Real-Time Class-Incremental Learning for Voice Command Recognition via Adaptive oiSGNG
IEEE International Joint Conference on Neural Network (IJCNN)(2022)
摘要
Online learning approach allows an Artificial Neural Network (ANN) to solve dynamic real-world problems. In this context, the objective of this work is to implement ANN-based voice recognition models with focus on class-incremental learning in real time, for low-cost implementations, such as an electric wheelchair. In this paper, the online incremental Supervised Growing Neural Gas (oiSGNG) with a feature extractor is proposed as a voice command classifier. About this model, two contributions are presented: (i) nodes are inserted according to an exponential function, that results in a higher accuracy rate with fewer nodes, which implies less latency; (ii) adaptive oiSGNG, this model is a novel implementation that enables online learning. In offline experiments, the model proposed performs better than the Self-Organizing Map (SOM) in its topological and supervised version. After simulations and experiments, it is proposed to use a keyword to avoid false positives. In the results, the accuracy of the proposed model is better than the original oiSGNG.
更多查看译文
关键词
Online Learning,Class-Incremental Learning,Supervised Growing Neural Gas,Real-Time Audio Signal Processing,Electric Wheelchair
AI 理解论文
溯源树
样例
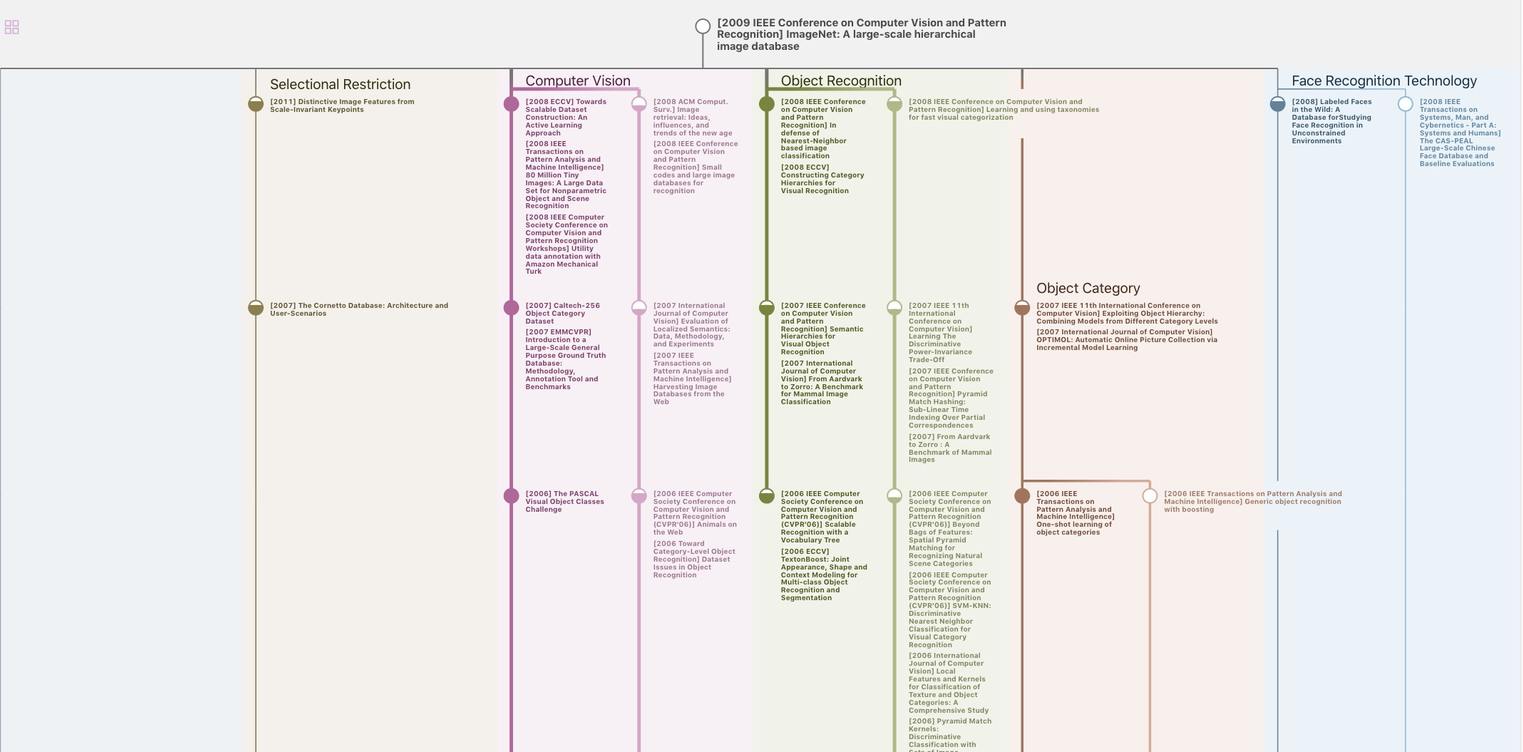
生成溯源树,研究论文发展脉络
Chat Paper
正在生成论文摘要