Exploring Coarse-grained Pre-guided Attention to Assist Fine-grained Attention Reinforcement Learning Agents
IEEE International Joint Conference on Neural Network (IJCNN)(2022)
摘要
Recently, people have applied the attention mechanism to deep reinforcement learning (DRL), which commits to helping agents focus on crucial factors to learn the task more effectively. However, there is still some margin between the current attention methods and natural human attention since evidence suggests that human attention can be pre-guided before they perform a task, allowing humans to quickly catch areas of important factors at the beginning of the task and then gradually refine fine-grained attention to learn the details during training. This allows humans to use their attention more efficiently. In this paper, we propose an attention method that mimics human attention for DRL in the Atari Games. The proposed method contains a fusion attention module, for which we build a simulated human coarse-grained pre-guided (SHCP) attention module to assist the original fine-grained attention of RL agents. The proposed SHCP attention module contains information about key objects for game tasks and is implemented as a coarse-grained attention region. The experimental results demonstrate that our method can quickly boost performance in the early stages and then outperform the current state-of-the-art fine-grained attention methods significantly in sample efficiency, just like human attention. Further analysis shows that, with fusion attention, agents can not only capture rich features of pre-guided attention but also extend to more improved features after training, which suggests the pre-guided attention signal acts as a good initializer. Therefore, we consider our work reveals a potential and promising direction that combines human attention signals to affect agents' behavior via attention mechanisms.
更多查看译文
关键词
deep reinforcement learning,attention mechanism
AI 理解论文
溯源树
样例
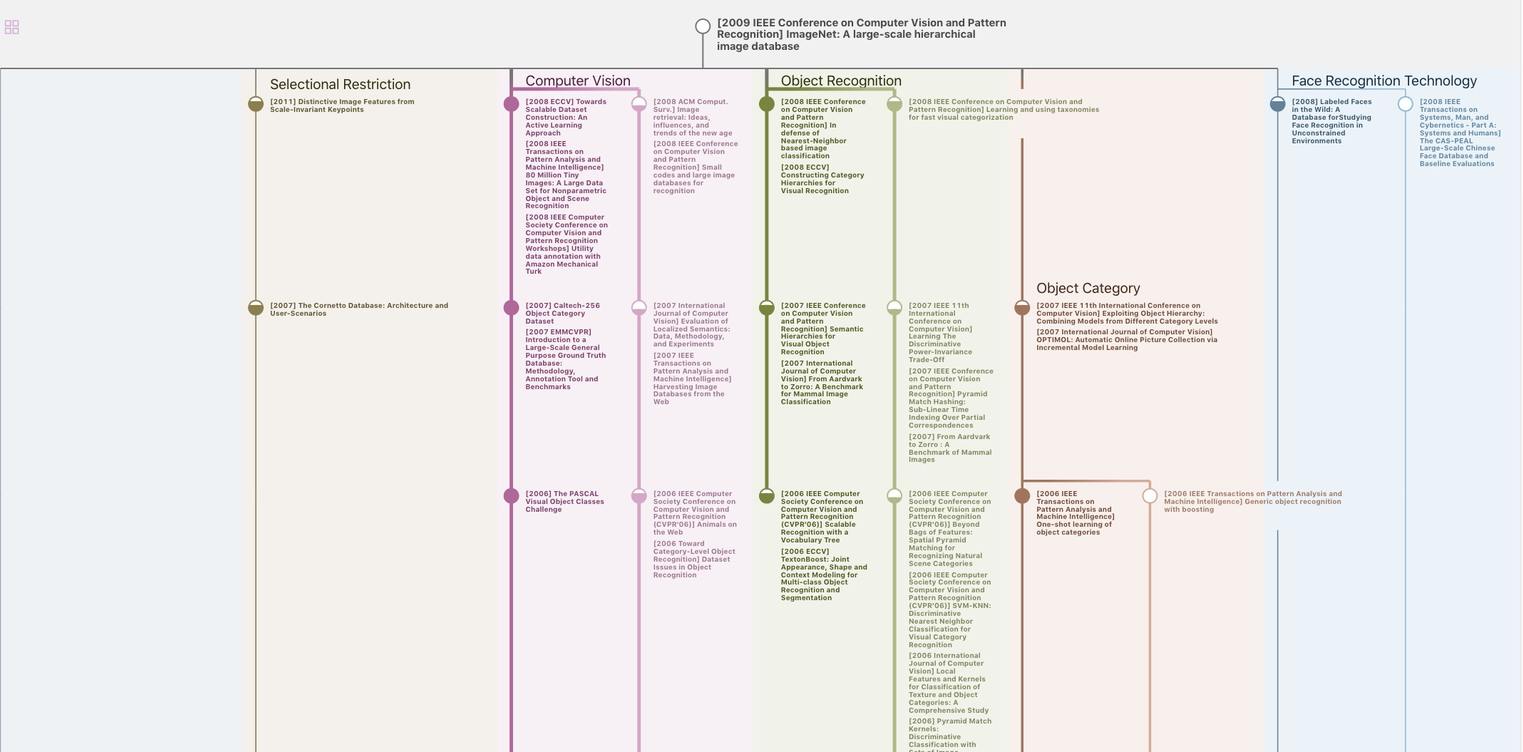
生成溯源树,研究论文发展脉络
Chat Paper
正在生成论文摘要