LRTD: A Low-rank Transformer with Dynamic Depth and Width for Speech Recognition
2022 International Joint Conference on Neural Networks (IJCNN)(2022)
摘要
Though Transformer-based models have achieved great success in the automatic speech recognition (ASR) field, they are generally resource-hungry and computation-intensive which makes them difficult to deploy in resource-restricted devices. In this paper, we propose LRTD, a lightweight Transformer for end-to-end ASR. LRTD compresses the model size using matrix decomposition during training and further reduces the depth and width of the trained model dynamically leveraging two structured pruning strategies. The performance of the pruned model reply on two additional structured dropout methods and two search techniques that can recognize the important layers and attention heads. The experimental results show that our proposed model can achieve competitive performance on Aishell-1 even with about 2.27 × fewer model parameters compared to the baseline transformer model. Moreover, we experimentally demonstrate that the matrix decomposition technique achieves a higher compression rate while two pruning methods can adjust the model size flexibly.
更多查看译文
关键词
transformer,speech recognition,prune,matrix decomposition,model compression
AI 理解论文
溯源树
样例
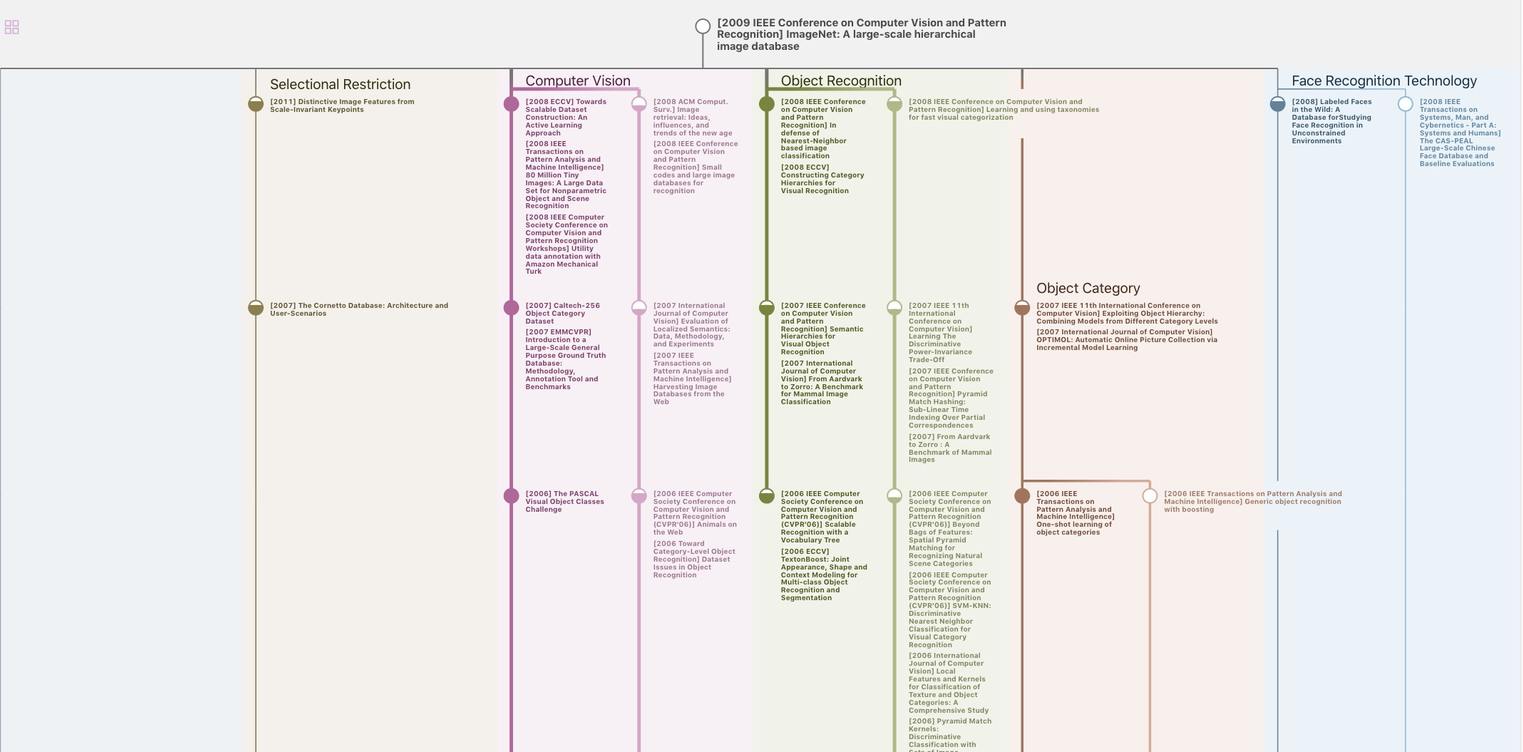
生成溯源树,研究论文发展脉络
Chat Paper
正在生成论文摘要