Evaluating Complex Sparse Representation of Hypervectors for Unsupervised Machine Learning
IEEE International Joint Conference on Neural Network (IJCNN)(2022)
摘要
The increasing use of Vector Symbolic Architectures (VSA) in machine learning has contributed towards en-ergy efficient computation, short training cycles and improved performance. A further advancement of VSA is to leverage sparse representations, where the VSA-encoded hypervectors are sparsified to represent receptive field properties when encoding sensory inputs. The hyperseed algorithm is an unsupervised machine learning algorithm based on VSA for fast learning a topology preserving feature map of unlabelled data. In this paper, we implement two methods of sparse block-codes on the hyperseed algorithm, they are selecting the maximum element of each block and selecting a random element of each block as the nonzero element. Finally, the sparsified hyperseed algorithm is empirically evaluated for performance using three distinct bench-mark datasets, Iris classification, classification and visualisation of synthetic datasets from the Fundamental Clustering Problems Suite and language classification using n-gram statistics.
更多查看译文
关键词
Sparse Representations,Vector Symbolic Architecture,Unsupervised Learning,Block Codes,Hyperseed
AI 理解论文
溯源树
样例
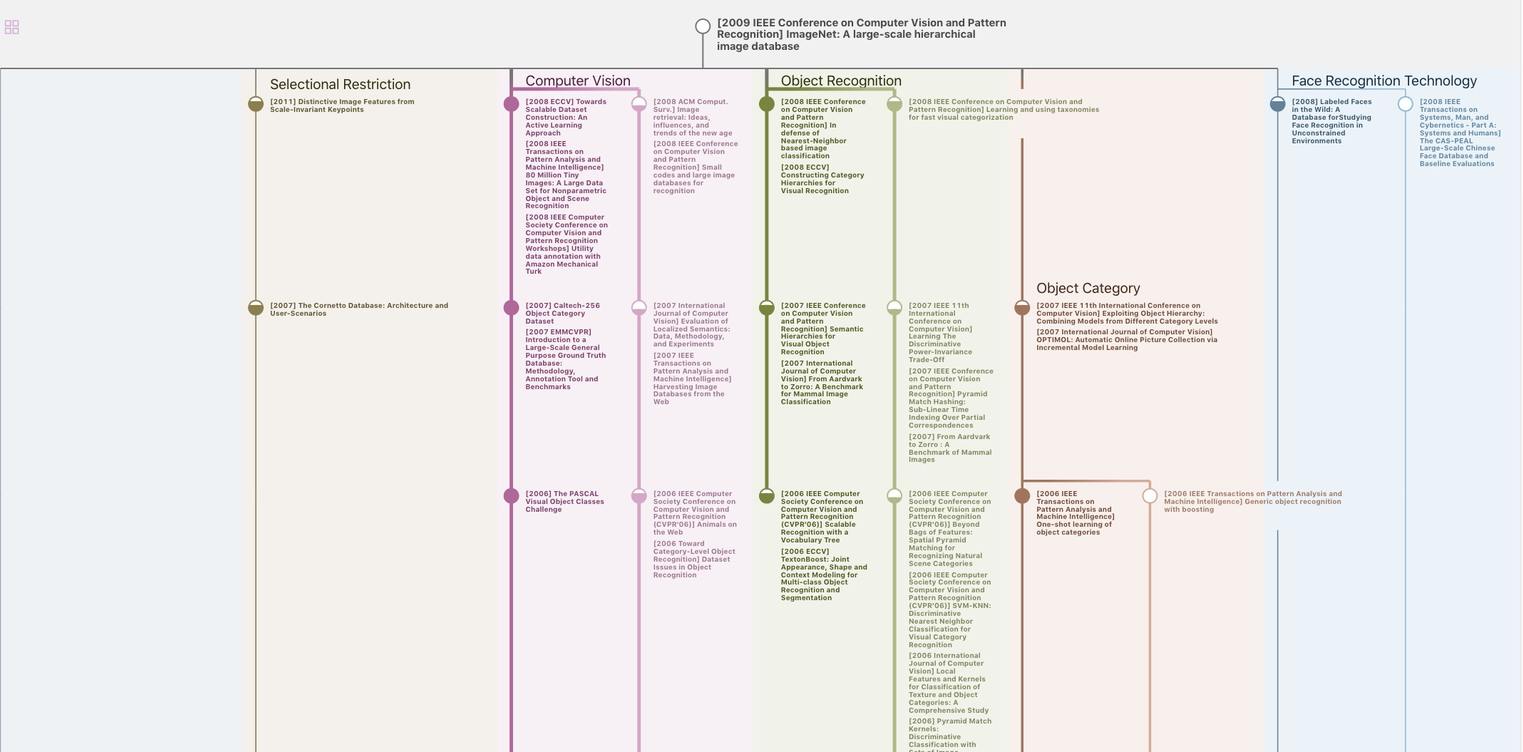
生成溯源树,研究论文发展脉络
Chat Paper
正在生成论文摘要