ACE: A Coarse-to-Fine Learning Framework for Reliable Representation Learning Against Label Noise.
IEEE International Joint Conference on Neural Network (IJCNN)(2022)
摘要
The prosperity of deep neural networks in various computer vision applications heavily depends on large-scale high-quality annotated datasets. However, inaccurate annotations are often inevitable when building hand-labeled datasets, which can lead to imperfectly supervision problems. Most existing methods to address these problems mainly use noisy labels to learn latent representations, which may be corrupted when the noise rate is high, thus limiting the predictions for clean test data set. In this paper, we propose a flexible coarse-to-fine representation learning framework to improve the quality of latent representations by exploiting the reliable geometry information from raw features. This framework includes: (i) an anchored representation learner, which is a coarse mechanism for learning reasonable feature-similarity to avoid feature corruption, (ii) a confident representation learner, which is used to learn confident data pairs, and (iii) an exploratory representation learner, which is proposed for learning from feasible unconfident pairs and performing label refurbishment. Comprehensive experiments have demonstrated the effectiveness of our proposed method, especially when the noise level is high and the task is relatively difficult.
更多查看译文
关键词
Noisy label,Weakly supervised learning,Feature representation learning
AI 理解论文
溯源树
样例
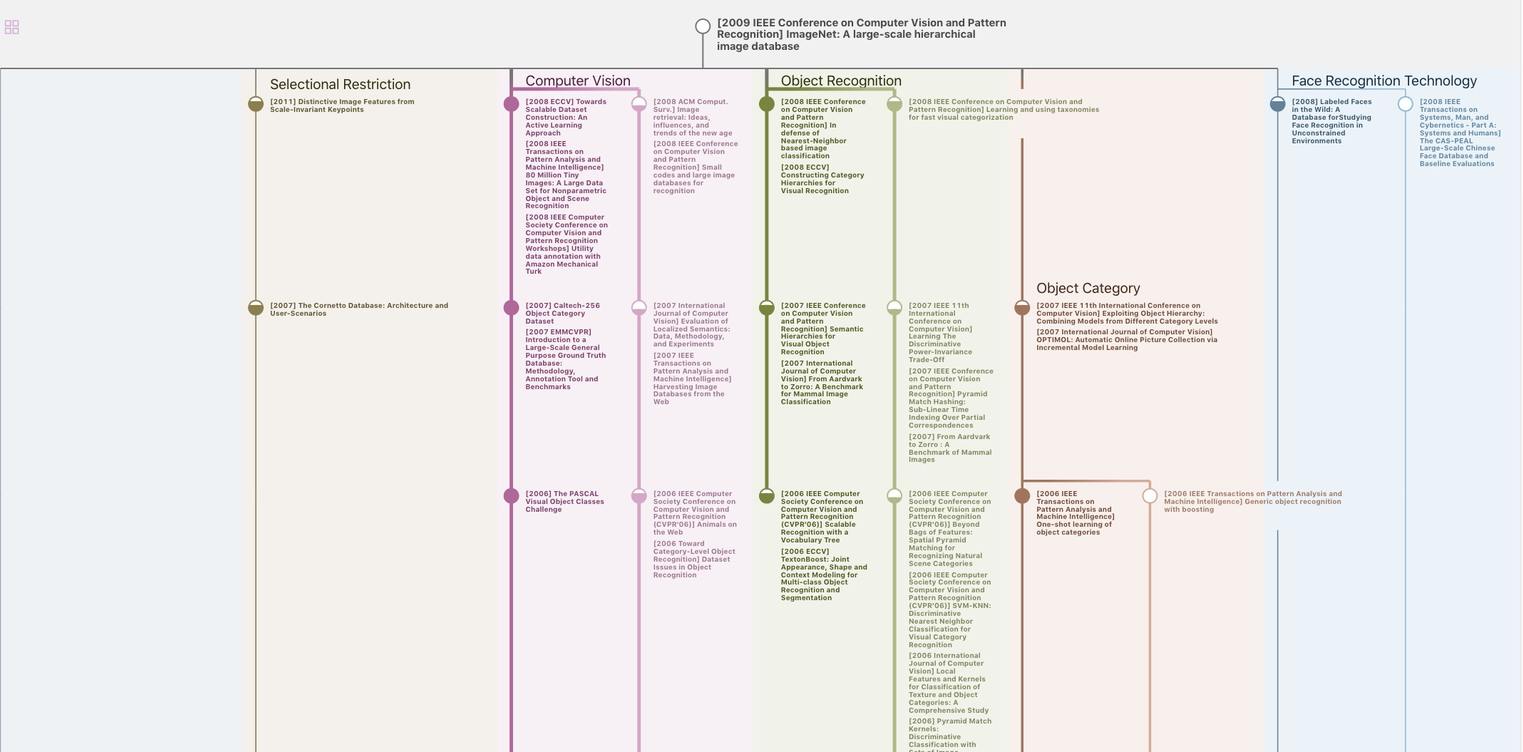
生成溯源树,研究论文发展脉络
Chat Paper
正在生成论文摘要