DGR: Decomposition Graph Reconstruction for Question Understanding
2022 International Joint Conference on Neural Networks (IJCNN)(2022)
摘要
To tackle the understanding of complex questions, Question Decomposition Meaning Representation (QDMR) decomposes a complex question into a sequence of atomic simple questions. However, state-of-the-art QDMR parsers neglect the type information of simple questions and the dependency information between simple questions, leading to limited overall performance. In this paper, we propose a Decomposition Graph Reconstruction (DGR) model to induce the information by introducing two additional tasks: simple question type classification and dependency relationship detection. DGR can generate sub-questions with the expected type and organize sub-questions with correct logical connections. Experimental results on the BREAK QDMR dataset demonstrate the effectiveness of our method compared with previous methods.
更多查看译文
关键词
Semantic parsing,Question decomposition,QDMR,Sequence-to-sequence,Neural network
AI 理解论文
溯源树
样例
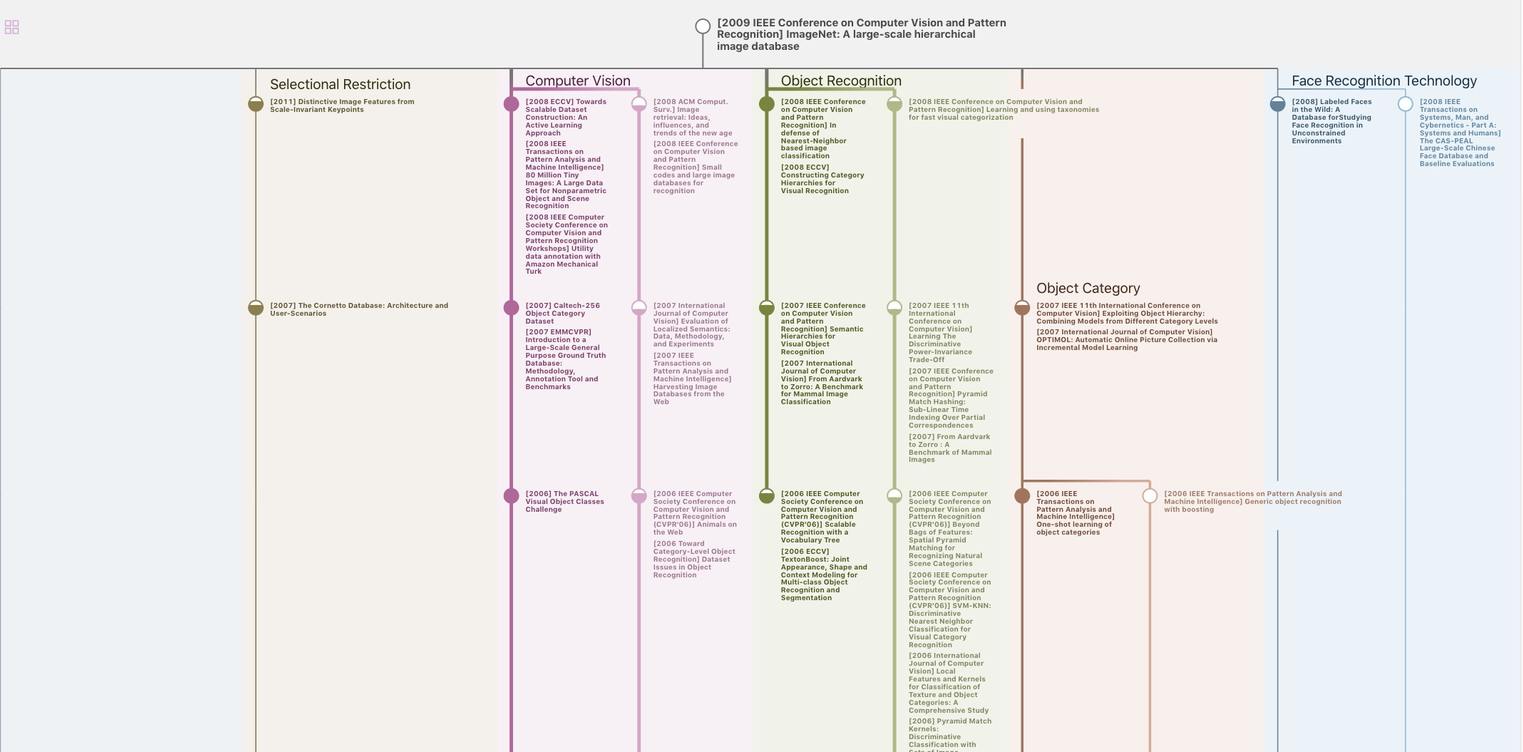
生成溯源树,研究论文发展脉络
Chat Paper
正在生成论文摘要