A multi-view extractive text summarization approach for long scientific articles
IEEE International Joint Conference on Neural Network (IJCNN)(2022)
摘要
Automatic text summarization aims to generate a simple and descriptive summary covering the main content of a document. Most of the literature in automatic text summarization focuses on proposing and improving Deep Learning methods in order to make these models applicable in the context of long text summarization. Unfortunately, these models still have limitations on the input sequence length. Such a limitation may lead to a loss of information that impairs the quality of the summaries generated. For this reason, we propose a Multi-View Extractive Text Summarization approach for long scientific texts. Our hypothesis is that focusing on getting a better representation of the text is a key attribute for this task. We treat extractive summarization as a binary classification problem. We propose and evaluate a view fusion method that are independent of the classification model. We show that using this strategy we generate more consistent models that have a small score variance. Our experiments with real world articles show that our approach outperforms recent state-of-the-art models in extractive. abstractive and hybrid summarization.
更多查看译文
关键词
articles,text,multi-view
AI 理解论文
溯源树
样例
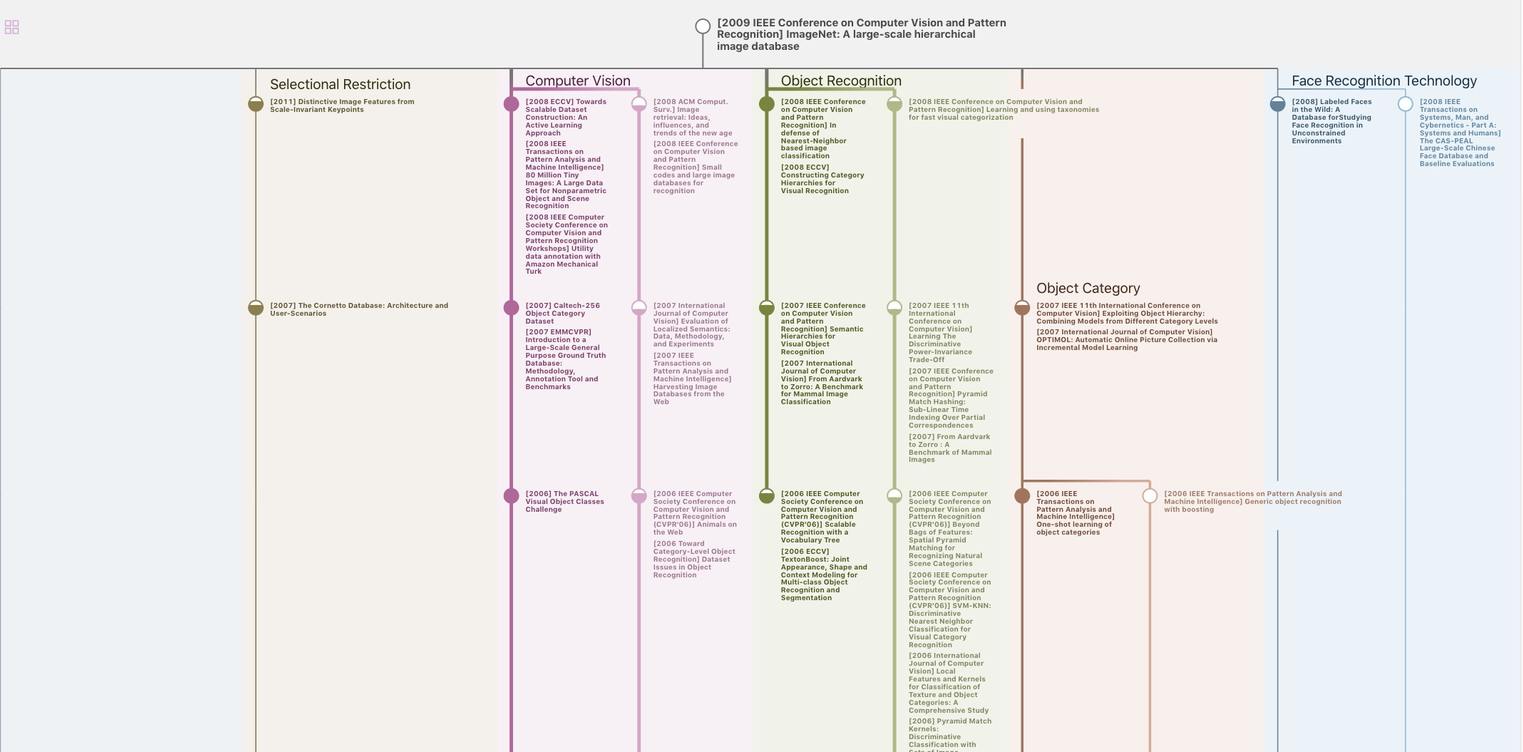
生成溯源树,研究论文发展脉络
Chat Paper
正在生成论文摘要