Explainable Retinopathy Diagnosis and Localisation by means of Class Activation Mapping
IEEE International Joint Conference on Neural Network (IJCNN)(2022)
摘要
Diabetic retinopathy is a disease afflicting the retina and currently is manually diagnosed by specialists through eye tomography inspection. In order to assist the clinician in this time-consuming task, in this paper, we propose a method aimed to automatically diagnose the (proliferative and non-proliferative) diabetic retinopathy by exploiting deep learning. Furthermore, we investigate the possibility to automatically localise the areas related to the disease by exploiting class activation maps. We evaluate different deep learning models from a quantitative point of view (i.e, using metrics like accuracy, precision and recall) and a qualitative point of view (by exploiting class activation maps and image similarity metrics) with the aim to understand the quality of predictions performed by a model in retinopathy diagnosis, reducing the amount of knowledge required to assess the model performance. From the experimental analysis is emerging that deep learning shows an interesting diagnostic potential in the retinopathy disease localisation and can effectively help the clinician in retinopathy diagnosis. Moreover, the adoption of the class activation maps and its comparison evaluation can help the developers to debug the training step of the model without medical expertise.
更多查看译文
关键词
retinopathy,deep learning,transfer learning,classification,diagnosis
AI 理解论文
溯源树
样例
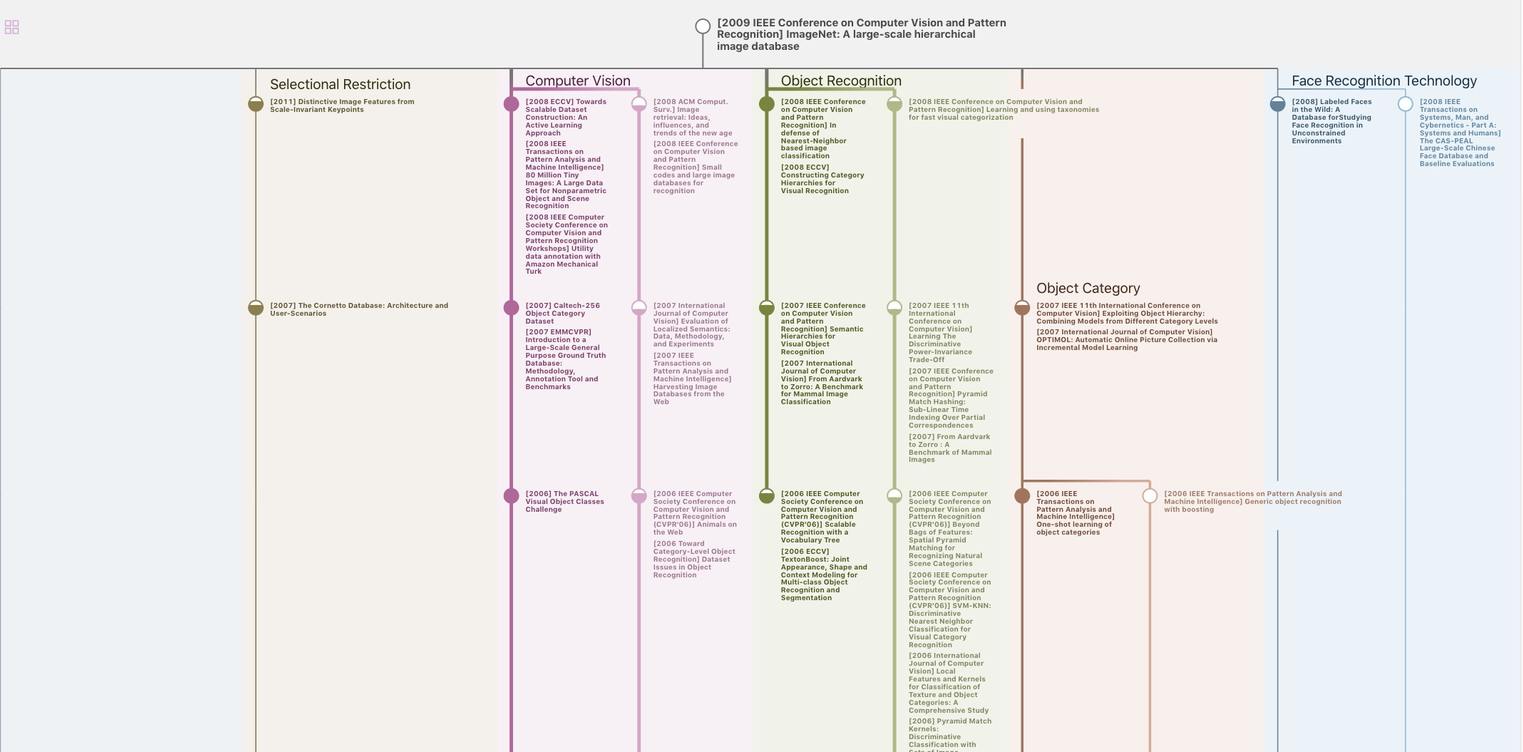
生成溯源树,研究论文发展脉络
Chat Paper
正在生成论文摘要