Improved Design of Bayesian Networks for Modelling Toxicity Risk in Breast Radiotherapy using Dynamic Discretization
IEEE International Joint Conference on Neural Network (IJCNN)(2022)
摘要
This study investigates the dynamic discretization approach with the purpose of improving probabilistic causal models for the task of toxicity risk assessment in breast radiotherapy. We considered a probabilistic causal model such as Bayesian Networks, and implemented a modified version of Fenton & Neil's dynamic discretization algorithm to validate and analyze these models in terms of performance. Our implementation performs discretization at the data- or distribution-level. This approach is shown to provide significant improvement over static methods when assessing distribution fit via relative entropy error, as well as being very computationally efficient. Predictive performance was compared across four distinct datasets using Bayesian Networks, and dynamic discretization was not found to consistently outperform static techniques despite generating discretizations with significantly better fit to their underlying distributions.
更多查看译文
关键词
Bayesian network,discretization,information theory,machine reasoning,probability distribution
AI 理解论文
溯源树
样例
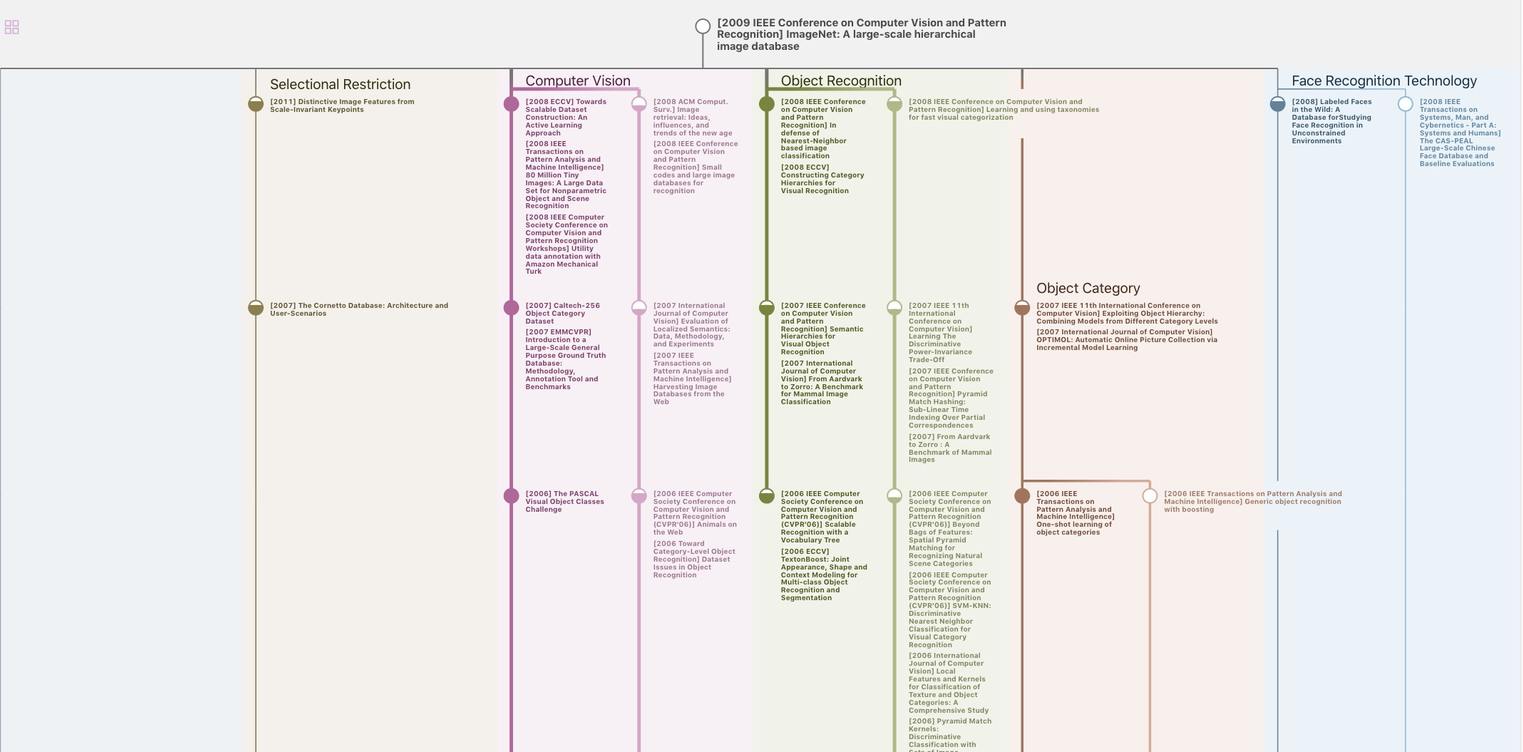
生成溯源树,研究论文发展脉络
Chat Paper
正在生成论文摘要