Few-shot chest x-ray diagnosis using discriminative ensemble learning
Meta-Learning with Medical Imaging and Health Informatics Applications(2023)
摘要
Few-shot learning, in spite of its recent popularity, remains almost unexplored in medical image analysis. We design a few-shot classifier for the diagnosis of chest x-rays using discriminative ensemble learning. Our method consists of a CNN-based coarse-learner for feature extraction from chest x-rays followed by a saliency-based classifier to classify chest x-rays through the extraction and utilization of disease-specific salient features. We propose a novel discriminative autoencoder ensemble to design the saliency-based classifier. Our algorithm proceeds through metatraining and metatesting phases. During the training phase of metatraining, we train the coarse-learner. However, during the training phase of metatesting, we train only the saliency-based classifier. Thus, our method is first-of-its-kind where the training phase of metatraining and the training phase of metatesting are architecturally and temporally disjoint. Consequently, the proposed method is architecturally modular and may be easily adaptable to new tasks requiring the training of only the saliency-based classifier. Experiments show as high as ∼19% improvement in terms of F1 score compared to the baseline in the diagnosis of chest x-rays from publicly available datasets.
更多查看译文
关键词
chest,learning,diagnosis,few-shot,x-ray
AI 理解论文
溯源树
样例
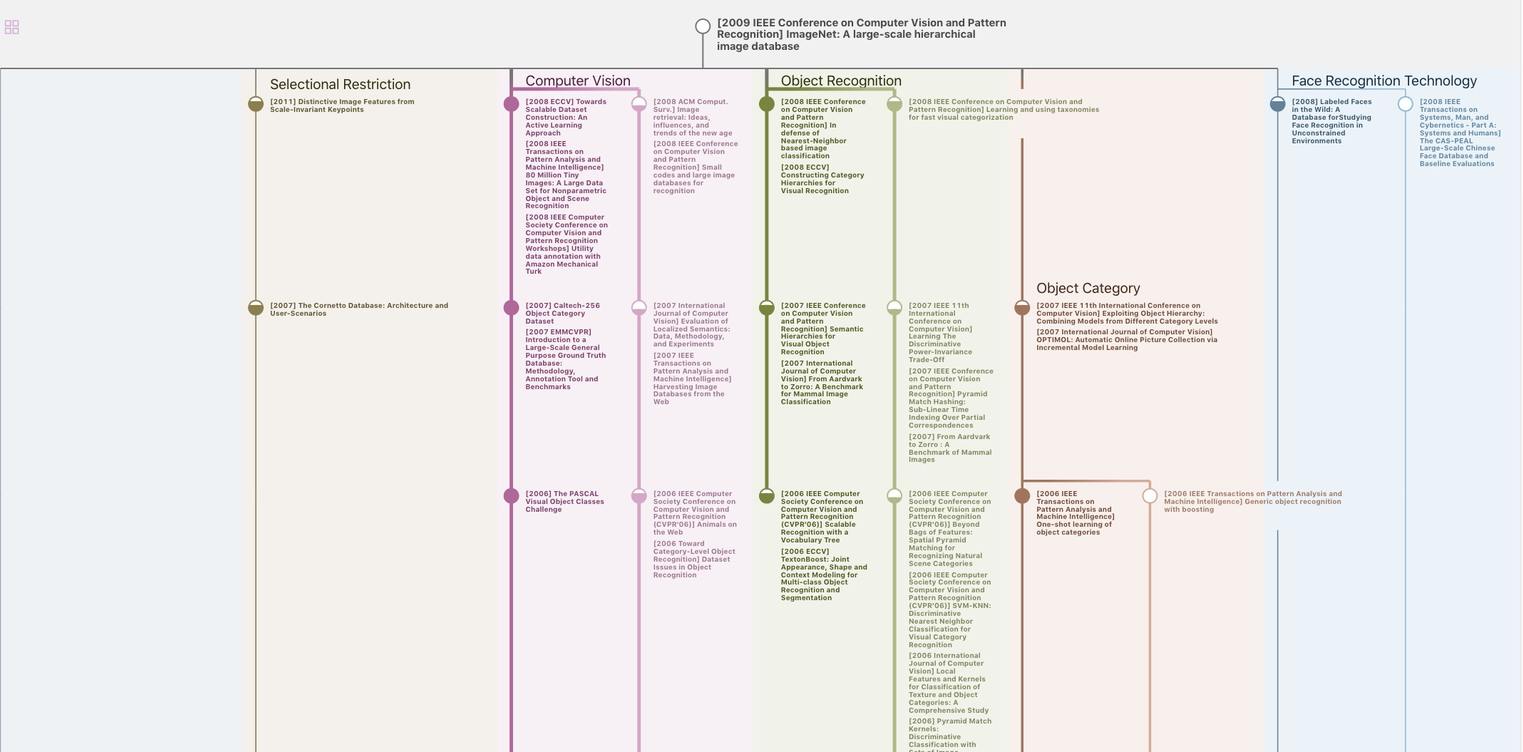
生成溯源树,研究论文发展脉络
Chat Paper
正在生成论文摘要