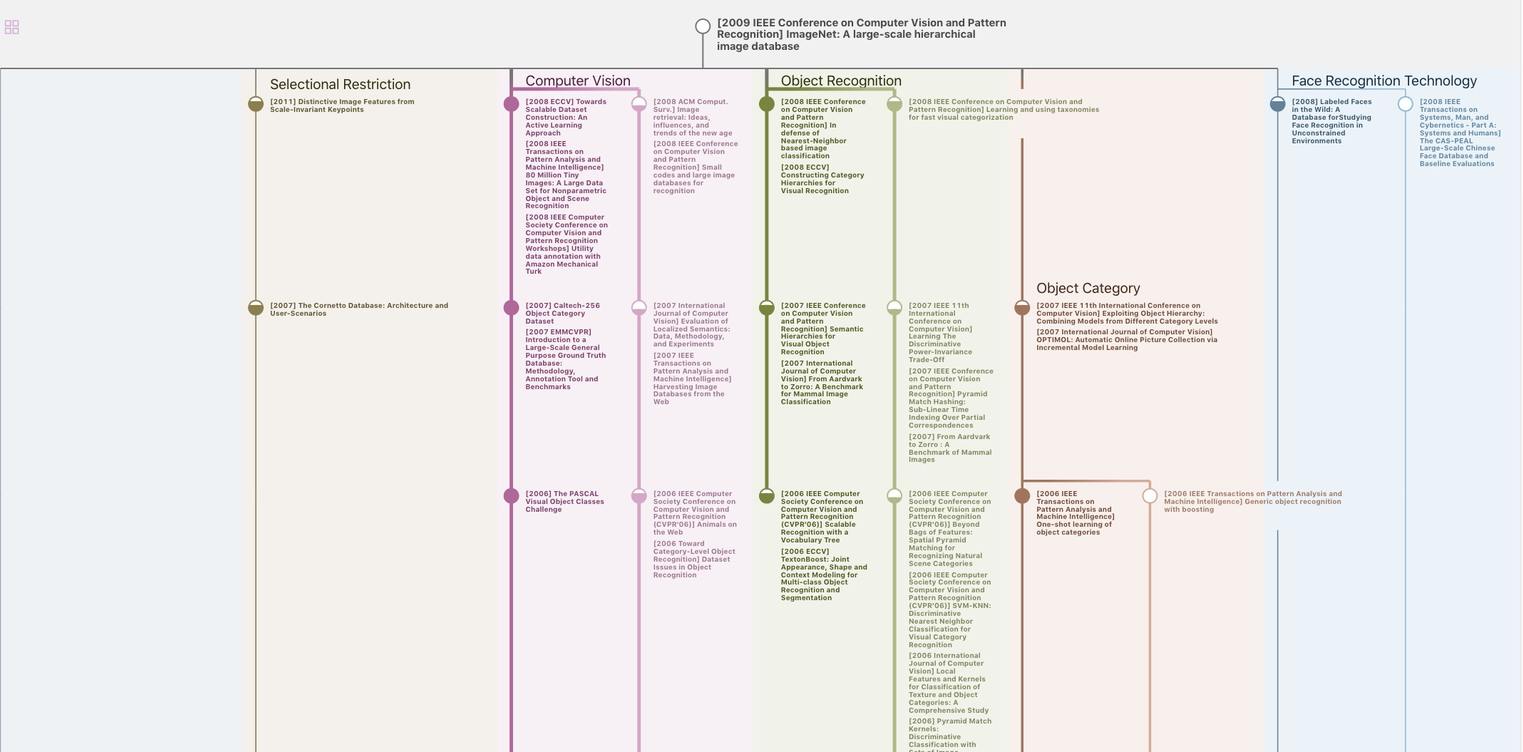
生成溯源树,研究论文发展脉络
Chat Paper
正在生成论文摘要