Efficient Time Series Classification using Spiking Reservoir
2022 International Joint Conference on Neural Networks (IJCNN)(2022)
摘要
In recent times, in many industrial domains, the need for in-situ processing of time series data from sensors have grown extensively, to ensure low-latency real-time responses, making embedded edge AI an important area of work. Most current techniques, being computationally intensive, are not suited for edge implementation; and in real world scenarios, connectivity to the cloud for processing sensed data leads to higher latency and often less reliability. Neuromorphic systems, coupled with spiking neural networks (SNN) offer a solution to such problems. This paper explores this evolving paradigm to address the need for low-footprint efficient time series classifiers implementable on edge, and targeted for predictive maintenance scenarios. A reservoir-based SNN architecture is designed and tried for classification of different vibration time series datasets. While the system is found to obtain at par classification accuracy for each of the datasets compared to prior arts, it is also observed to be more efficient in terms of synaptic operations per timestep (13% to 38%) using Gaussian temporal spike encoding scheme compared to Poisson rate encoding. Moreover, the system is found to be robust with respect to learning with reduction in training data (upto 20%).
更多查看译文
关键词
Spiking Neural Network,Reservoir computing,Time series classification,Neuromorphic computing
AI 理解论文
溯源树
样例
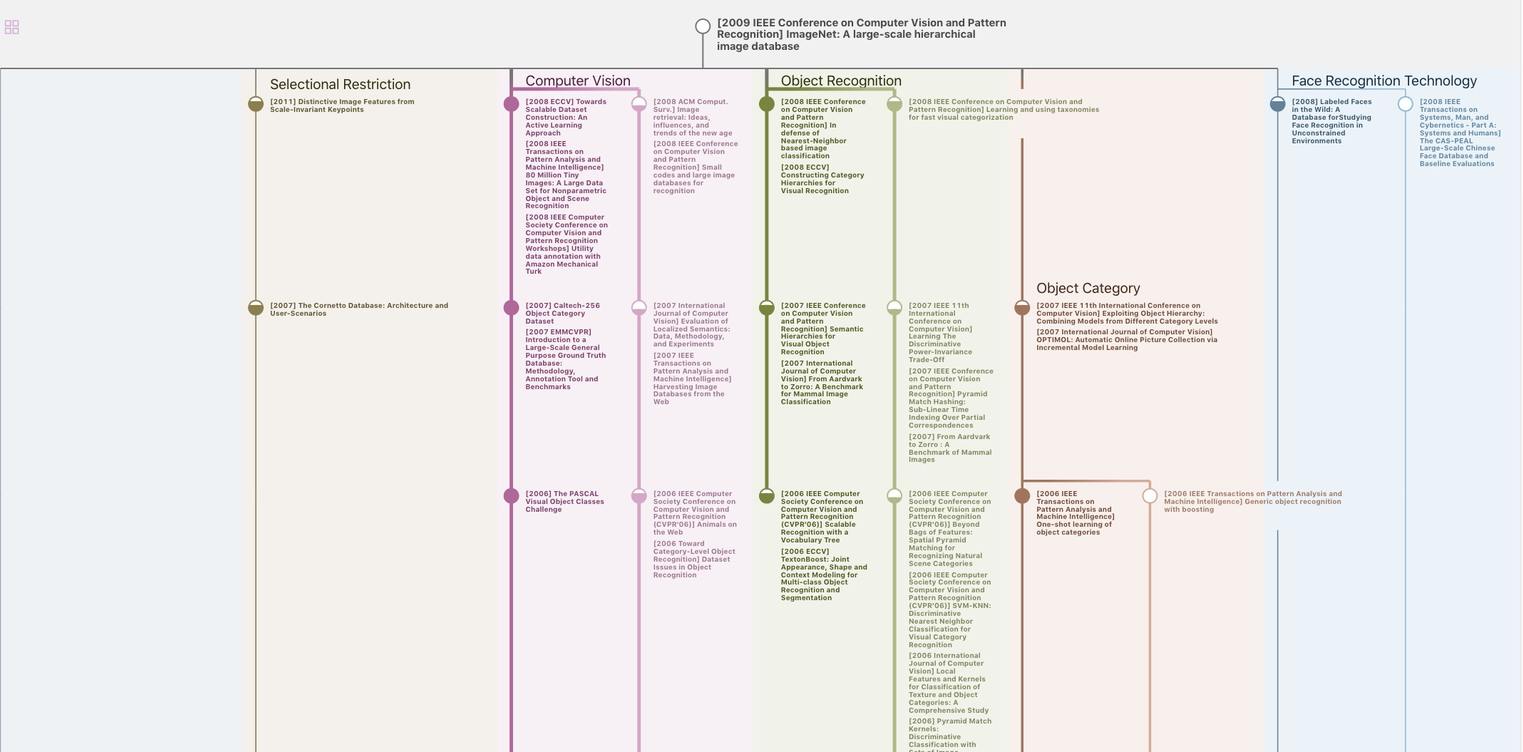
生成溯源树,研究论文发展脉络
Chat Paper
正在生成论文摘要