Crafting Textual Adversarial Examples through Second-Order Enhanced Word Saliency
IEEE International Joint Conference on Neural Network (IJCNN)(2022)
摘要
Textual adversarial examples crafted with well-designed perturbation can mislead state-of-the-art natural language models. Most previous works on word-level black-box attacks propose different text substituting strategies based on the word saliency determined by Leave One Out (LOO) methods, while the attack effectiveness is actually limited due to the model pathology. The word saliency determined by LOO methods can be severely affected by the model pathology, and unconscious bias is introduced. In this paper, we propose a word saliency method called Second-Order Enhanced Word Saliency (SOEWS), which considers the overfitting information obtained from the second-order model pathological behavior that helps to reduce the bias of LOO methods. Extensive experiments show that our method outperforms current black-box word saliency methods and can boost the effectiveness of previous attack frameworks. Performing adversarial training utilizing our method best improves model robustness, and human evaluation shows that the adversarial examples crafted by our method are almost imperceptible to humans. We also conduct case study to intuitively demonstrate the superiority of our method.
更多查看译文
关键词
adversarial attack,word saliency,natural language processing
AI 理解论文
溯源树
样例
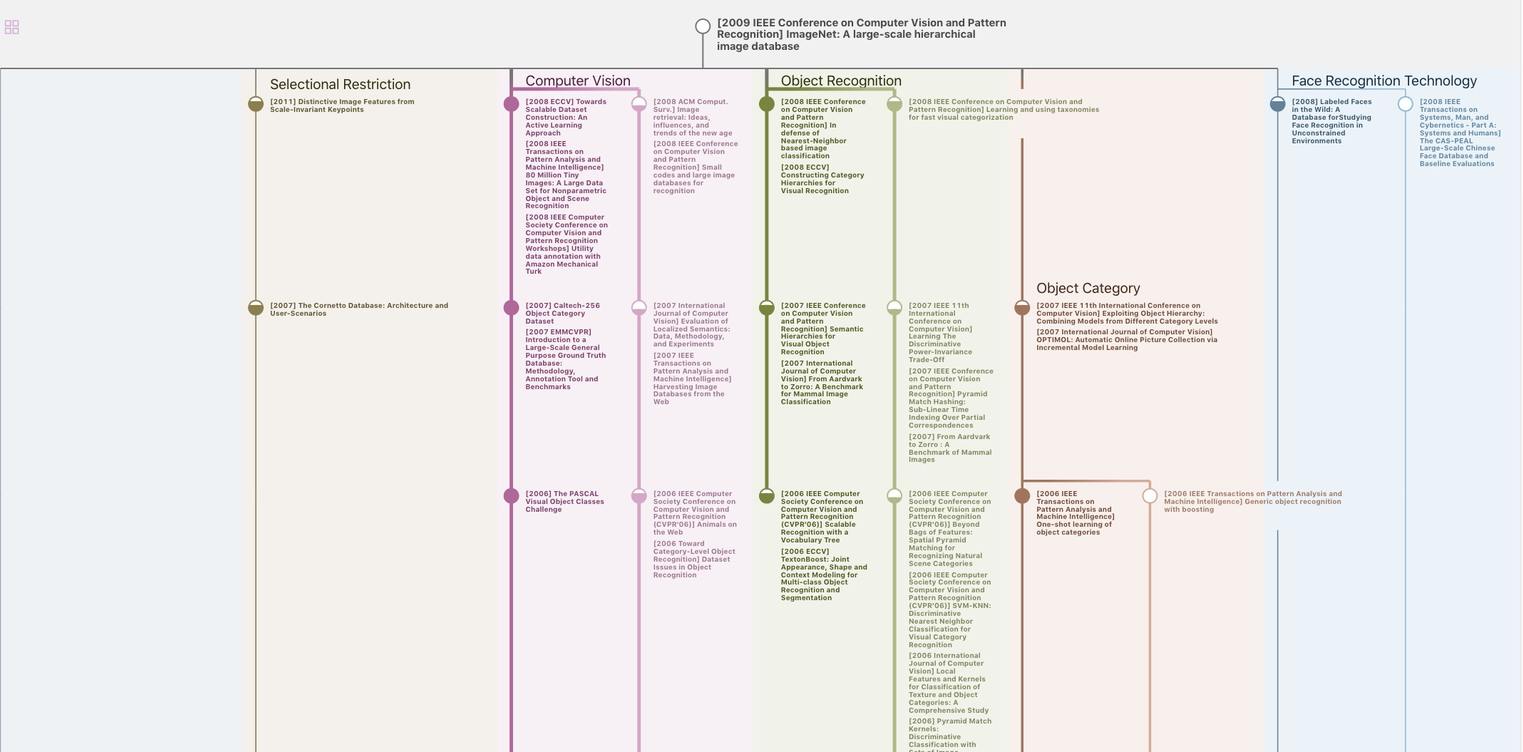
生成溯源树,研究论文发展脉络
Chat Paper
正在生成论文摘要