RAP-Net: A Resource Access Pattern Network for Insider Threat Detection.
IEEE International Joint Conference on Neural Network (IJCNN)(2022)
摘要
The subtle and dynamic nature of insider threat makes it one of the most challenging problems in cyber security domain. Most of the existing studies model the problem from the perspective of user behavior, but the imbalance of data categories and the weak correlation between discrete behaviors are not considered simultaneously. To address these problems, we use reinforcement learning-based Generative Adversarial Network to synthesize high-quality minority class data, and use Word2Vec language model to learn the distance metric between different behaviors. In this paper, we propose a Resource Access Pattern Network (RAP-Net), which applies reinforcement learningbased Generative Adversarial Network, Word2Vec, Convolutional Neural Network, Recurrent Neural Network, and Attention Mechanism to insider threat detection. RAP-Net extracts user resource access pattern sequences from audit log files, and then performs data augmentation on the minority class sequences. After learning the distance metric of different tokens in sequences, feature vectors are sent to the classifier for anomaly detection. RAP-Net successfully addresses two major pain points in the current field, namely data imbalance and weak correlation of discrete behaviors. Intensive experimental results on the CMU-CERT r4.2 dataset demonstrate that RAP-Net outperforms state-of-the-art studies in the field.
更多查看译文
关键词
insider threat detection,generative adversarial network,word2vec,recurrent neural network
AI 理解论文
溯源树
样例
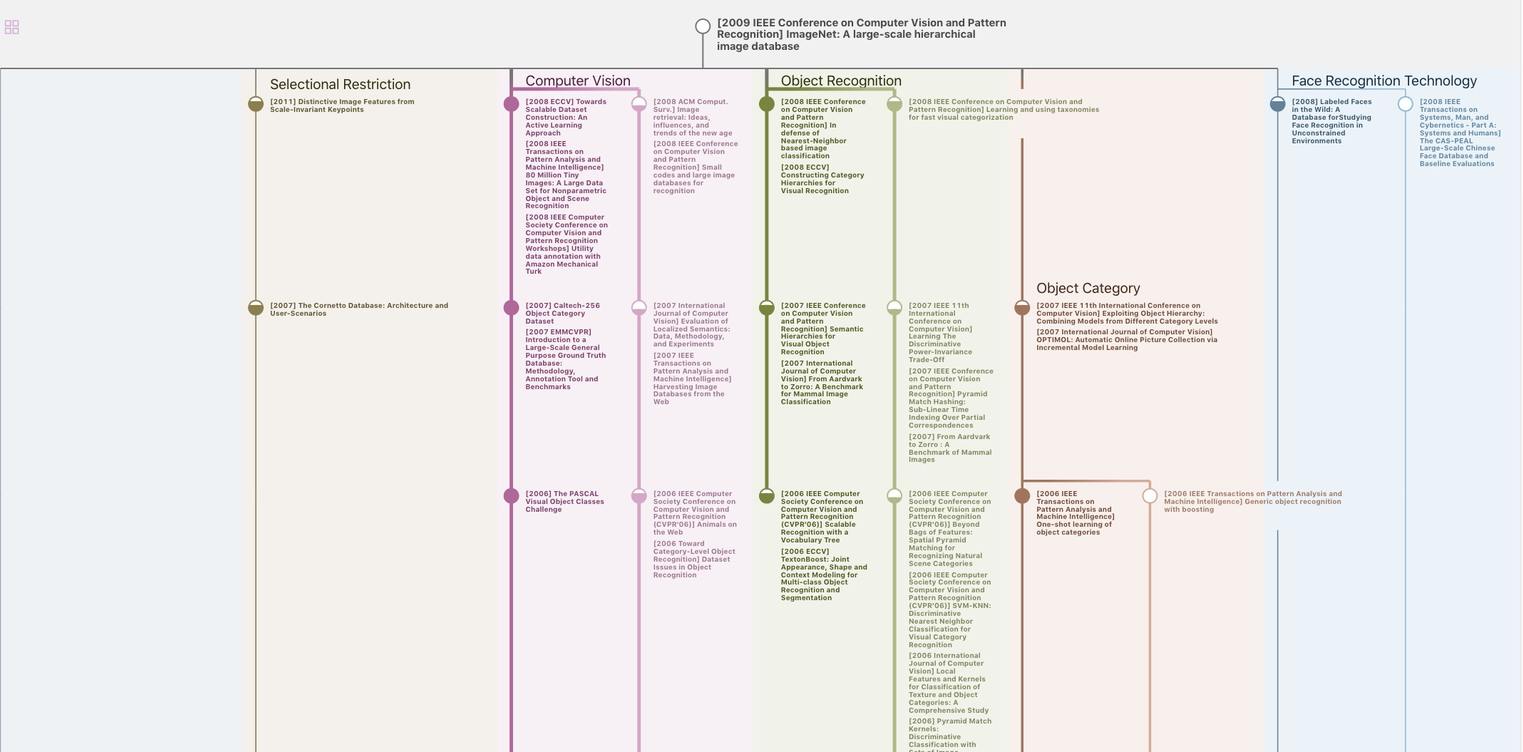
生成溯源树,研究论文发展脉络
Chat Paper
正在生成论文摘要